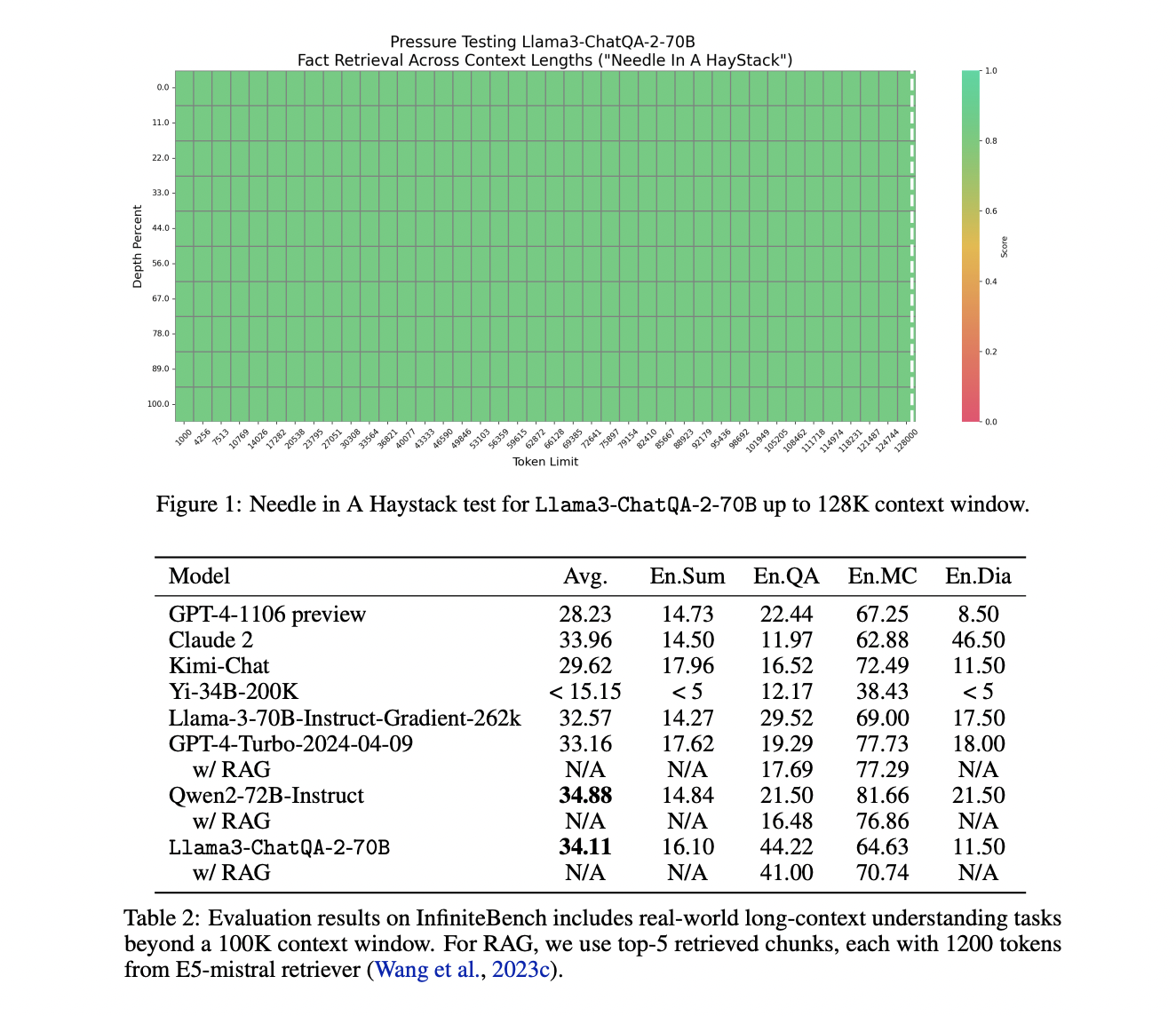
Practical Solutions and Value of ChatQA 2: A Llama3-based Model
Enhanced Long-Context Understanding and RAG Capabilities
Long-context understanding and retrieval-augmented generation (RAG) in large language models (LLMs) are crucial for tasks such as document summarization, conversational question answering, and information retrieval.
ChatQA 2 extends the context window to 128K tokens and utilizes a three-stage instruction tuning process to significantly enhance instruction-following, RAG performance, and long-context understanding. This model achieves accuracy comparable to GPT-4-Turbo and excels in RAG benchmarks, demonstrating superior results in functions requiring extensive text processing.
The model addresses significant issues in the RAG pipeline, such as context fragmentation and low recall rates, improving retrieval accuracy and efficiency. It offers flexible solutions for various downstream tasks, balancing accuracy and efficiency through advanced long-context and retrieval-augmented generation techniques.
For AI KPI management advice, connect with us at hello@itinai.com. For continuous insights into leveraging AI, stay tuned on our Telegram or Twitter.
Discover how AI can redefine your sales processes and customer engagement. Explore solutions at itinai.com.