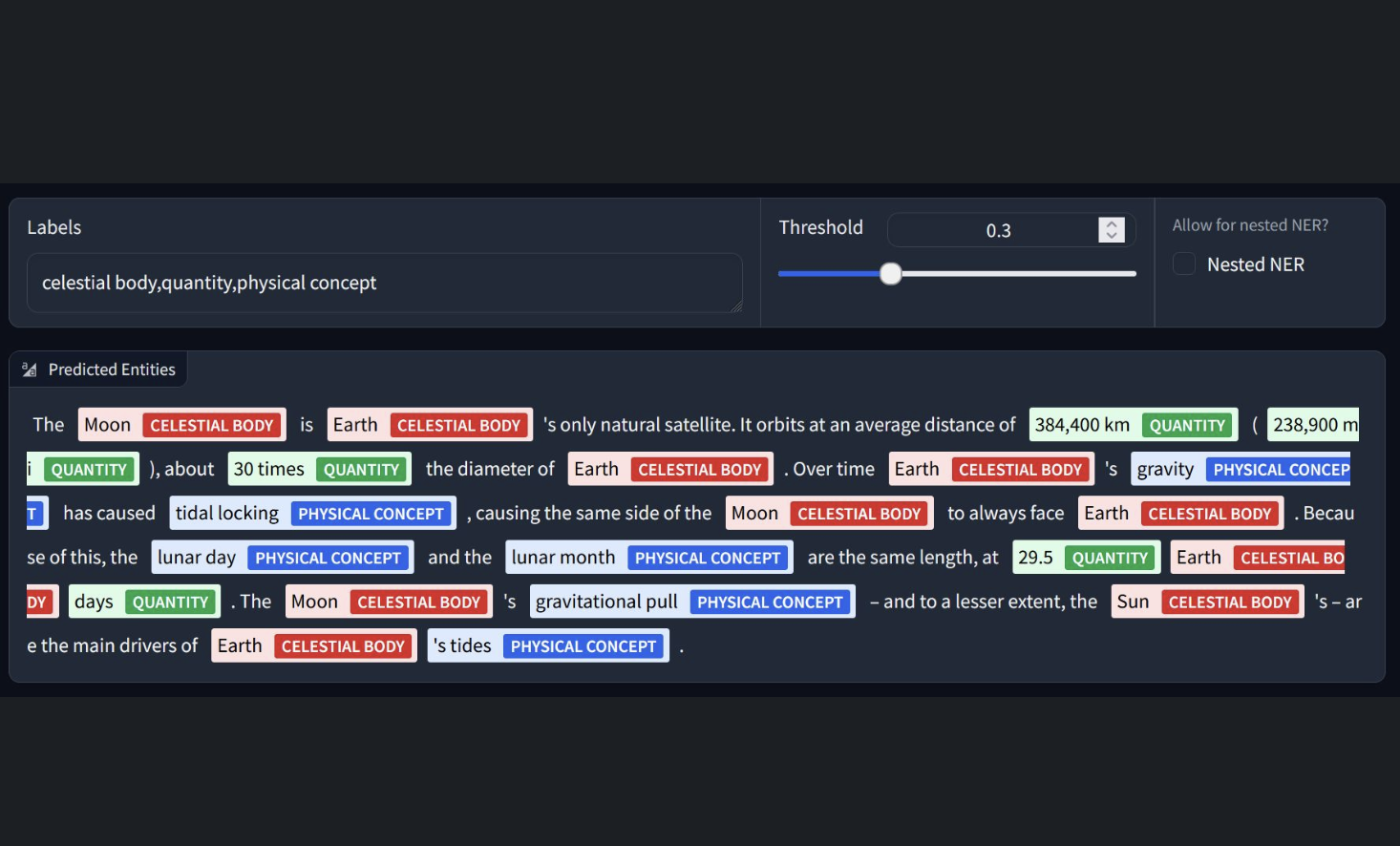
Practical AI Solutions for Named Entity Recognition (NER)
Introduction
Named Entity Recognition (NER) is vital in natural language processing, with applications in various fields such as medical coding, financial analysis, and legal document parsing. Custom models are typically created using transformer encoders pre-trained on self-supervised tasks like masked language modeling (MLM).
NuMind Approach
The NuMind team introduces an innovative approach that utilizes large language models (LLMs) to reduce human annotations for custom model creation. They propose using LLMs to annotate a diverse, multi-domain dataset covering various NER problems. Subsequently, a smaller foundation model like BERT is further pre-trained on this annotated dataset, which can then be fine-tuned for any downstream NER task.
NuMind’s NER Models
NuMind has introduced three NER models with distinct features and applications:
- NuNER Zero: A compact zero-shot NER model suitable for moderate token size.
- NuNER Zero 4K: A variation of NuNER that performs better in scenarios where context size matters.
- NuNER Zero-span: The span-prediction version of NuNER Zero, showing slightly better performance for entities under 12 tokens.
Value Proposition
In conclusion, NER is crucial in natural language processing, and the NuMind team’s approach and NER models offer practical solutions to streamline custom model creation and address the challenges posed by large language models.
AI Solutions for Your Business
Stay competitive and redefine your way of work with AI. Identify automation opportunities, define KPIs, select AI solutions that align with your needs, and implement gradually. For AI KPI management advice and continuous insights into leveraging AI, connect with us at hello@itinai.com.
Practical AI Solution Spotlight
Explore the AI Sales Bot from itinai.com/aisalesbot, designed to automate customer engagement 24/7 and manage interactions across all customer journey stages.