MIT researchers have developed a machine-learning technique called Diffusion-CCSP that enables robots to efficiently solve complex packing problems. The technique uses a collection of machine-learning models, each representing a specific type of constraint, which are combined to generate global solutions. The method outperformed other techniques, generating a greater number of effective solutions. The researchers aim to test Diffusion-CCSP in more complicated scenarios and enable it to tackle problems in different domains without retraining. Funding for the research was provided by various organizations.
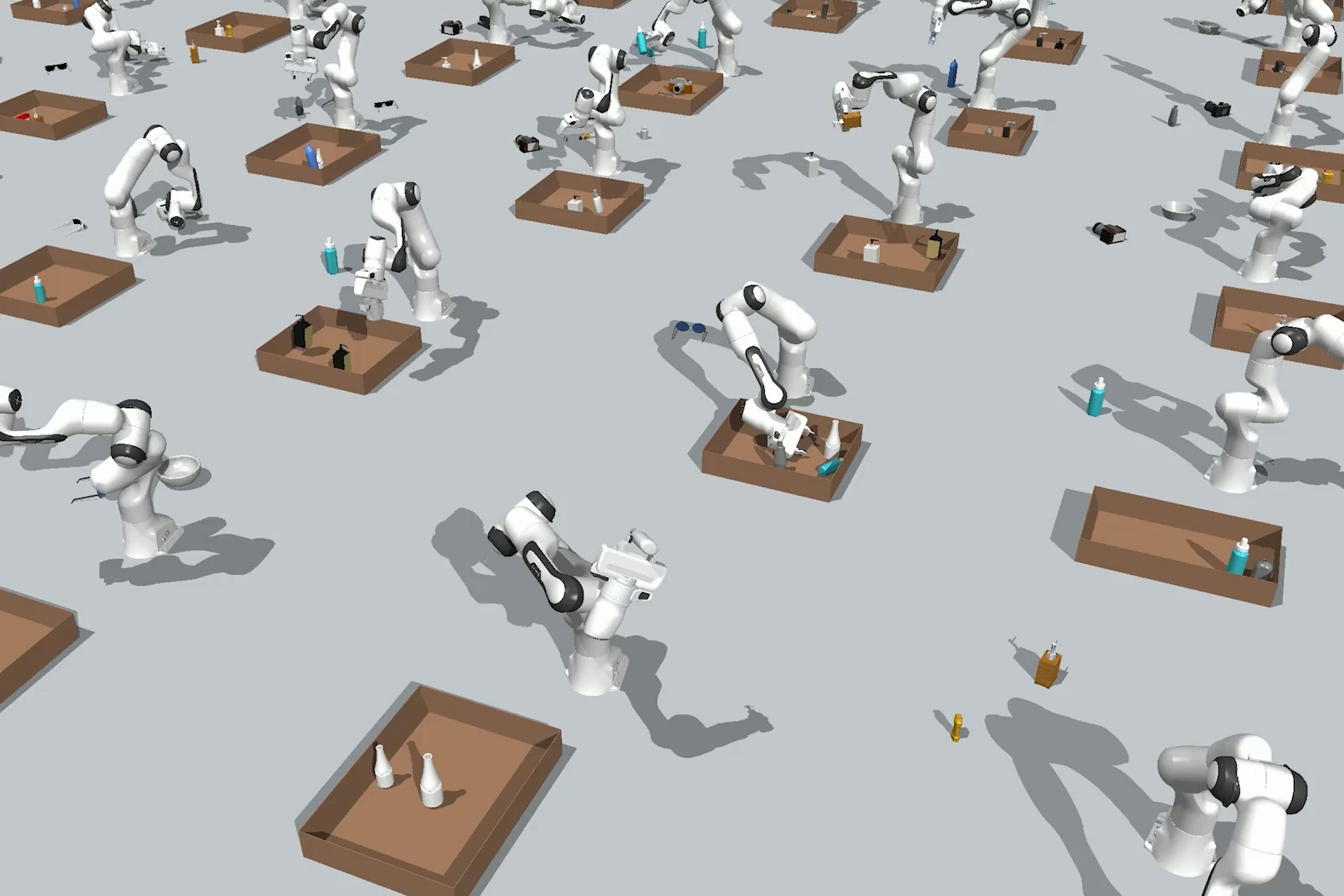
New Technique Helps Robots Pack Objects into a Tight Space
MIT researchers have developed a new machine-learning technique called Diffusion-CCSP that enables robots to efficiently solve complex packing problems. The technique uses a collection of machine-learning models, each trained to represent a specific type of constraint, to generate global solutions that take into account all constraints at once. This method outperformed other techniques, producing effective solutions faster and with a greater success rate. The researchers believe that this technique can be applied to a wide range of complex tasks in various environments, from warehouse order fulfillment to organizing objects in a home.
How Diffusion-CCSP Works
Diffusion-CCSP utilizes diffusion models, which learn to generate new data samples that resemble samples in a training dataset by iteratively refining their output. The models start with a random, poor solution and gradually improve it. They are well-suited for continuous constraint-satisfaction problems because they can compose influences from multiple models to encourage the satisfaction of all constraints. Diffusion-CCSP captures the interconnectedness of constraints by training a family of diffusion models, each representing a specific constraint type. These models work together to find solutions that jointly satisfy all constraints.
Benefits and Applications
The Diffusion-CCSP technique provides several benefits:
- Efficiency: It generates effective solutions faster than other methods.
- Generalizability: It can solve problems with novel combinations of constraints and larger numbers of objects.
- Cost-effectiveness: Training the models requires less data compared to other approaches.
Robots trained using this technique can be applied to a wide array of complex tasks, such as packing objects, stacking items, and organizing spaces. They can be used in diverse environments, including warehouses and homes.
How to Evolve Your Company with AI
If you want to stay competitive and leverage AI to redefine your way of work, consider the following steps:
- Identify Automation Opportunities: Locate key customer interaction points that can benefit from AI.
- Define KPIs: Ensure your AI endeavors have measurable impacts on business outcomes.
- Select an AI Solution: Choose tools that align with your needs and provide customization.
- Implement Gradually: Start with a pilot, gather data, and expand AI usage judiciously.
For AI KPI management advice and continuous insights into leveraging AI, connect with us at hello@itinai.com. Stay tuned on our Telegram channel t.me/itinainews or follow us on Twitter @itinaicom.
Spotlight on a Practical AI Solution: AI Sales Bot
Consider the AI Sales Bot from itinai.com/aisalesbot. It is designed to automate customer engagement 24/7 and manage interactions across all customer journey stages. Discover how AI can redefine your sales processes and customer engagement by exploring solutions at itinai.com.