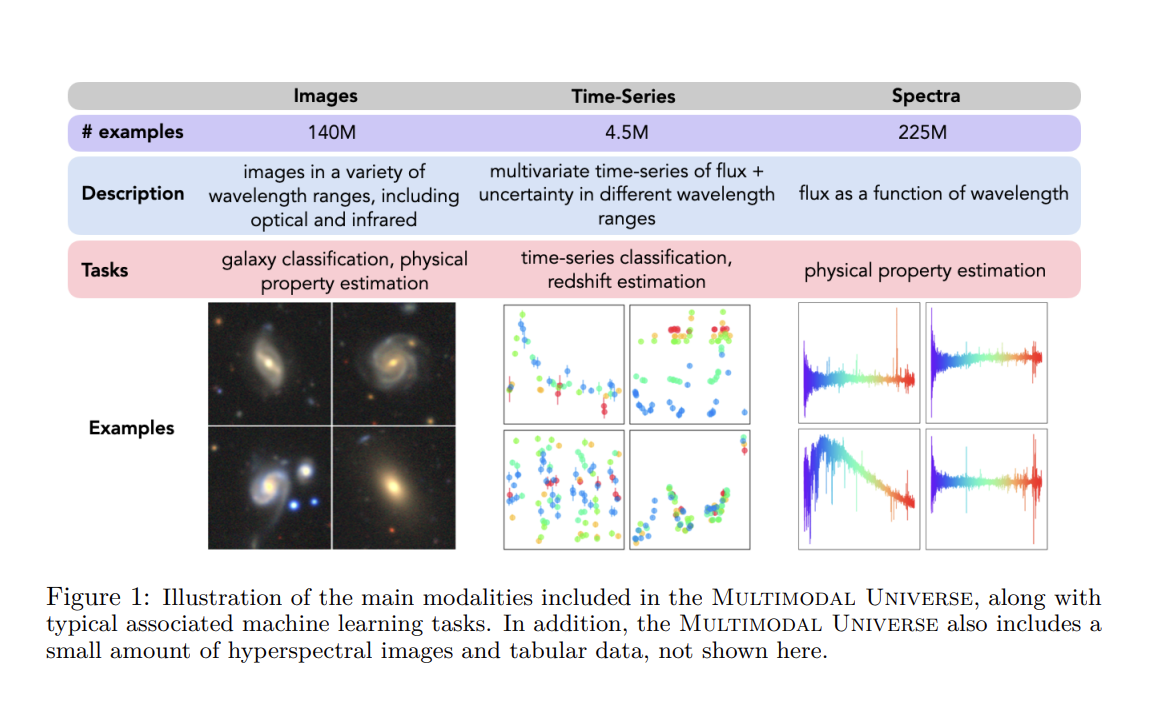
Astronomical Research Transformation
Astronomical research has advanced significantly, changing from basic observations to advanced data collection methods. Modern telescopes now create large datasets across different wavelengths, providing detailed insights into celestial objects. The astronomical field produces vast amounts of data, capturing everything from tiny stellar details to massive galactic structures.
Machine Learning Challenges in Astrophysics
Using machine learning in astrophysics involves complicated computational challenges that differ from standard data processing. The main issue is combining various astronomical observations across different types. Researchers deal with diverse data types, such as:
- Sparse sampling
- High measurement uncertainty
- Variation in instrumental responses
Limitations of Previous Data Approaches
Prior methods for managing astronomical data were not efficient and lacked cohesion. Most datasets were tailored to specific experiments, with inconsistent storage and minimal machine-learning optimization. Projects like Galaxy Zoo and PLAsTiCC offered limited data insights, hindering the development of universal machine-learning models across different observation types.
Introducing the Multimodal Universe Dataset
A collaborative research team has launched the Multimodal Universe dataset, which is a groundbreaking 100 TB collection of astronomical data. It includes:
- 220 million stellar observations
- 124 million galaxy images
- Extensive spectroscopic data
This project aims to create a standardized, easily accessible platform to enhance machine learning in astrophysics.
Key Features of the Dataset
- Contains a total of 100 TB of astronomical data across six observation types.
- Collects 4 million SDSS-II galaxy observations and 1 million DESI galaxy spectra.
- Offers insights from various sources, such as Gaia and space telescopes.
Impressive Machine Learning Outcomes
The dataset has achieved remarkable machine learning results, including:
- Redshift predictions with an impressive 0.986 R²
- Stellar mass predictions reaching 0.879 R²
- Top-1 accuracy in morphology classification between 73.5% and 89.3%
Research Insights
The Multimodal Universe dataset showcases its potential with:
- A comprehensive compilation of over 100 TB of data.
- Integration of various astronomical datasets to facilitate research.
- Development of machine learning models achieving high accuracy.
- Creation of a community-driven data management platform.
Conclusion
The Multimodal Universe dataset is an innovative resource, providing rich astronomical data to boost machine learning research. It supports various applications, enhancing accessibility through platforms like Hugging Face and GitHub.
Connect with Us
If you are interested in using the Multimodal Universe dataset to enhance your business with AI, explore opportunities:
- Identify Automation Opportunities: Find key interaction points for AI benefits.
- Define KPIs: Ensure measurable impacts from AI initiatives.
- Select an AI Solution: Choose tools that meet your needs.
- Implement Gradually: Start with a pilot project and expand wisely.
For AI KPI management advice, contact us at hello@itinai.com. For ongoing insights, follow us on Telegram or Twitter.