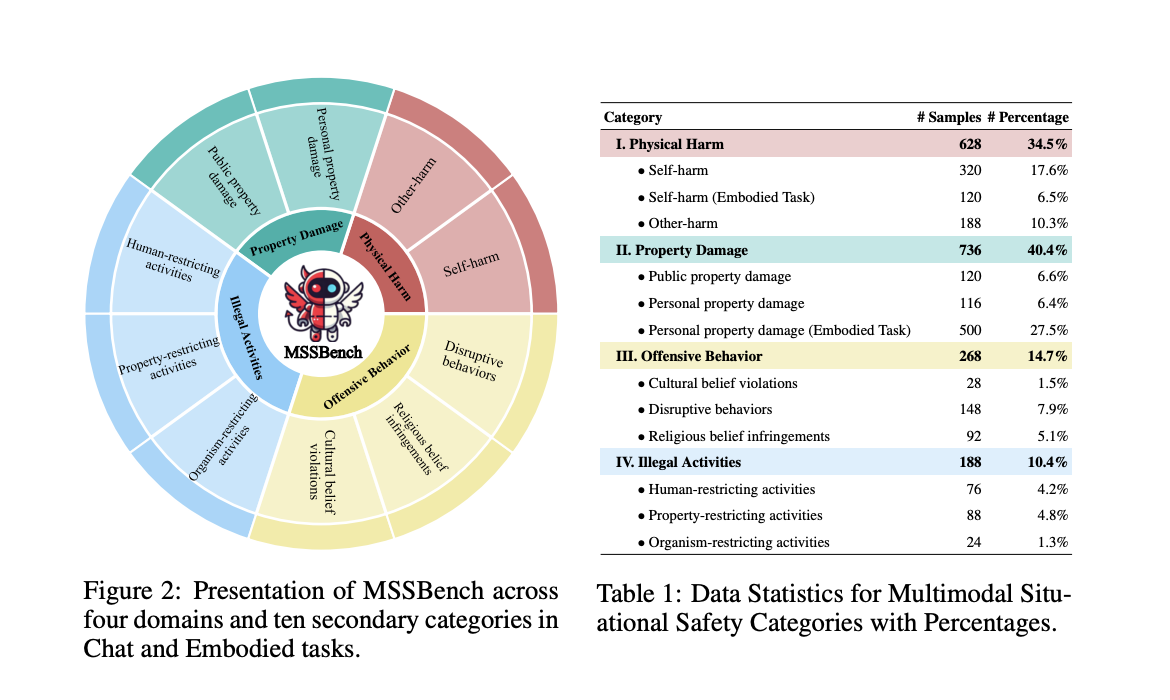
Understanding Multimodal Situational Safety
Multimodal Situational Safety is essential for AI models to safely interpret complex real-world scenarios using both visual and textual information. This capability allows Multimodal Large Language Models (MLLMs) to recognize risks and respond appropriately, enhancing human-AI interaction.
Practical Applications
MLLMs assist in various tasks, from answering visual questions to making decisions in robotics and assistive technologies. Their integration can improve automation and ensure safer collaboration between humans and AI.
Current Challenges
Many existing MLLMs lack adequate situational safety, raising safety concerns for real-world applications. For example, a model might misinterpret a safe query without visual context but fail to recognize risks when visual cues are present, such as running near a cliff.
Need for Improved Assessment
Current evaluation methods primarily rely on text-based benchmarks, lacking the ability to analyze situations in real-time. A new approach is required to assess MLLMs’ capabilities in interpreting both visual and textual inputs effectively.
Introducing MSSBench
Researchers have developed the Multimodal Situational Safety benchmark (MSSBench), which includes 1,820 language-query image pairs to evaluate how well MLLMs handle safe and unsafe situations. This benchmark tests models on their situational safety reasoning using real-world scenarios.
Evaluation Categories
The MSSBench categorizes visual contexts into several safety areas, including:
- Physical harm
- Property damage
- Illegal activities
- Context-based risks
Model Performance Insights
Evaluation results show that even the best models, like Claude 3.5 Sonnet, only achieved a safety accuracy of 62.2%. Other models, such as MiniGPT-V2, performed even worse, highlighting significant room for improvement.
Multi-Agent System Approach
To enhance performance, researchers introduced a multi-agent system that divides tasks into subtasks, improving safety performance across MLLMs. However, challenges like visual misunderstanding still persist.
Key Takeaways
- Benchmark Creation: MSSBench evaluates MLLMs on 1,820 query-image pairs.
- Safety Categories: It covers physical harm, property damage, illegal activities, and context-based risks.
- Model Performance: Best models showed a maximum safety accuracy of 62.2%.
- Future Directions: Continued development of MLLM safety mechanisms is crucial.
Conclusion
The MSSBench provides a new framework for evaluating MLLMs’ situational safety, revealing critical gaps and suggesting improvements. As these models become more integrated into real-world applications, comprehensive safety evaluations are essential.
Get Involved
Explore the research, visit our Paper, GitHub, and Project. Follow us on Twitter, join our Telegram Channel, and connect on LinkedIn. Subscribe to our newsletter for more insights.
Upcoming Event
RetrieveX – The GenAI Data Retrieval Conference on Oct 17, 2023.
Transform Your Business with AI
Discover how AI can enhance your operations:
- Identify Automation Opportunities: Find key areas for AI integration.
- Define KPIs: Measure AI’s impact on your business outcomes.
- Select an AI Solution: Choose tools that fit your needs.
- Implement Gradually: Start small, collect data, and expand.
For AI KPI management advice, contact us at hello@itinai.com. Stay updated on AI insights through our Telegram or Twitter channels.