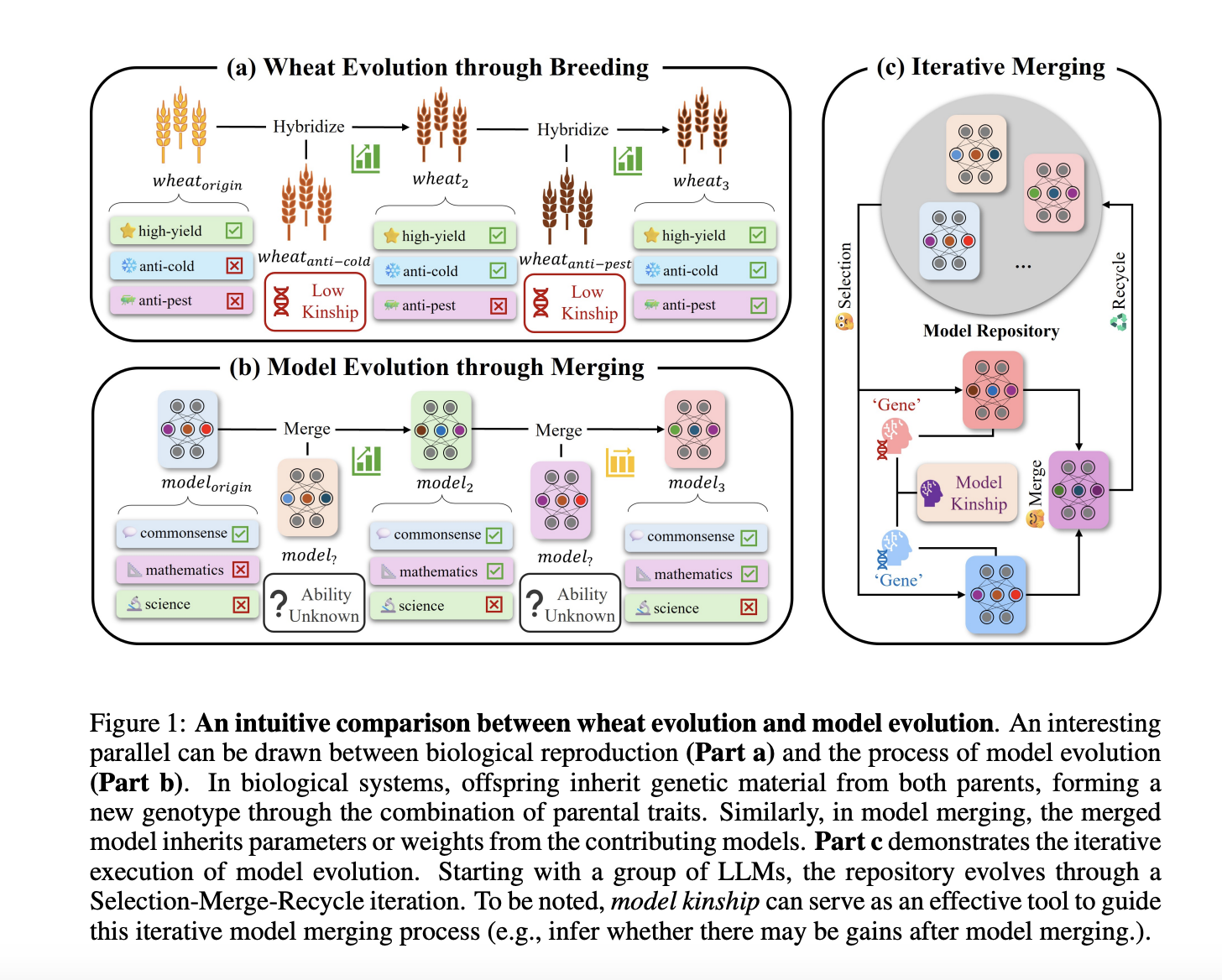
Understanding Model Kinship in Large Language Models
Challenges with Current Approaches
Large Language Models (LLMs) are increasingly popular, but fine-tuning separate models for each task can be resource-intensive. Researchers are now looking into model merging as a solution to handle multiple tasks more efficiently.
What is Model Merging?
Model merging combines several expert models to work on different tasks simultaneously. This method shows promise for improving LLM capabilities. However, the merging process often relies on trial and error, requiring human expertise to navigate challenges.
Innovative Techniques for Merging
Researchers have developed various strategies to enhance model merging, including:
– **Weight Averaging**: A method to combine model checkpoints effectively.
– **Linear Mode Connectivity (LMC)**: A technique that improves the merging of fine-tuned models.
– **Task Vectors and Parameter Interference Reduction**: Techniques like TIES and DARE help prevent conflicts during merging.
Recent Advances in Model Evolution
New approaches, such as CoLD Fusion and automated merging tools, aim to optimize model combinations. These innovations help uncover patterns in the merging process that might be overlooked.
Introducing Model Kinship
Researchers from Zhejiang University and the National University of Singapore have introduced the concept of **model kinship**, inspired by evolutionary biology. This metric assesses the relatedness between LLMs, providing insights that can improve merging strategies.
Key Findings from Research
The study identifies two stages in the merging process:
1. **Learning Stage**: Significant performance improvements occur.
2. **Saturation Stage**: Improvements plateau, indicating optimization challenges.
To address these challenges, the researchers propose **Top-k Greedy Merging with Model Kinship**, which enhances the merging process.
Practical Applications and Benefits
The research highlights several practical contributions:
– **Model Kinship**: A tool for assessing relatedness between LLMs.
– **Empirical Analysis**: Insights into model evolution through iterative merging.
– **Improved Efficiency**: The kinship-based method showed better performance over time, escaping local optima traps.
Additionally, model kinship can serve as an early stopping criterion, improving efficiency by about 30% without sacrificing performance.
How to Leverage AI for Your Business
To stay competitive and harness the power of AI, consider these steps:
– **Identify Automation Opportunities**: Find key areas in customer interactions that can benefit from AI.
– **Define KPIs**: Ensure your AI initiatives have measurable impacts.
– **Select the Right AI Solution**: Choose tools that fit your needs and allow for customization.
– **Implement Gradually**: Start small, gather data, and expand your AI usage wisely.
For more insights on AI implementation, connect with us at hello@itinai.com or follow us on our social media channels.
Join Our Community
Stay updated with the latest in AI by joining our newsletter, Telegram channel, and LinkedIn group. Don’t miss out on our upcoming live webinar on October 29, 2024, about the best platform for serving fine-tuned models.
Explore More
Discover how AI can transform your sales processes and customer engagement at itinai.com.