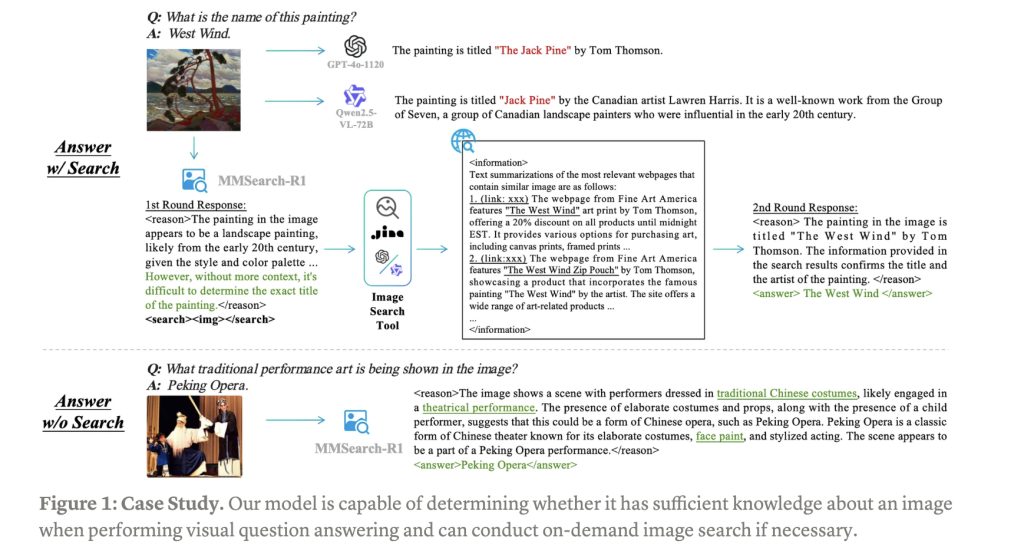
MMSearch-R1: Enhancing AI Capabilities in Business
Introduction to Large Multimodal Models (LMMs)
Large Multimodal Models (LMMs) have made significant strides in understanding and processing visual and textual data. However, they often face challenges when dealing with complex, real-world knowledge, particularly when it comes to information that is not included in their training data. This limitation can lead to inaccuracies, known as “hallucinations,” which can undermine their reliability in critical applications.
Challenges in Current AI Systems
While Retrieval-Augmented Generation (RAG) has been a common solution to enhance LMMs, it comes with its own set of challenges. The separation of retrieval and generation processes can hinder overall optimization, leading to unnecessary delays and increased operational costs. Furthermore, existing methods often struggle to balance computational efficiency with the accuracy of responses.
Innovative Solutions through Reinforcement Learning
Recent advancements in reinforcement learning (RL) have shown promise in overcoming these limitations. For instance, models like OpenAI’s o-series and Kimi K-1.5 have demonstrated improved reasoning capabilities. However, integrating external knowledge retrieval with generation remains a challenge.
Key Research Questions
- Can LMMs learn to recognize their knowledge boundaries and effectively use search tools?
- How effective and efficient is the RL approach in enhancing model performance?
- Can this RL framework lead to the development of robust multimodal intelligent behaviors?
Introducing MMSearch-R1
MMSearch-R1 is a groundbreaking approach that equips LMMs with active image search capabilities using an end-to-end reinforcement learning framework. This system enhances visual question answering (VQA) by enabling models to autonomously engage with image search tools, making informed decisions about when to initiate searches and how to process the retrieved information effectively.
Architecture and Dataset
The architecture of MMSearch-R1 combines advanced data engineering with reinforcement learning techniques, utilizing the FactualVQA dataset. This dataset includes 50,000 visual concepts and is designed to ensure reliable evaluation through automated methods. It provides a balanced mix of queries that can be answered with or without image search assistance.
Performance and Efficiency
Experimental results indicate that MMSearch-R1 significantly enhances performance across various benchmarks. The system not only expands the knowledge boundaries of LMMs but also learns to make intelligent decisions regarding when to use external tools. This leads to improved accuracy while maintaining resource efficiency.
Comparative Analysis
Reinforcement learning has proven to be more efficient than traditional supervised fine-tuning methods. For example, when applied to Qwen2.5-VL-Instruct models, the RL approach achieved superior results using only half the training data required by conventional methods. This efficiency highlights the potential of RL in optimizing model performance while conserving resources.
Conclusion
MMSearch-R1 demonstrates that outcome-based reinforcement learning can effectively train LMMs to utilize active image search capabilities. This innovative approach allows models to autonomously decide when to access external visual knowledge, thereby enhancing their computational efficiency and overall performance. The promising results pave the way for the development of future multimodal systems that can dynamically interact with the visual world.
Call to Action
Explore how artificial intelligence can transform your business processes. Identify areas where automation can add value, establish key performance indicators (KPIs) to measure the impact of your AI investments, and start with small projects to gauge effectiveness before scaling up. For guidance on implementing AI in your business, contact us at hello@itinai.ru.