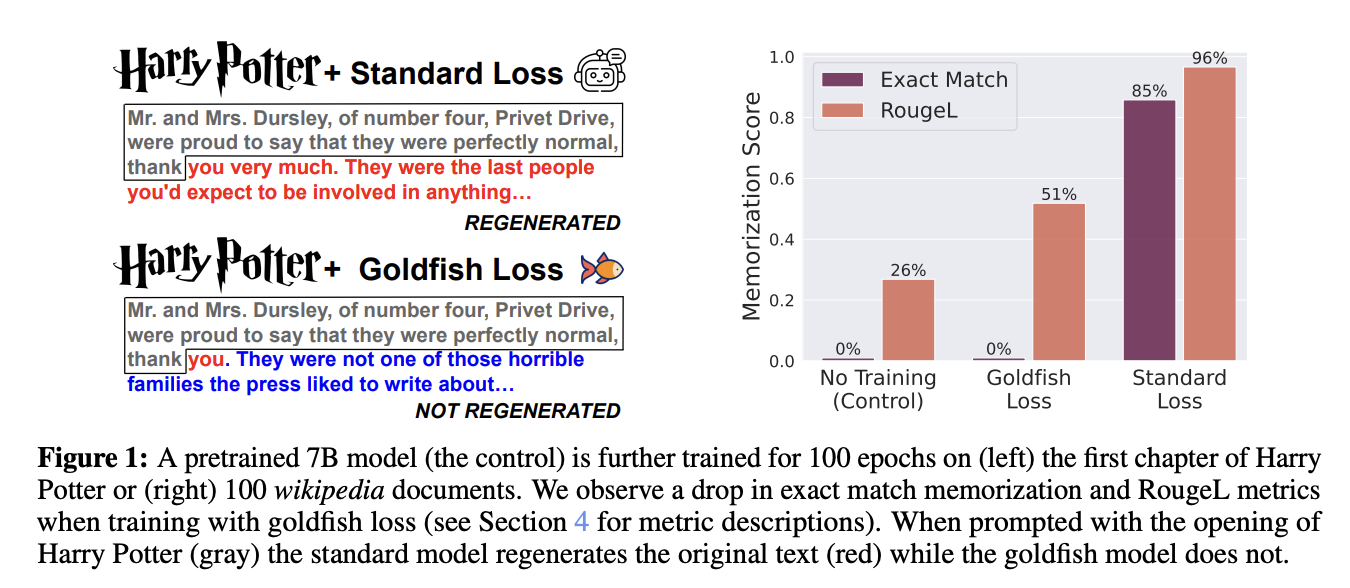
Practical Solutions for Mitigating Memorization in Language Models
Addressing Privacy and Copyright Risks
Language models can pose privacy and copyright risks by memorizing and reproducing training data. This can lead to conflicts with licensing terms and exposure of sensitive information. To mitigate these risks, it’s crucial to address memorization during the initial model training.
Goldfish Loss Training Technique
Researchers have developed the “goldfish loss” training technique to reduce memorization in language models. This method excludes a random subset of tokens from the loss computation during training, preventing the model from memorizing exact sequences from its training data. Extensive experiments have shown that goldfish loss significantly reduces memorization with minimal impact on performance.
Quantifying and Mitigating Memorization
Researchers have explored various methods to quantify and mitigate memorization in language models, including extracting training data via prompts, differentially private training, and regularization methods. Innovative approaches like consistent token masking have also been developed to prevent the model from learning specific data passages verbatim.
Enhancing Privacy in Industrial Applications
The goldfish loss effectively prevents memorization in large language models across different training scenarios. Despite limitations against certain adversarial extraction methods, it remains a viable strategy for enhancing privacy in industrial applications.
Evolve Your Company with AI
Discover how AI can redefine your way of work and redefine your sales processes and customer engagement. Identify automation opportunities, define KPIs, select an AI solution, and implement gradually to leverage AI for your business.
For AI KPI management advice and continuous insights into leveraging AI, connect with us at hello@itinai.com and stay tuned on our Telegram t.me/itinainews or Twitter @itinaicom.