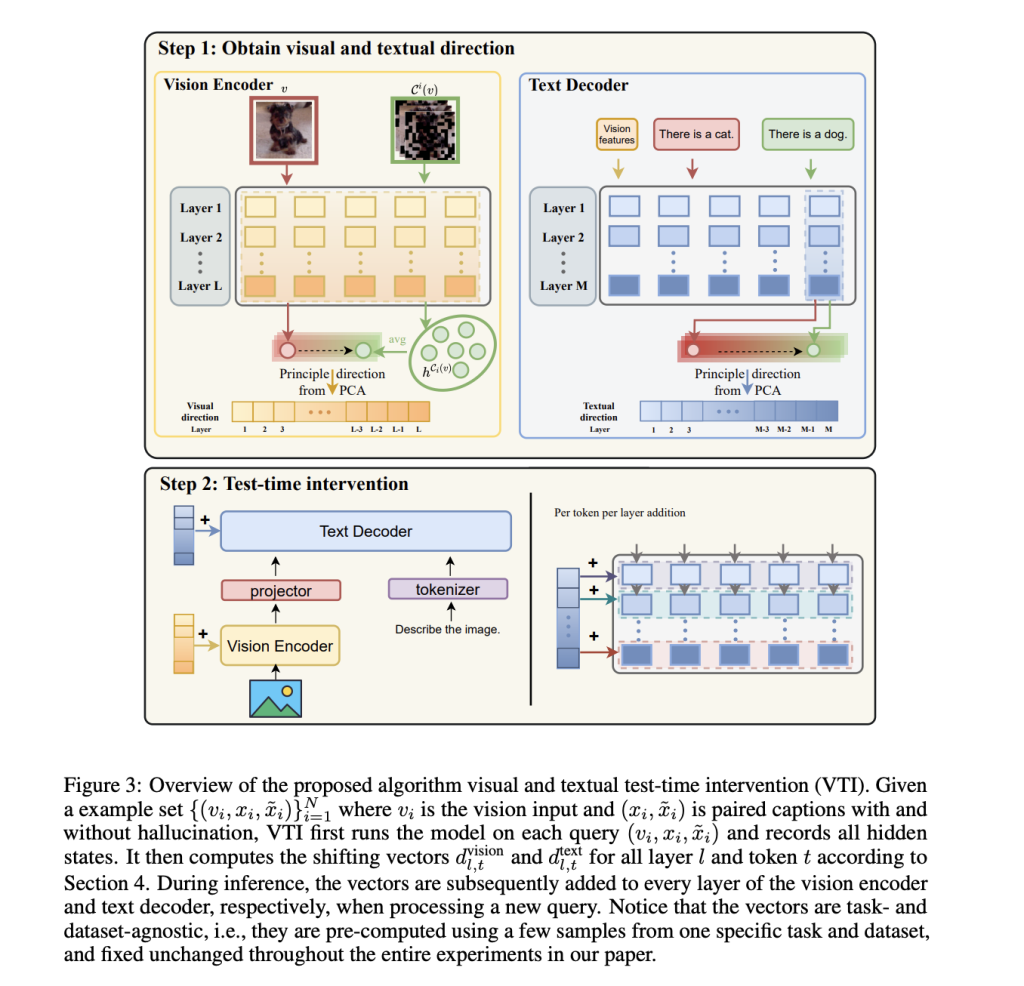
Mitigating Hallucinations in Large Vision-Language Models: Practical Business Solutions
Understanding the Challenge of Hallucinations in LVLMs
Large Vision-Language Models (LVLMs) are powerful tools that combine visual and textual data to perform tasks such as image captioning and visual question answering. However, they often produce inaccurate outputs, known as hallucinations, where the generated text does not accurately reflect the visual input. This misalignment can occur due to various factors, including biases in model training and the distinct nature of visual and textual data processing.
Strategies for Mitigating Hallucinations
1. Training-Based Approaches
Training-based methods aim to enhance model accuracy by aligning outputs with actual data through additional supervision. However, these approaches require significant datasets and computational power, making them less feasible for many businesses.
2. Training-Free Methods
In contrast, training-free methods, such as self-feedback correction and auxiliary model integration, offer efficient alternatives. These methods improve the decoding process and can significantly reduce hallucinations without the need for extensive re-training.
Case Study: Visual and Textual Intervention (VTI)
Researchers from Stanford University developed a technique called Visual and Textual Intervention (VTI) to address hallucinations in LVLMs. VTI stabilizes the vision features by adjusting the latent space representations during inference, which allows for improved accuracy without additional training costs. Experimental results indicate that VTI outperforms traditional methods across various benchmarks, underscoring its potential for enhancing LVLM reliability.
Practical Applications for Businesses
To leverage the advancements in LVLMs and mitigate hallucinations, businesses can implement the following strategies:
- Identify Automation Opportunities: Look for processes that can be automated using AI, particularly in customer interactions where AI can add significant value.
- Establish Key Performance Indicators (KPIs): Determine essential metrics to evaluate the effectiveness of AI investments and ensure they positively impact business outcomes.
- Select Customizable Tools: Choose AI tools that can be tailored to meet specific business needs and objectives.
- Start Small: Begin with a pilot project to gather data on effectiveness before scaling up AI applications within the organization.
Conclusion
The research on VTI presents a promising method for mitigating hallucinations in LVLMs, demonstrating that effective stabilization of vision features can lead to more accurate and reliable outputs. By adopting practical strategies for implementing AI, businesses can enhance their operations and capitalize on the transformative potential of artificial intelligence. For further guidance on managing AI in business, please reach out to us at hello@itinai.ru.