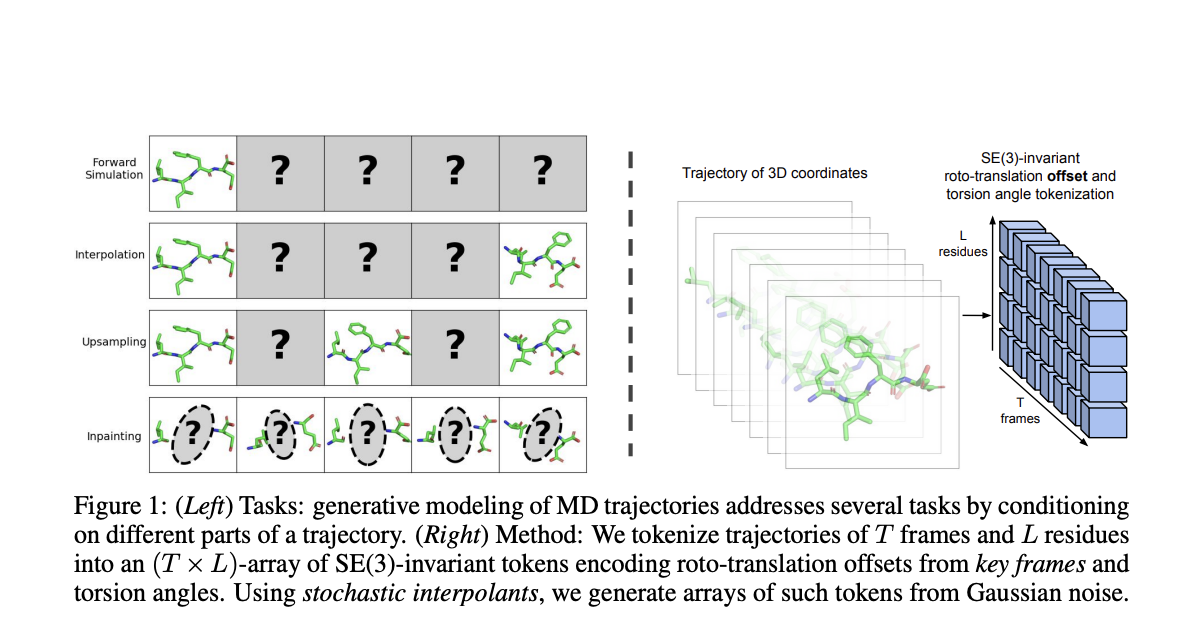
Practical Solutions and Value of Generative Modeling in Molecular Dynamics
Overview:
Molecular dynamics (MD) is essential for studying molecular systems at the atomic level. However, it can be computationally expensive. Generative modeling offers a solution to speed up simulations without losing accuracy.
Key Tasks and Solutions:
- Forward Simulation: Predict chemical system evolution from an initial configuration.
- Transition Paths Sampling: Generate routes explaining molecular state shifts.
- Trajectory Upsampling: Increase temporal resolution for quicker molecular motions.
- Inpainting: Predict missing molecular components for molecular design.
Benefits:
- Enables dynamics-conditioned molecular design.
- Enhances capabilities in molecular simulations for fields like drug discovery and materials research.
Impact and Evaluation:
Generative models have shown effectiveness in generating realistic molecular trajectories, even in complex biological systems like proteins.
Conclusion:
Generative modeling in molecular dynamics unlocks additional value, offering new possibilities in molecular design and simulations.
AI Integration Recommendations:
- Identify Automation Opportunities.
- Define KPIs for measurable impacts.
- Select AI solutions aligned with your needs.
- Implement AI gradually for optimal results.
Contact Us:
For AI KPI management advice, reach out at hello@itinai.com. Stay updated on AI insights through our Telegram t.me/itinainews or Twitter @itinaicom.