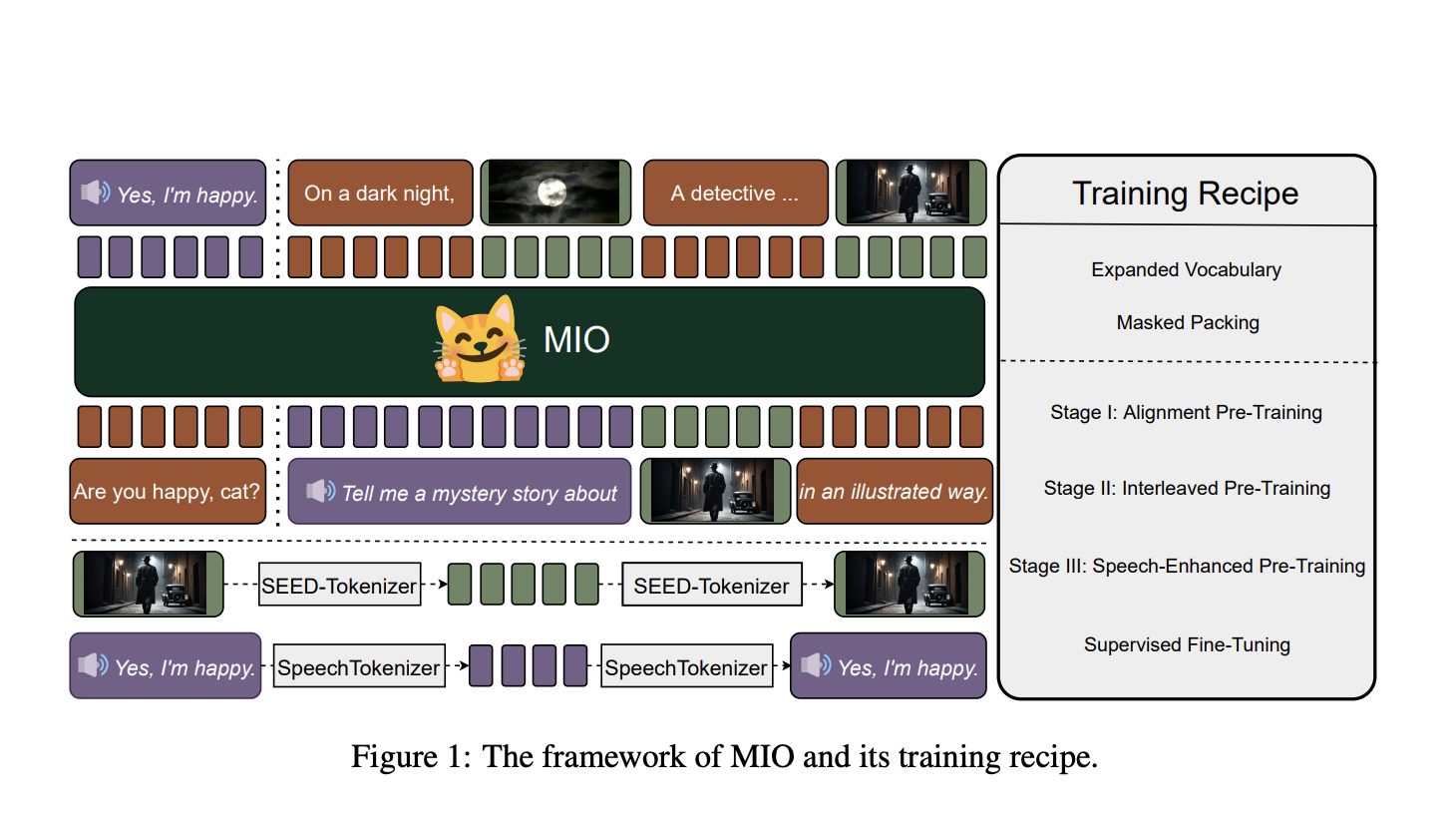
Multimodal Models: Enhancing AI Capabilities
Overview
Multimodal models combine different data types like text, speech, images, and videos to improve AI systems’ understanding and performance. They mimic human-like perception and cognition, enabling tasks such as visual question answering and interactive storytelling.
Challenges and Solutions
Current multimodal models face limitations in processing diverse data types and generating interleaved content. To address this, new approaches like MIO have been developed, offering open-source, any-to-any multimodal capabilities for comprehensive interactions.
Training Process
MIO undergoes a four-stage training process, aligning tokens across modalities and enhancing its understanding and generation abilities. This process includes alignment pre-training, interleaved pre-training, speech-enhanced pre-training, and supervised fine-tuning for various tasks.
Performance
Experimental results show that MIO outperforms existing models in tasks like visual question answering, speech recognition, and video understanding. Its robustness and efficiency in handling complex multimodal interactions make it a valuable tool for AI research and development.
Value Proposition
MIO represents a significant advancement in multimodal AI, offering a powerful solution for integrating and generating content across different modalities. Its performance and comprehensive training process set new standards in AI research, paving the way for future innovations.