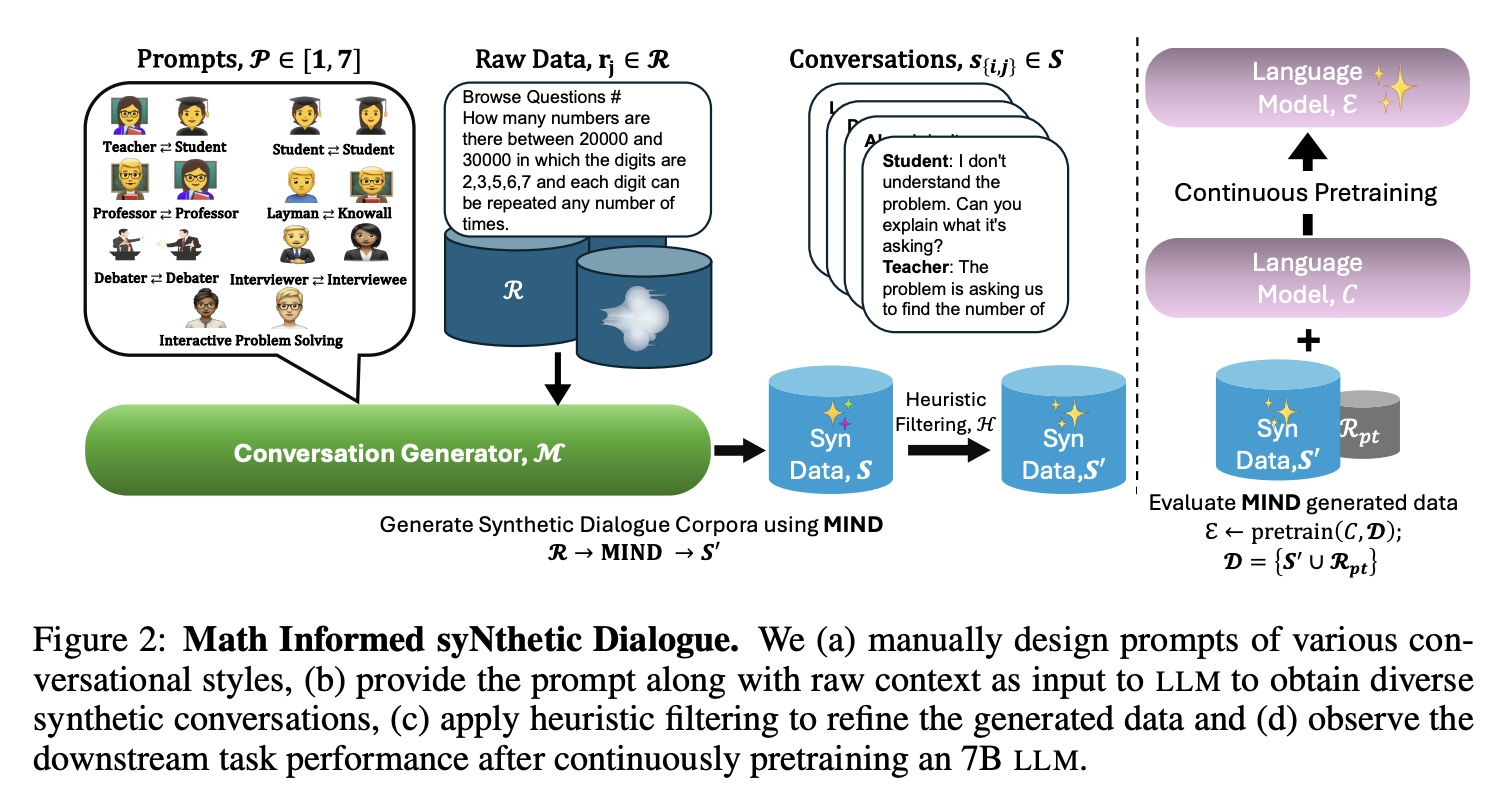
Understanding Large Language Models (LLMs)
Large language models (LLMs) can understand and create text that resembles human language. However, they struggle with mathematical reasoning, especially in complex problems that require logical, step-by-step thinking. Enhancing their mathematical skills is essential for both academic and practical applications, such as in science, finance, and technology.
Challenges in Mathematical Reasoning
LLMs excel in general tasks but find intricate mathematical problems challenging due to:
- A lack of structured, high-quality mathematical data during training.
- Insufficient exposure to complex problems formatted in a stepwise manner.
- The absence of curated datasets specific to mathematical reasoning.
Current Solutions and Limitations
To improve LLMs, researchers are using synthetic data to enhance training. However, existing methods often fail to provide the detailed, step-by-step problem-solving processes needed for effective learning in mathematics. This lack of structure in synthetic data limits its usefulness for developing LLMs’ mathematical skills.
Introducing MIND: A New Approach
Researchers from NVIDIA, Carnegie Mellon University, and Boston University have developed a new method called MIND (Math Informed syNthetic Dialogue). This technique creates synthetic conversations that mimic the step-by-step process of solving complex math problems. MIND uses a large dataset called OpenWebMath to generate structured dialogues that enhance LLMs’ reasoning abilities.
How MIND Works
The MIND method prompts an LLM with raw mathematical text and instructs it to break down problems into conversational turns. This structured approach allows the model to focus on each component logically. The researchers refined these conversations to ensure relevance and accuracy, helping models tackle multi-step problems effectively.
Results of MIND Implementation
Experiments showed that LLMs trained with MIND data significantly outperformed those trained on raw data alone:
- 13.42% improvement in solving math word problems (GSM 8K).
- 2.30% improvement on the MATH dataset.
- 4.55% improvement in specialized knowledge tasks (MMLU).
- 2.51% increase in general reasoning tasks.
Key Benefits of MIND
- Structured dialogues improve LLMs’ ability to solve complex mathematical problems.
- Scalable and cost-effective solution for enhancing reasoning capabilities.
- Combines raw and synthetic data for a comprehensive learning experience.
Conclusion
The MIND research presents a groundbreaking method to boost the mathematical reasoning skills of LLMs. By generating diverse synthetic dialogues, MIND fills the gap left by traditional training methods that rely on unstructured data. This structured approach enables LLMs to tackle complex problems logically and effectively, enhancing overall AI performance.
Get Involved
To learn more, check out the research paper. Follow us on Twitter, join our Telegram Channel, and connect with our LinkedIn Group. If you appreciate our work, subscribe to our newsletter and join our 50k+ ML SubReddit.
Upcoming Webinar
Live Webinar – Oct 29, 2024: Discover the best platform for serving fine-tuned models: Predibase Inference Engine.
Transform Your Business with AI
Stay competitive by leveraging MIND to enhance your AI capabilities:
- Identify automation opportunities in customer interactions.
- Define measurable KPIs for your AI initiatives.
- Select AI solutions that meet your specific needs.
- Implement AI gradually, starting with pilot projects.
For AI KPI management advice, contact us at hello@itinai.com. For continuous insights, follow us on Telegram or Twitter.
Explore AI Solutions
Discover how AI can transform your sales processes and customer engagement at itinai.com.