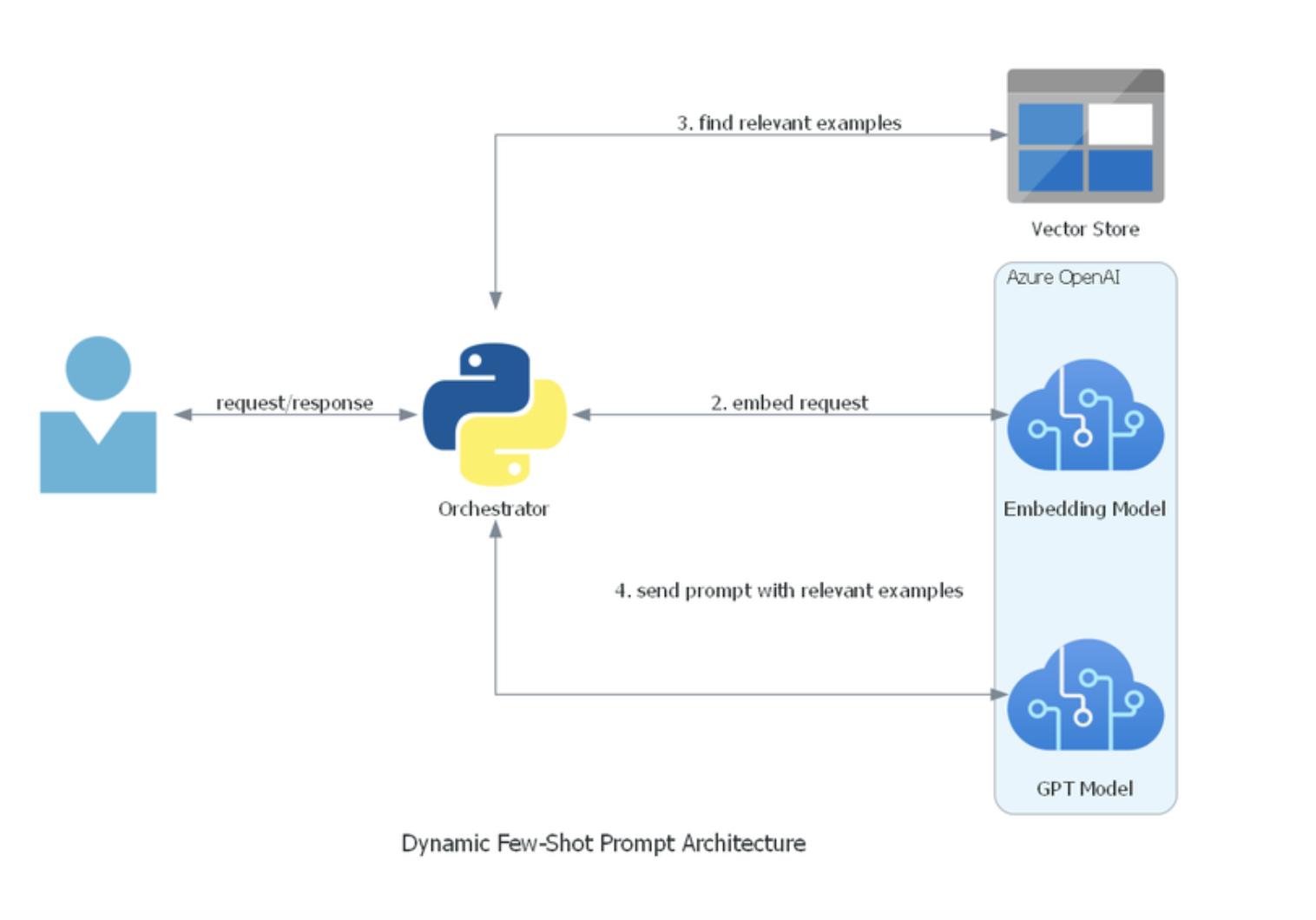
Practical Solutions and Value of Microsoft’s Dynamic Few-Shot Prompting
Understanding Few-Shot Prompting
Microsoft’s innovative technique with Azure OpenAI optimizes few-shot learning by selecting relevant examples for user input, improving performance and efficiency in NLP tasks.
Challenges and the Dynamic Solution
Dynamic few-shot prompting overcomes scalability issues of static prompting by selecting the most relevant examples for user input, enhancing model efficiency and reducing computational costs.
The Role of Vector Stores and OpenAI Embeddings
Vector store, embedding model, and GPT model work together to ensure contextually relevant examples are included in the prompt, improving model responses and precision.
Implementing the Dynamic Few-Shot Technique
Implementing dynamic few-shot prompting with Azure OpenAI is straightforward, involving defining examples, indexing them, and using the ‘SemanticSimilarityExampleSelector’ class for selection.
Use Cases and Benefits
Dynamic few-shot prompting improves model accuracy, reduces computational overhead, and allows for easy addition of new examples, making it cost-efficient and performance-optimized for various NLP applications.
Conclusion
Microsoft’s Dynamic Few-Shot Prompting with Azure OpenAI offers an efficient and contextually aware model for high-quality outputs in NLP applications, representing a paradigm shift in few-shot learning implementation.
For more details, visit MarkTechPost.