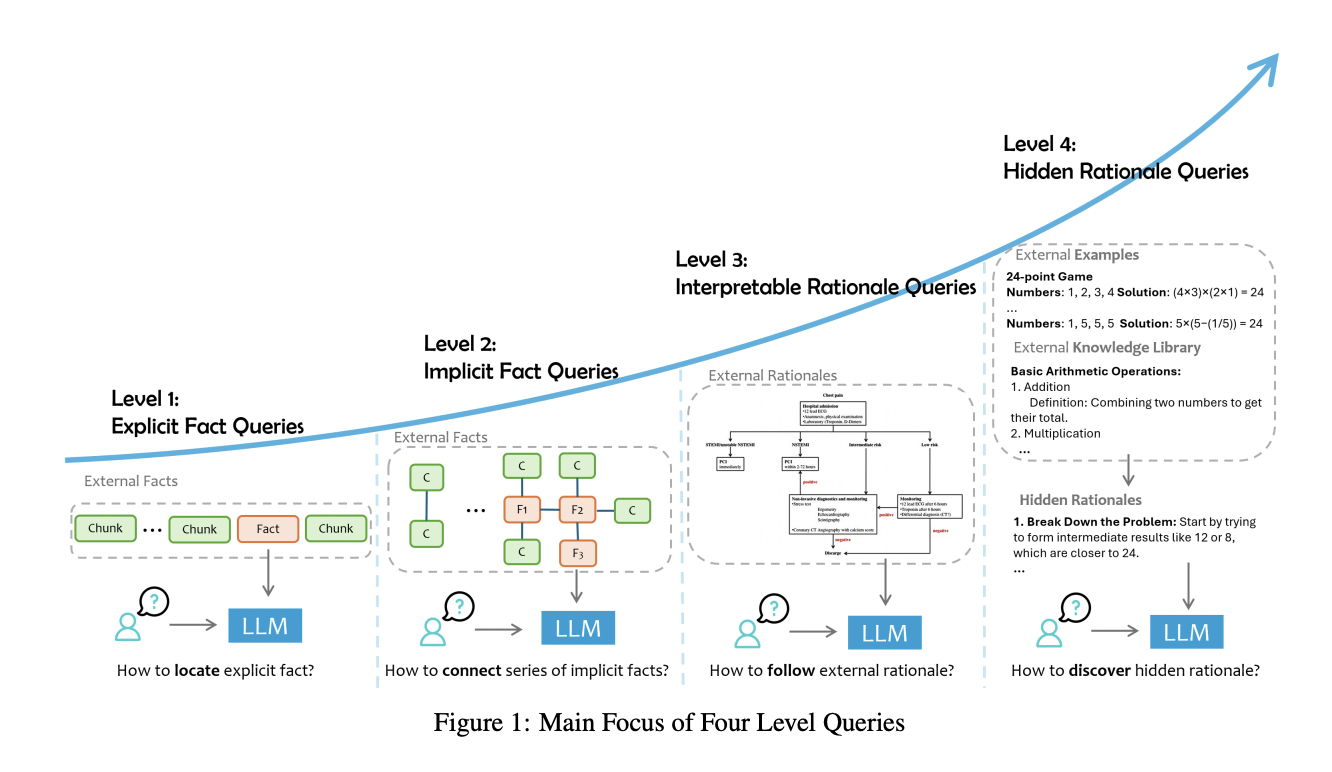
Practical Solutions for Enhancing Large Language Models (LLMs)
Overview
Large language models (LLMs) have transformed AI by generating human-like text and complex reasoning. However, they struggle with domain-specific tasks in sectors like healthcare, law, and finance. Enhancing LLMs with external data through techniques like Retrieval-Augmented Generation (RAG) can significantly improve their precision and effectiveness.
Challenges Addressed
LLMs face challenges in handling specialized and time-sensitive queries due to static training data. Fine-tuning and RAG techniques have made progress, but limitations like overfitting and data processing obstacles remain.
Microsoft’s Query Categorization System
Microsoft Research Asia’s method categorizes user queries for efficient data retrieval. It classifies queries into explicit facts, implicit facts, interpretable rationales, and hidden rationales, enabling tailored reasoning levels for different query types.
Results and Benefits
The approach significantly improves LLM performance in healthcare and legal analysis, reducing hallucinations and enhancing accuracy. By categorizing queries, the model retrieves relevant data more efficiently, leading to better decision-making and reliable outputs.
Conclusion
This research offers a crucial solution for deploying LLMs in specialized domains, enhancing accuracy and interpretability. By categorizing queries and integrating external data effectively, the study demonstrates improved results and paves the way for more reliable AI applications.
For more information on AI solutions and consultation, contact us at hello@itinai.com or follow us on Telegram and Twitter for continuous insights.