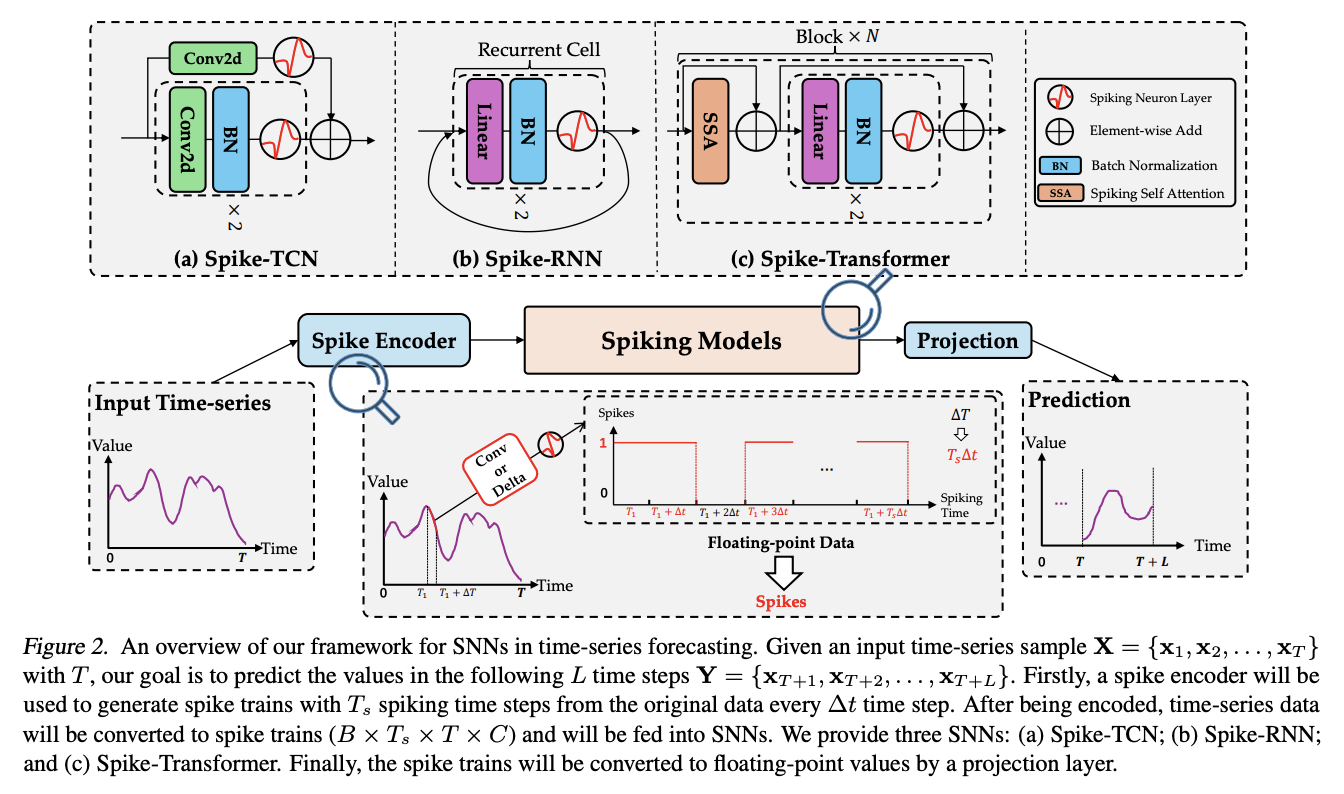
Practical Solutions for Time-Series Forecasting with Spiking Neural Networks
Efficient Temporal Alignment
Properly aligning temporal data is crucial for using SNNs in time-series forecasting. This alignment can be challenging, especially with irregular or noisy data, but it is essential for accurate modeling of temporal connections.
Difficulties in Encoding Procedures
Converting time-series data into an encoding format suitable for SNNs is a complex task. Advanced encoding techniques are required to transform continuous time-series data into discrete spikes that retain important temporal information.
Lack of Standardised Recommendations
The absence of standardized recommendations for model selection and training adds complexity to applying SNNs in time-series forecasting. This has restricted the use of SNNs in real-world applications.
Value of SNNs in Time-Series Forecasting
In recent research, Microsoft has proposed a methodology for using SNNs in time-series forecasting, addressing the challenges mentioned. The study showed that SNN-based techniques outperformed conventional forecasting methods while consuming less energy.
The research also highlighted the ability of SNNs to identify temporal connections and capture subtle temporal patterns in time-series data, demonstrating the potential of biologically inspired methods in creating more effective forecasting models.
AI Solutions for Business Transformation
Microsoft’s research suggests the potential of SNNs for energy-efficient time-series forecasting, showcasing the benefits of AI in redefining business processes. To evolve your company with AI, consider identifying automation opportunities, defining measurable KPIs, selecting suitable AI tools, and implementing AI gradually.
For AI KPI management advice and insights into leveraging AI, connect with us at hello@itinai.com or stay tuned on our Telegram t.me/itinainews and Twitter @itinaicom.
Discover how AI can redefine sales processes and customer engagement at itinai.com.