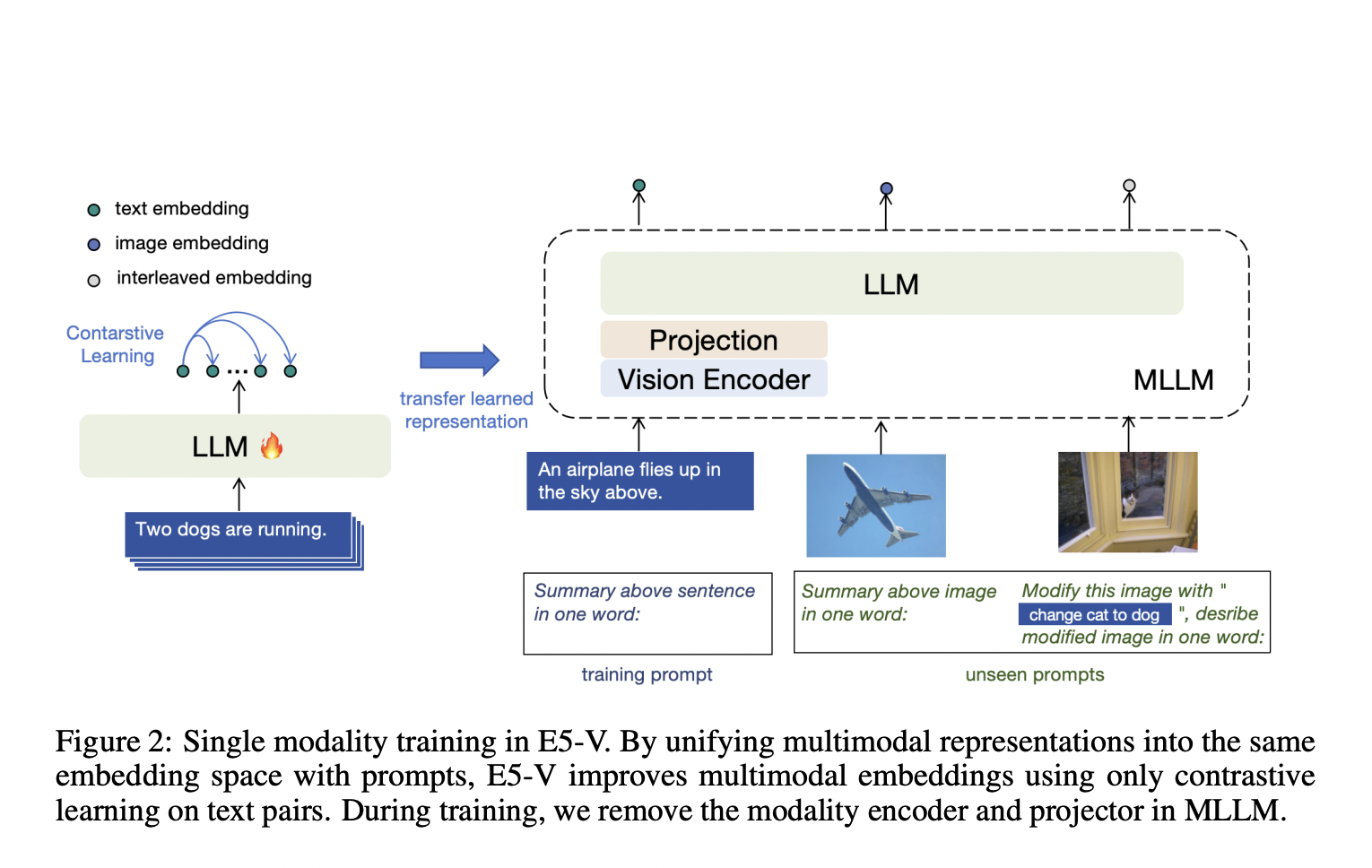
A Universal AI Framework for Multimodal Embeddings
Practical Solutions and Value
A major development in artificial intelligence, multimodal large language models (MLLMs) combine verbal and visual comprehension to produce more accurate representations of multimodal inputs. These models improve understanding of intricate relationships between various modalities, enabling sophisticated tasks requiring thorough comprehension of diverse data.
Current research includes frameworks like CLIP, which align visual and language representations using contrastive learning on image-text pairs. To address limitations in current methods, the E5-V framework was introduced to adapt MLLMs for universal multimodal embeddings. It leverages single-modality training on text pairs, significantly reducing training costs and eliminating the need for multimodal data collection.
The innovative prompt-based representation method unifies multimodal embeddings into a single space, enabling the model to handle highly accurate tasks like composed image retrieval. Across various tasks, E5-V outperforms state-of-the-art models, showcasing its superior ability to integrate visual and language information. The framework demonstrates a significant advancement in multimodal learning, revolutionizing tasks that require integrated visual and language understanding.
If you want to evolve your company with AI, stay competitive, use for your advantage Microsoft Research Introduces E5-V: A Universal AI Framework for Multimodal Embeddings with Single-Modality Training on Text Pairs. Discover how AI can redefine your way of work. Connect with us at hello@itinai.com for AI KPI management advice and continuous insights into leveraging AI.