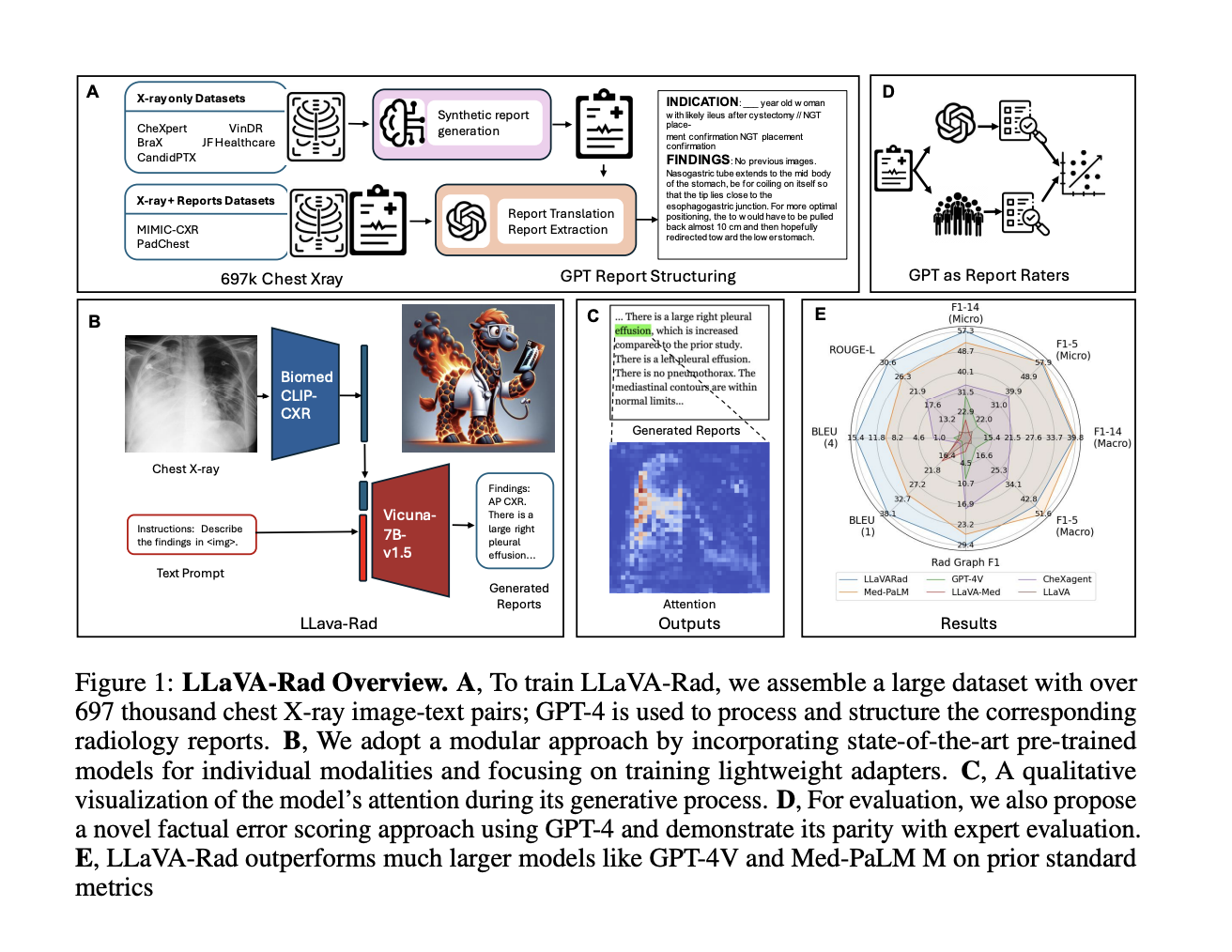
Introduction to LLaVA-Rad
Large foundation models have shown great promise in the biomedical field, especially in tasks requiring minimal labeled data. However, using these advanced models in clinical settings faces challenges such as performance gaps and high operational costs. This makes it difficult for clinicians to utilize these models effectively with patient data.
Challenges in Clinical Implementation
Recent advancements in multimodal generative AI have helped in tasks like visual question answering and radiology report generation. Yet, there are still significant hurdles:
- High Resource Requirements: Large models need substantial computational power, making them costly and environmentally taxing.
- Performance Gaps: Smaller models, while more efficient, often lag behind larger models in performance.
- Lack of Open-Source Models: There’s a shortage of accessible models and reliable evaluation methods to ensure accuracy, especially regarding hallucination detection.
Introducing LLaVA-Rad
Researchers from several prestigious institutions have developed LLaVA-Rad, a Small Multimodal Model (SMM) focused on chest X-ray imaging for generating high-quality radiology reports. Key features include:
- Efficient Training: Trained on 697,435 radiology image-report pairs using a single V100 GPU for inference.
- Modular Design: The model undergoes a structured training process in three stages: pre-training, alignment, and fine-tuning.
- High Performance: Outperforms larger models in key metrics, achieving significant improvements in radiology text evaluation.
Why LLaVA-Rad Stands Out
Despite being smaller, LLaVA-Rad excels against similar-sized models by:
- Robust Architecture: It efficiently integrates non-text data into a text-based framework.
- Consistent Results: Performs well across multiple datasets, even with new data.
- Practical Application: Its efficiency and performance make it ideal for real-world clinical use.
Significance of the Research
LLaVA-Rad marks a major step towards making advanced AI models usable in clinical settings. The model is open-source and lightweight, achieving top-tier performance in radiology report generation. Additionally, CheXprompt offers an automated evaluation method that matches expert radiologists’ accuracy, further bridging the gap between technology and clinical needs.
Engagement and Resources
Explore the following resources for more information:
Stay connected by following us on Twitter, joining our Telegram Channel, and participating in our LinkedIn Group. Join our growing ML SubReddit community as well.
Transform Your Business with AI
By adopting LLaVA-Rad, companies can enhance their operations and stay competitive. Here’s how:
- Identify Automation Opportunities: Find areas in customer interactions that can benefit from AI.
- Define KPIs: Ensure your AI initiatives have measurable business impacts.
- Select an AI Solution: Choose tools that fit your needs and allow customization.
- Implement Gradually: Start small, gather data, and scale AI use wisely.
For AI KPI management advice, reach out at hello@itinai.com. For ongoing insights, follow us on Telegram or Twitter.
Discover how AI can enhance your sales and customer engagement processes at itinai.com.