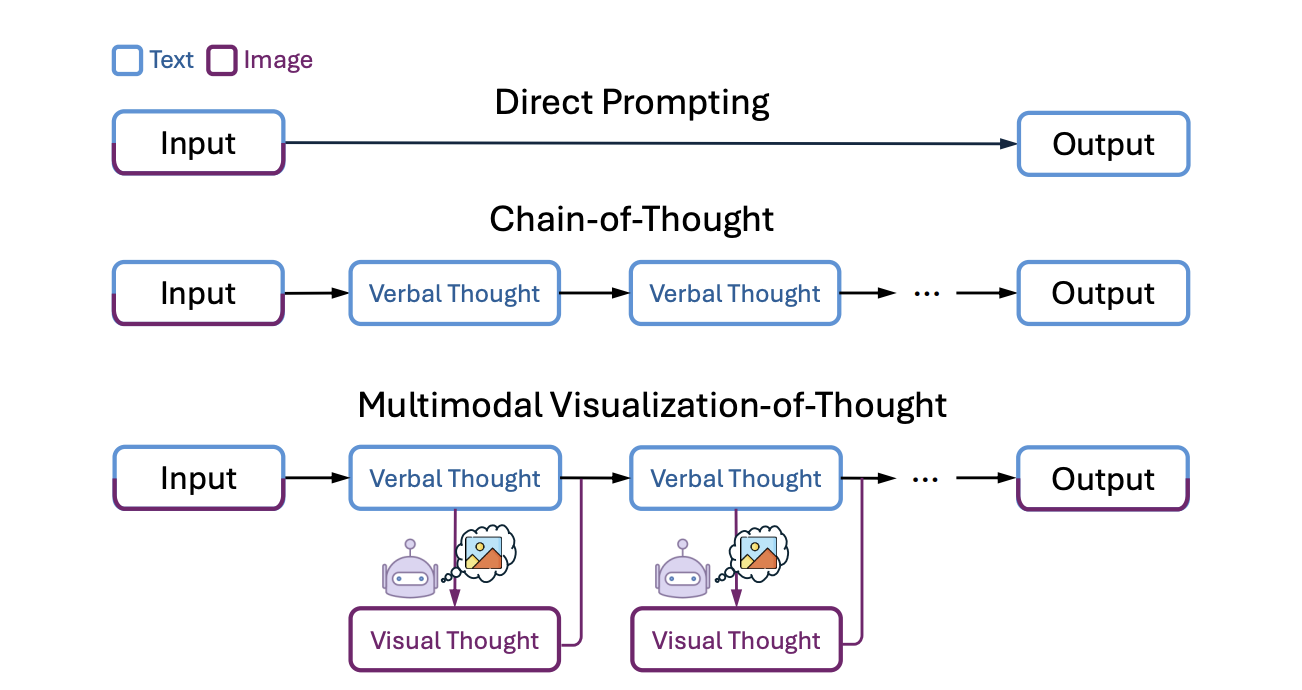
Transforming AI with Multimodal Reasoning
Introduction to Multimodal Models
The study of artificial intelligence (AI) has evolved significantly, especially with the development of large language models (LLMs) and multimodal large language models (MLLMs). These advanced systems can analyze both text and visual data, allowing them to handle complex tasks better than traditional models that rely solely on verbal reasoning.
Challenges in Current Models
However, existing models struggle to connect text and visual reasoning in real-time situations. They perform well with either text or images but can’t effectively integrate both. This limitation affects their performance in tasks that involve spatial reasoning, like navigating mazes or interpreting dynamic layouts.
Proposed Solutions
Various methods have been suggested to improve these models. One approach, called chain-of-thought (CoT) prompting, enhances reasoning through step-by-step textual explanations. However, CoT does not address tasks that require spatial understanding. Other methods use external tools for visual inputs, but these often lack flexibility and may lead to errors.
Introducing the MVoT Framework
To tackle these issues, researchers from Microsoft Research, the University of Cambridge, and the Chinese Academy of Sciences developed the Multimodal Visualization-of-Thought (MVoT) framework. MVoT allows models to create visual and verbal reasoning traces together, leading to a more comprehensive and effective approach to complex reasoning tasks.
Implementation of MVoT
Using Chameleon-7B, an autoregressive MLLM, the researchers fine-tuned MVoT for multimodal reasoning. This method closes the gap between text and image processing, enabling the model to produce visualizations that correspond with verbal reasoning. For example, when navigating a maze, the model generates visual steps that enhance understanding and performance.
Performance and Accuracy
MVoT has shown impressive results in various spatial reasoning tasks. It achieved a remarkable accuracy of 92.95% in maze navigation, surpassing traditional methods. In the MINI BEHAVIOR task, it reached 95.14% accuracy, demonstrating its effectiveness in dynamic environments. MVoT also excelled in the challenging FROZEN LAKE task with an accuracy of 85.60%.
Enhanced Interpretability
Beyond performance, MVoT improves interpretability by creating visual thought traces alongside verbal reasoning. This allows users to easily follow the model’s thought process, making it simpler to understand and validate its conclusions. This integrated approach reduces errors that can arise from relying solely on text.
Conclusion: The Future of AI Reasoning
The MVoT framework marks a significant advancement in AI reasoning capabilities by uniting text and vision in complex tasks. By aligning visual reasoning with textual processing, MVoT bridges existing gaps and sets the stage for developing more sophisticated AI systems for real-world applications.
Next Steps
Check out the research paper for more insights into this groundbreaking work. For businesses looking to leverage AI, consider these strategies:
– **Identify Automation Opportunities**: Find customer interaction points that can benefit from AI.
– **Define KPIs**: Establish measurable goals for your AI initiatives.
– **Select an AI Solution**: Choose tools that fit your needs and allow for customization.
– **Implement Gradually**: Start small, gather data, and expand wisely.
For further assistance on AI KPI management, contact us at hello@itinai.com. Stay updated on the latest AI insights by following our channels on Telegram and Twitter. Discover how AI can transform your business at itinai.com.