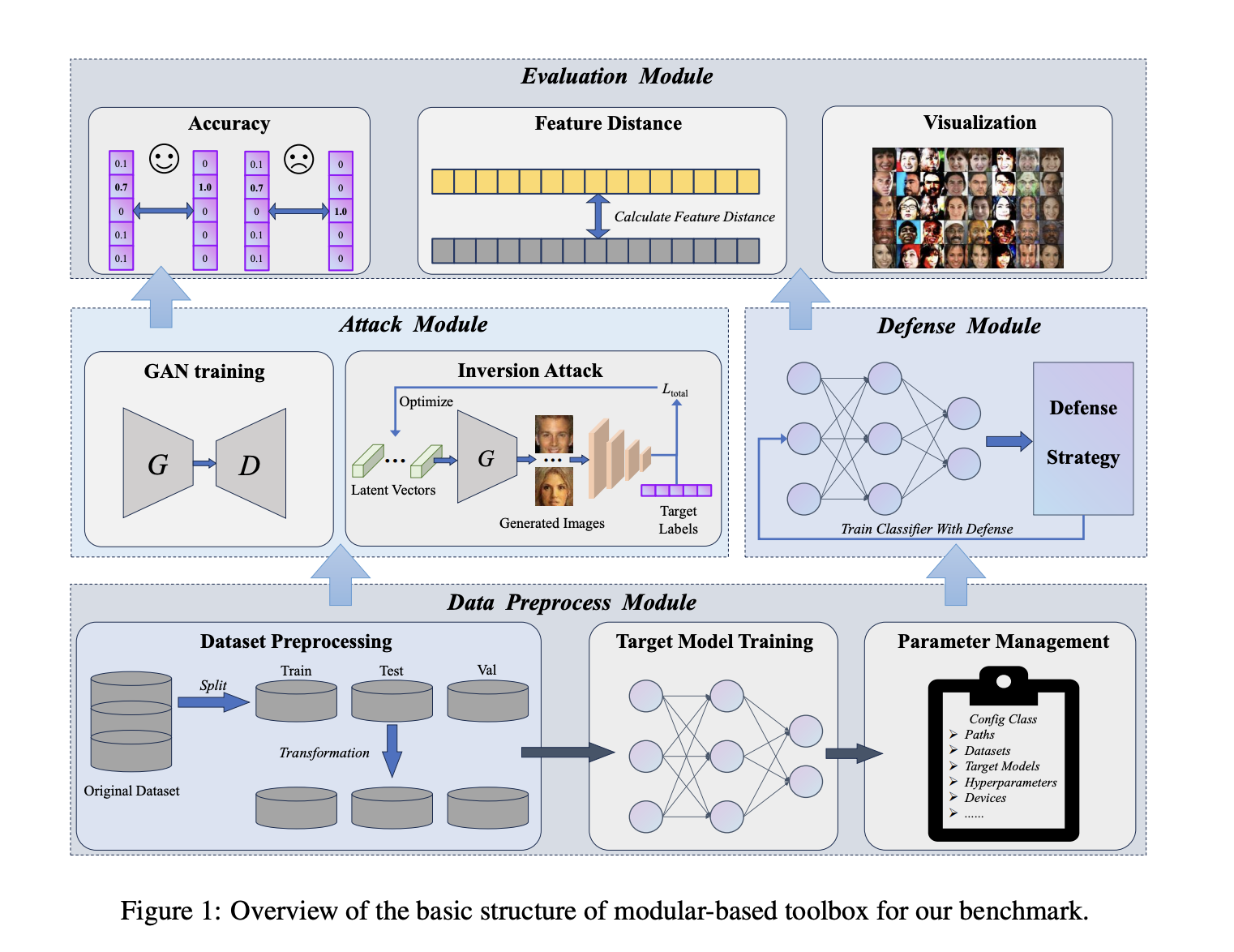
Understanding Model Inversion Attacks
Model Inversion (MI) attacks are privacy threats targeting machine learning models. Attackers aim to reverse-engineer the model’s outputs to reveal sensitive training data, including private images, health information, financial details, and personal preferences. This raises significant privacy concerns for Deep Neural Networks (DNNs).
The Challenge
As MI attacks grow more sophisticated, there is no reliable way to test and compare them, making it hard to evaluate model security. This lack of standardized protocols leads to inconsistent results and inadequate comparisons between different attack methods.
Current Defense Strategies
Defending against MI attacks generally falls into two categories:
- Model Output Processing: Techniques that reduce private information in model outputs. For example, using autoencoders to purify outputs or applying adversarial noise to confuse attackers.
- Robust Model Training: Incorporating defense measures during training to minimize information leakage. This includes penalizing mutual information between inputs and outputs.
Introducing MIBench
To address these issues, researchers from the UniHarbin Institute of Technology and Tsinghua University developed MIBench, the first benchmark for evaluating MI attacks and defenses. This toolbox breaks down the MI process into four modules:
- Data Preprocessing
- Attack Methods
- Defense Strategies
- Evaluation
MIBench includes 16 methods: 11 for attacks and 4 for defenses, along with 9 evaluation protocols focused on Generative Adversarial Network (GAN)-based attacks. It categorizes MI attacks into white-box (full model knowledge) and black-box (limited access) methods.
Testing and Results
The researchers tested MI strategies on two models using various datasets, measuring parameters like accuracy and feature distance. Strong methods like PLGMI showed high accuracy, while others produced realistic images, particularly at higher resolutions. Notably, the effectiveness of MI attacks increased with the model’s predictive capability, indicating a need for improved defenses that maintain model accuracy.
Future Implications
The MIBench benchmark will advance research in the MI field, providing a unified toolbox for rigorous testing and fair evaluations. However, there is a risk that malicious users could exploit these attack methods. To mitigate this, data users must implement strong defense strategies and access controls.
Get Involved
For further insights and updates, follow us on Twitter, join our Telegram Channel, and connect on LinkedIn. If you appreciate our work, consider subscribing to our newsletter and joining our 50k+ ML SubReddit.
AI Solutions for Your Business
To stay competitive and leverage AI effectively, consider the following steps:
- Identify Automation Opportunities: Find areas in customer interactions that can benefit from AI.
- Define KPIs: Ensure measurable impacts on business outcomes from your AI initiatives.
- Select an AI Solution: Choose tools that fit your needs and allow for customization.
- Implement Gradually: Start small, gather data, and expand your AI usage wisely.
For AI KPI management advice, contact us at hello@itinai.com. Stay updated on leveraging AI by following us on Telegram or Twitter.