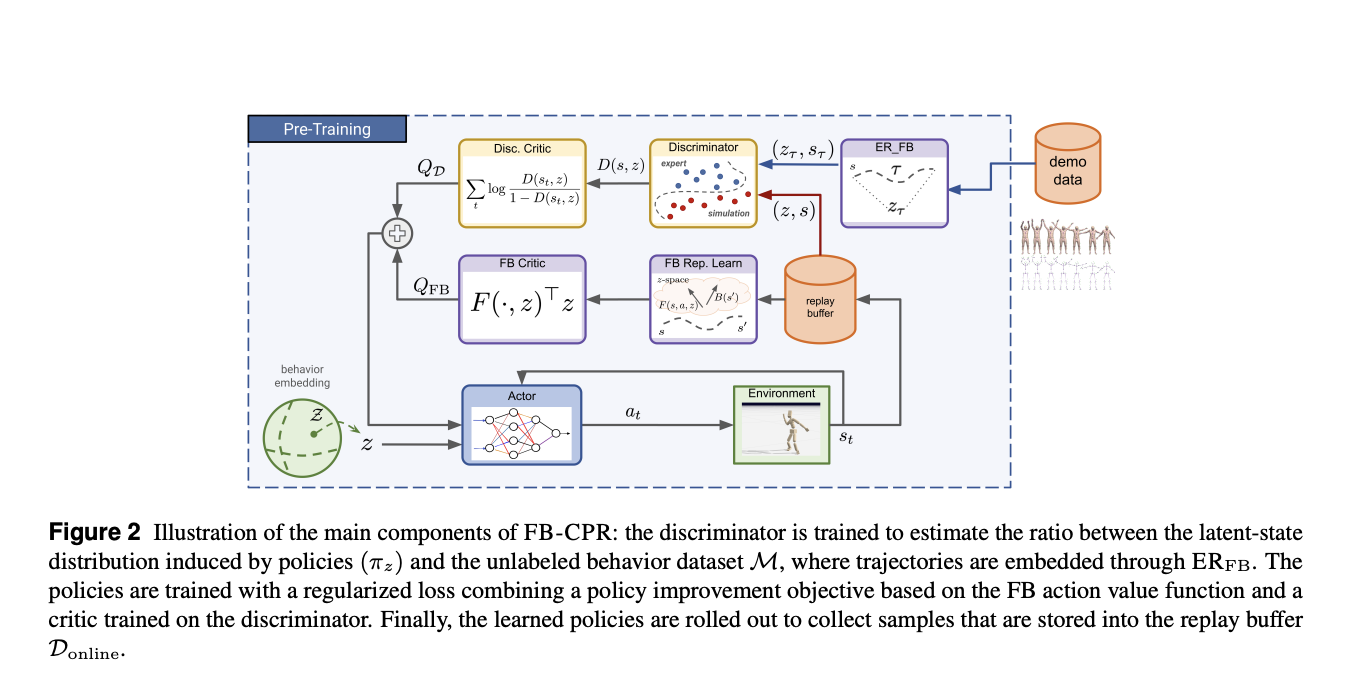
Introduction to Foundation Models
Foundation models are advanced AI systems trained on large amounts of unlabeled data. They can perform complex tasks by responding to specific prompts. Researchers are now looking to expand these models beyond just language and visuals to include Behavioral Foundation Models (BFMs) for agents that interact with changing environments.
Focus on Humanoid Agents
The goal is to create BFMs for humanoid robots that can control their entire body using sensory feedback. This approach tackles the challenges of controlling humanoid robots, which often face issues due to their complex structures and instabilities. The aim is to build models that can adapt their behavior based on different prompts, such as imitating actions, achieving goals, or optimizing rewards.
Introducing FB-CPR
Meta researchers have developed FB-CPR (Forward-Backward representations with Conditional Policy Regularization), a new online learning algorithm. This algorithm allows policy learning based solely on observations of behaviors without needing labeled data. Its main innovation is using forward-backward representations to map behaviors into a shared space, helping policies to cover all states in the dataset.
Performance of META MOTIVO
The FB-CPR algorithm has been used to create META MOTIVO, a BFM for humanoid control. This model can perform various tasks like motion tracking and goal achievement without prior specific training. It uses a combination of the SMPL skeleton and the AMASS motion capture dataset to display a wide range of behaviors.
Key Innovations in Learning
The research emphasizes a strong approach to learning representations by using conditional policy regularization. During pre-training, agents learn from an unlabeled dataset of behaviors. The goal is to create a set of policies that can adapt based on different conditions, capturing various motion patterns effectively.
Impressive Results
The FB-CPR algorithm has shown excellent performance across various tasks. It achieved 73.4% of the best algorithm’s performance without needing specific training. In reward-maximization tasks, it outperformed other unsupervised methods, achieving 177% of DIFFUSER’s performance while being less computationally intensive. In goal-reaching tasks, it surpassed zero-shot alternatives significantly.
Future Directions
While the FB-CPR method has achieved remarkable results, it has limitations. It struggles with tasks that are very different from motion-capture data and can produce imperfect movements in some scenarios. Future research will focus on incorporating more variables, improving perception methods, and enhancing the model’s ability to understand and interact with its environment.
Get Involved
Check out the Paper and GitHub Page for more details. Follow us on Twitter, join our Telegram Channel, and connect with our LinkedIn Group. Don’t forget to join our community of over 60k+ ML enthusiasts on Reddit.
Transform Your Business with AI
Stay competitive by leveraging AI solutions like META MOTIVO. Here’s how you can implement AI effectively:
- Identify Automation Opportunities: Find areas in customer interactions that can benefit from AI.
- Define KPIs: Ensure your AI initiatives have measurable impacts on your business.
- Select an AI Solution: Choose tools that meet your specific needs and allow for customization.
- Implement Gradually: Start with a pilot project, gather data, and expand AI usage carefully.
For AI KPI management advice, connect with us at hello@itinai.com. Stay updated on AI insights via our Telegram or Twitter.
Enhance Your Sales and Customer Engagement
Discover more about redefining your sales processes and customer engagement at itinai.com.