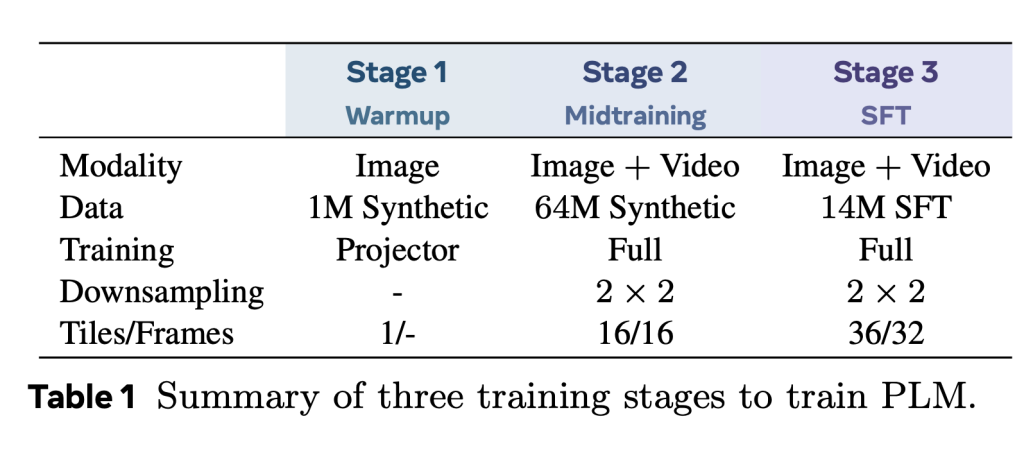
Meta AI’s Perception Language Model: A Business Perspective
Introduction to the Perception Language Model (PLM)
Meta AI has recently launched the Perception Language Model (PLM), an innovative and open framework for vision-language modeling. This model aims to overcome the challenges posed by proprietary datasets that hinder transparency and reproducibility in scientific research, particularly in complex visual recognition tasks.
Addressing Industry Challenges
Limitations of Proprietary Models
Many existing models rely on closed-source systems, which can lead to biased benchmark performances that do not accurately reflect true advancements in research. This creates significant barriers for businesses and researchers who seek to understand and leverage these technologies effectively.
Open and Reproducible Framework
The PLM is designed to be fully open, utilizing large-scale synthetic data and human-labeled datasets. This approach allows for a transparent evaluation of model behavior and training dynamics, which is essential for businesses looking to adopt AI solutions.
Key Features of the PLM
Modular Architecture
The PLM integrates a vision encoder with LLaMA 3 language decoders, supporting various input types, including images and videos. Its multi-stage training pipeline ensures stability and scalability, allowing businesses to implement AI solutions with confidence.
Large-Scale Datasets
Meta AI has released two significant datasets: PLM–FGQA, which includes 2.4 million question-answer pairs focused on human actions, and PLM–STC, with 476,000 spatio-temporal captions. These datasets are crucial for businesses aiming to enhance their video analysis capabilities.
Practical Business Solutions
Implementing AI in Business
- Identify Automation Opportunities: Look for processes that can be automated to improve efficiency.
- Enhance Customer Interactions: Use AI to add value during key customer interactions.
- Measure Impact: Establish important KPIs to assess the effectiveness of AI investments.
- Select the Right Tools: Choose customizable tools that align with your business objectives.
- Start Small: Initiate with a pilot project, gather data, and gradually expand AI applications.
Case Studies and Performance Metrics
Empirical evaluations of the PLM show that it performs competitively across over 40 benchmarks. For instance, in video captioning tasks, the PLM achieved an average improvement of +39.8 CIDEr over existing models. Such performance metrics highlight the potential of PLM to drive significant advancements in various business applications.
Conclusion
The Perception Language Model from Meta AI represents a significant step forward in the field of vision-language modeling. By providing an open and reproducible framework, it empowers businesses to explore AI solutions with transparency and confidence. The availability of high-quality datasets and robust performance metrics positions PLM as a vital resource for organizations aiming to enhance their visual reasoning capabilities in an increasingly data-driven world.