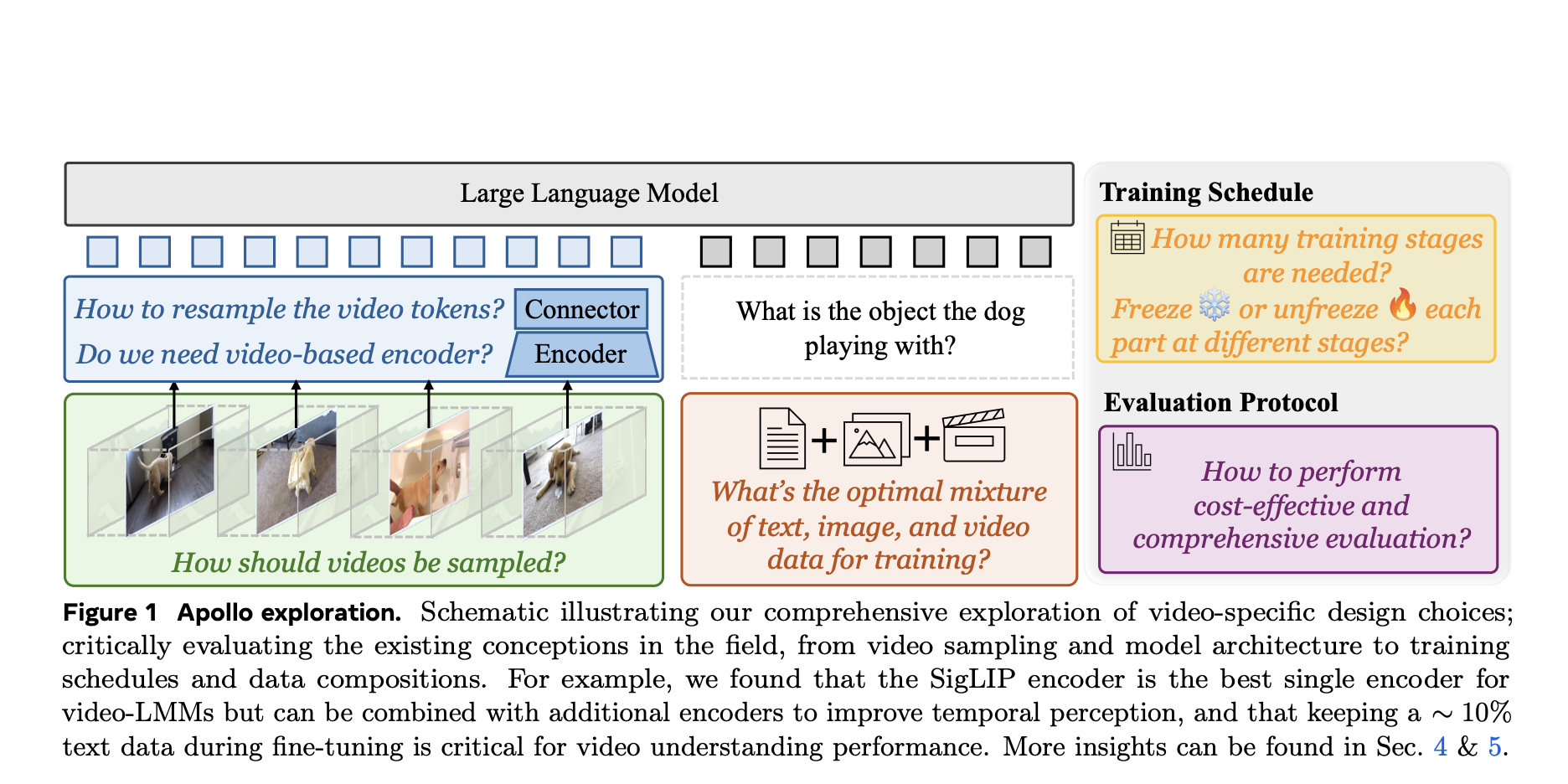
Introduction to Apollo: Advanced Video Models by Meta AI
Despite great progress in multimodal models for text and images, models for analyzing videos lag behind. Videos are complex due to their spatial and temporal elements, requiring significant computational resources. Current methods often use simple image techniques or uniformly sample frames, which do not effectively capture motion or timing. Furthermore, developing large video models is costly and limits efficient exploration of design options.
Apollo’s Innovative Approach
To address these challenges, researchers from Meta AI and Stanford created Apollo, a series of multimodal models focused on video understanding. Apollo sets new standards for tasks like temporal reasoning and answering questions related to videos.
Key Features of Apollo
- Video Length Capability: Apollo can handle videos up to an hour long while excelling in core video-language tasks.
- Model Sizes: Available in 1.5B, 3B, and 7B parameters, Apollo caters to various computational needs.
Innovative Techniques
Apollo employs several groundbreaking techniques:
- Consistent Scaling: Insights gained from smaller models apply well to larger versions, minimizing the need for extensive testing.
- Efficient Frame Sampling: Fox sampling maintains temporal accuracy, improving motion analysis and event sequencing.
- Dual Vision Encoders: Combining SigLIP for spatial info and InternVideo2 for temporal analysis creates superior video representations.
- ApolloBench: A streamlined benchmark that enhances evaluation efficiency and delivers detailed model performance insights.
Performance Benefits of Apollo
- Enhanced Motion Understanding: Apollo’s fps sampling captures events better than standard methods.
- Cost-Effective Scaling: Design choices from mid-sized models apply to larger ones, reducing costs without sacrificing quality.
- Information Retention: Token resampling preserves crucial data while cutting down on processing needs.
- Optimized Training Process: A structured training method ensures effective learning through gradual integration of various datasets.
- Interactive Capabilities: Apollo can facilitate multi-turn conversations based on video content, ideal for chat systems or analysis applications.
Apollo Performance Metrics
Apollo demonstrates impressive performance across multiple benchmarks:
- Apollo-1.5B: Outperforms models like Phi-3.5-Vision and LongVA-7B with scores of 60.8 on Video-MME and 63.3 on MLVU.
- Apollo-3B: Competes with various 7B models, scoring 58.4 on Video-MME and 68.7 on MLVU.
- Apollo-7B: Matches or exceeds performance of models over 30B parameters, scoring 61.2 on Video-MME and 70.9 on MLVU.
Conclusion: Value of Apollo
Apollo represents a significant advancement in video understanding models. By addressing key challenges like efficient sampling and scalability, Apollo offers practical, high-performance solutions for various real-world applications, from question answering to content analysis.
For further insights on leveraging AI, connect with us or check out our resources: Paper, Website, Demo, Code, and Models. Join our communities on Twitter, Telegram, or LinkedIn.
Maximize Your AI Potential
Transform your business with AI solutions designed to enhance competitive edge:
- Identify Opportunities: Find key areas for AI implementation in customer interactions.
- Set KPIs: Ensure your AI projects lead to measurable improvements.
- Select Suitable Tools: Choose customizable AI solutions to match your needs.
- Gradual Implementation: Start small, analyze data, then expand your AI usage.
For guidance on AI KPI management, contact us at hello@itinai.com. Stay updated on leveraging AI via our Telegram or Twitter channels.