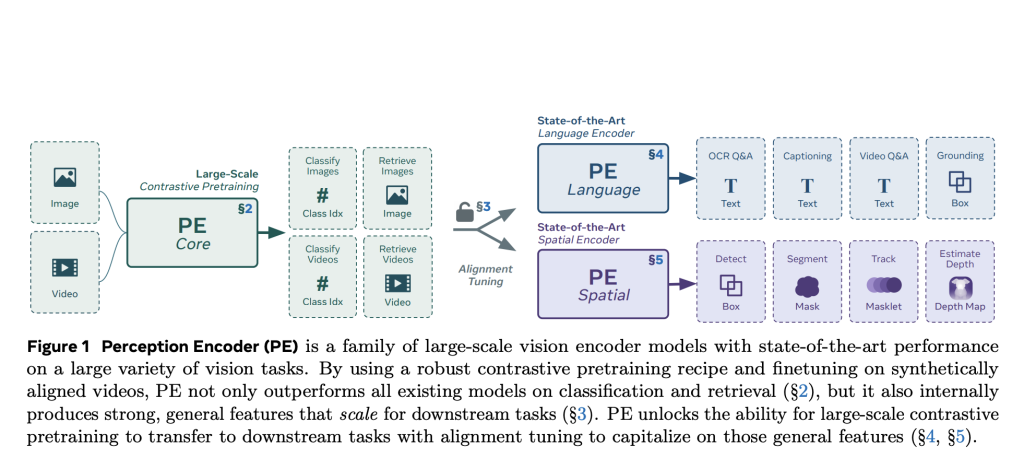
Meta AI’s Perception Encoder: A Business Perspective
The Challenge of General-Purpose Vision Encoders
As artificial intelligence (AI) systems evolve, the demand for sophisticated visual perception models has increased. These models are not only required to identify objects and scenes but also to perform various tasks such as captioning, answering questions, and spatial reasoning across images and videos. Traditional models often depend on multiple pretraining objectives, which can hinder scalability and complicate deployment.
A Unified Solution: The Perception Encoder
Meta AI has introduced the Perception Encoder (PE), a vision model designed to streamline the training process. Unlike conventional models that use multiple objectives, PE employs a single contrastive vision-language objective, enhanced with specific alignment techniques for various tasks. This innovative approach allows PE to deliver highly generalizable visual representations.
Model Variants
The Perception Encoder consists of three variants: PEcoreB, PEcoreL, and PEcoreG, with the largest model containing 2 billion parameters. These models are engineered to serve as versatile encoders for both image and video inputs, excelling in classification, retrieval, and multimodal reasoning.
Training Methodology
PE’s training occurs in two stages:
- Stage One: Robust contrastive learning on a large dataset of 5.4 billion image-text pairs, incorporating advanced techniques to enhance accuracy and robustness.
- Stage Two: Video understanding is integrated through a video data engine that creates high-quality video-text pairs, allowing the model to adapt for video tasks effectively.
Empirical Performance Across Modalities
The Perception Encoder has demonstrated impressive performance across various benchmarks:
- Image Classification: Achieved 86.6% on ImageNet-val and 92.6% on ImageNet-Adversarial.
- Fine-Grained Datasets: Competitive results on iNaturalist, Food101, and Oxford Flowers.
- Video Tasks: State-of-the-art results in zero-shot classification and retrieval, outperforming other models with significantly fewer training data.
Practical Business Solutions
1. Identify Automation Opportunities
Examine your current processes to find areas where AI can enhance efficiency. For instance, automating customer interactions can free up resources for more strategic tasks.
2. Establish Key Performance Indicators (KPIs)
Determine essential KPIs to measure the effectiveness of your AI investments. This will help ensure that your initiatives yield positive business outcomes.
3. Choose the Right Tools
Select AI tools that align with your business needs and allow for customization to meet your specific objectives.
4. Start Small and Scale
Begin with a pilot project to gather data on AI’s effectiveness. Use the insights gained to gradually expand your AI applications across the organization.
Conclusion
The Perception Encoder exemplifies how a single, well-implemented contrastive objective can create powerful general-purpose vision encoders. By adopting this unified and scalable approach, businesses can enhance their visual understanding capabilities. The release of PE, along with its accompanying resources, provides a solid foundation for developing advanced multimodal AI systems. As the complexity of visual reasoning tasks increases, PE offers a promising pathway for achieving integrated and robust visual comprehension.