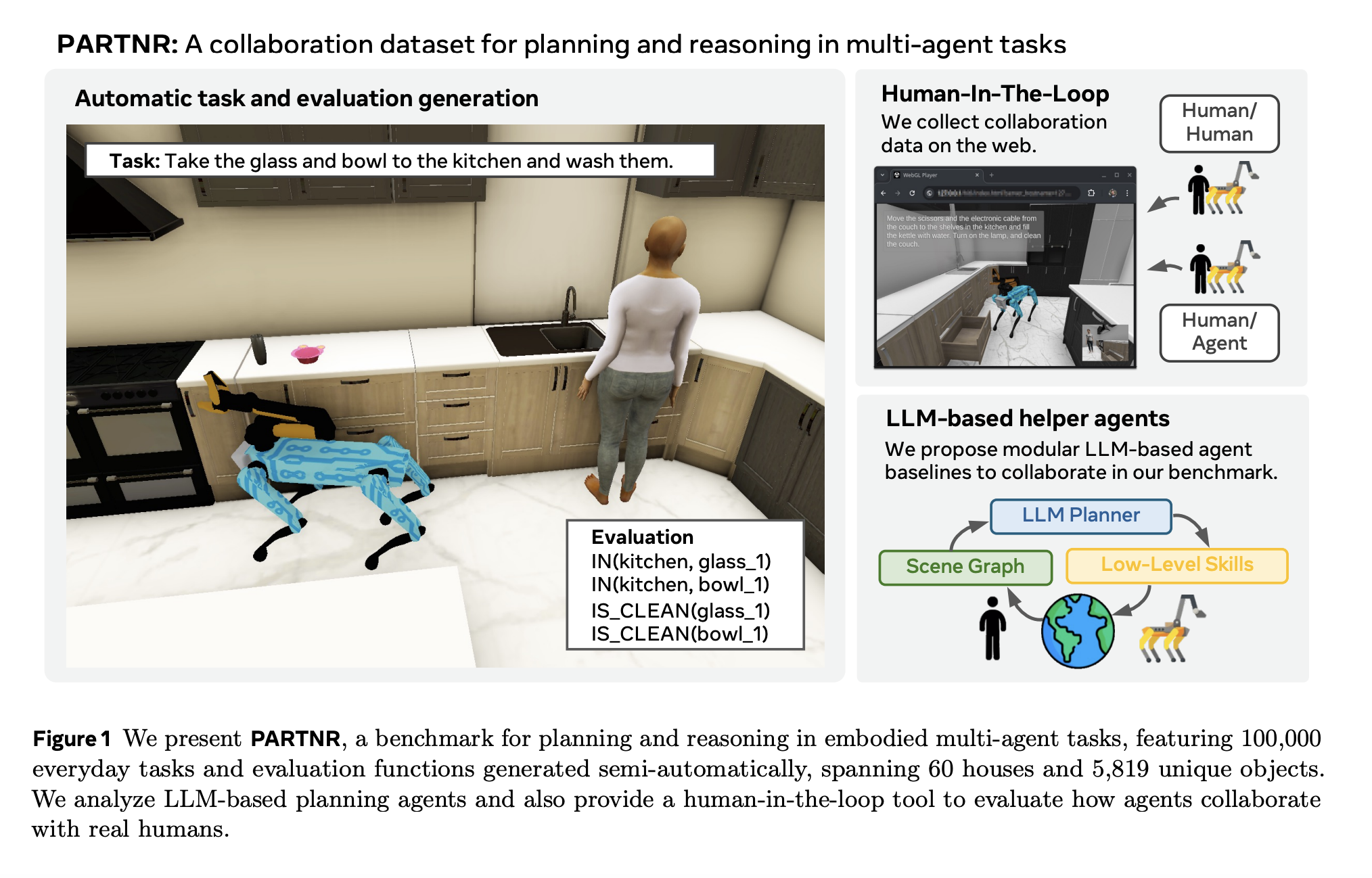
Understanding Human-Robot Collaboration
Human-robot collaboration is about creating smart systems that work with people in changing environments. The goal is to develop robots that can understand everyday language and adapt to various tasks, such as household chores, healthcare, and industrial automation. This collaboration is essential for improving efficiency and making robots more useful in our daily lives.
Challenges in Collaboration
A key challenge is the absence of a standard way to evaluate how well robots can plan and reason in teamwork situations. Many existing models focus on simple tasks and do not address the complexities of real-world interactions. This makes it hard to measure and enhance the performance of collaborative AI systems.
Current Limitations
Most current AI solutions focus on single tasks, ignoring the need for teamwork. Some rely on fixed instructions, limiting their flexibility, while others use manual methods that are not practical for large-scale evaluations. Even advanced language models struggle with tracking tasks and recovering from mistakes, which is crucial in environments where robots work closely with humans.
Introducing PARTNR
Researchers at FAIR Meta have developed PARTNR (Planning And Reasoning Tasks in humaN-Robot collaboration), a benchmark to evaluate how well robots can work with humans in simulated settings. PARTNR includes:
- 100,000 natural language tasks
- 60 simulated homes
- 5,819 unique objects
This benchmark assesses tasks with various constraints, ensuring a realistic evaluation of AI capabilities.
Task Categories
The tasks in PARTNR are divided into four categories:
- Constraint-free: Flexible execution order
- Spatial: Requires specific object placement
- Temporal: Needs tasks to be done in a specific order
- Heterogeneous: Involves actions needing human help
Findings from Evaluations
Evaluations showed that current AI models face significant challenges in coordination and task execution. For example, when working with humans, AI-guided robots needed more steps to complete tasks compared to human teams. The success rate of these models was only 30% in real-world conditions, compared to 93% for humans. Smaller models, when fine-tuned, performed comparably to larger models but were faster and more efficient.
The Value of PARTNR
PARTNR reveals important gaps in current AI models for human-robot collaboration, highlighting the need for better planning and decision-making strategies. This benchmark serves as a foundation for improving AI’s ability to work alongside humans effectively. Future research can focus on enhancing AI planners and coordination methods.
Get Involved
To learn more about this research, check out the Paper. Follow us on Twitter, join our Telegram Channel, and connect with us on LinkedIn. Join our community of over 75k on our ML SubReddit.
Transform Your Business with AI
To stay competitive, consider how AI can enhance your operations:
- Identify Automation Opportunities: Find areas where AI can improve customer interactions.
- Define KPIs: Ensure your AI initiatives have measurable impacts.
- Select an AI Solution: Choose tools that fit your needs and allow customization.
- Implement Gradually: Start with a pilot project, gather data, and expand wisely.
For AI KPI management advice, reach out to us at hello@itinai.com. For ongoing insights, follow us on Telegram or @itinaicom.
Discover how AI can enhance your sales and customer engagement at itinai.com.