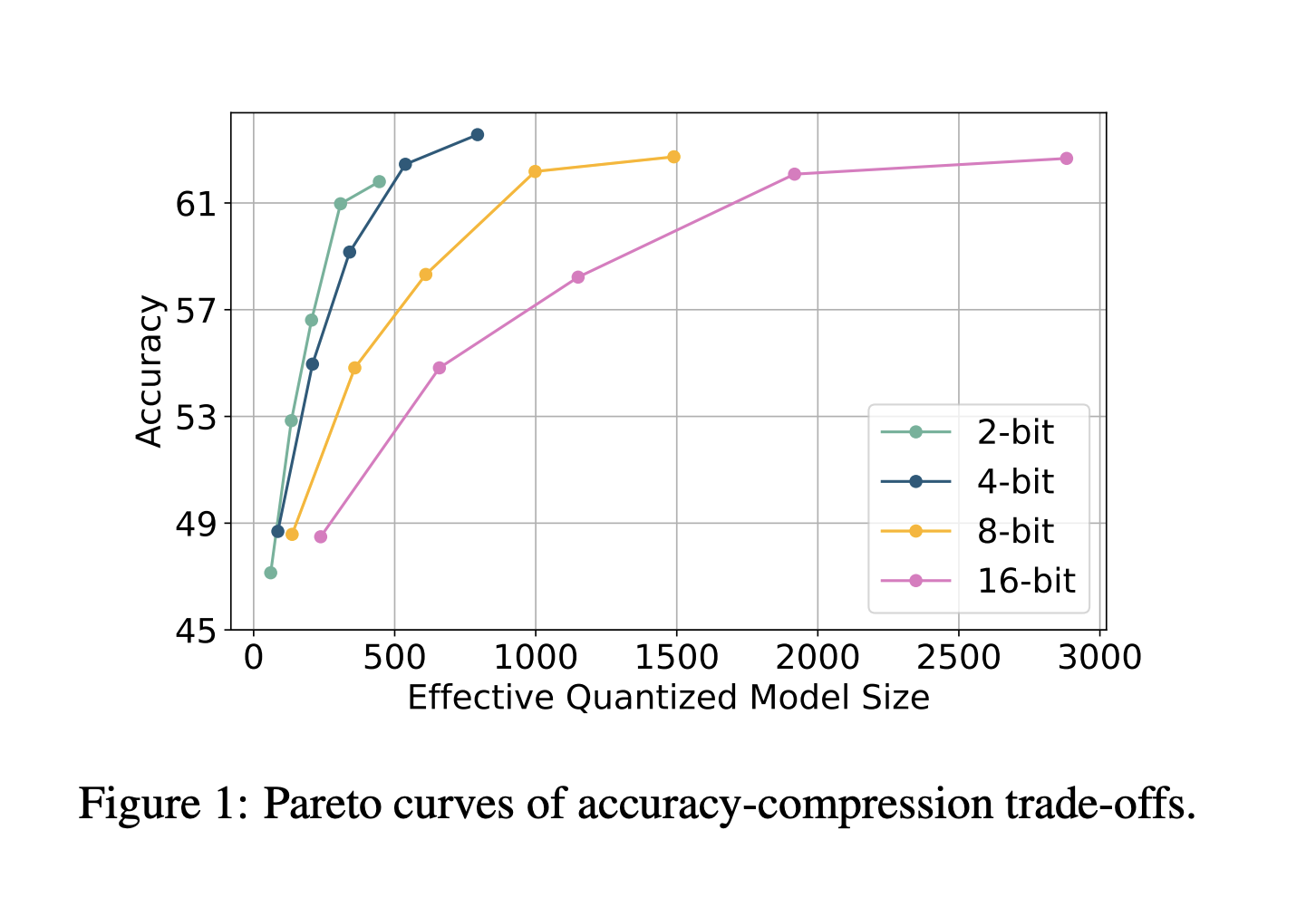
Understanding Low-Bit Quantization in AI
Why Quantization Matters
As deep learning models evolve, it’s crucial to compress them effectively. Low-bit quantization reduces model size while aiming to keep accuracy intact. Researchers are exploring the best bit-width settings to maximize efficiency without sacrificing performance.
The Challenge of Bit-Width Selection
Finding the right balance between computational efficiency and model accuracy is challenging. There’s ongoing debate about the most effective bit-width, with some suggesting 4-bit quantization and others advocating for 1.58-bit models. The lack of a standardized evaluation framework has led to inconsistent findings, complicating the establishment of reliable scaling laws.
Different Quantization Techniques
Quantization methods vary in effectiveness. Post-training quantization (PTQ) is easy to deploy but may lose accuracy at low bit-widths. In contrast, quantization-aware training (QAT) incorporates quantization during training, helping models adapt better. Other strategies, like learnable quantization and mixed-precision approaches, also exist but lack a universal evaluation framework.
Introducing ParetoQ
Researchers at Meta have developed ParetoQ, a structured framework for assessing sub-4-bit quantization techniques. This framework allows for rigorous comparisons across various bit-widths, improving accuracy and efficiency. Unlike previous methods, ParetoQ offers a consistent evaluation process for quantization trade-offs.
Optimized Training Strategies
ParetoQ uses an optimized quantization-aware training strategy to minimize accuracy loss while ensuring model compression. It identifies key differences in learning between 2-bit and 3-bit quantization, optimizing training allocation and bit-specific strategies.
Proven Performance
Extensive experiments show ParetoQ outperforms existing methods. A ternary 600M-parameter model developed with ParetoQ surpasses a previous 3B-parameter model in accuracy while using significantly fewer parameters. Notably, 2-bit quantization shows a 1.8 percentage point accuracy improvement over a comparable 4-bit model.
Future of Low-Bit Quantization
The findings support optimizing low-bit quantization in large language models. The structured framework addresses accuracy trade-offs and bit-width optimization challenges. While extreme low-bit quantization is possible, 2-bit and 3-bit quantization currently provide the best performance and efficiency balance.
Explore More
For more insights, check out the research paper. Follow us on Twitter, join our Telegram Channel, and connect with our LinkedIn Group. Join our 75k+ ML SubReddit community for ongoing discussions.
Transform Your Business with AI
Stay competitive by leveraging AI solutions like ParetoQ. Here’s how to get started:
– **Identify Automation Opportunities:** Find key customer interaction points for AI benefits.
– **Define KPIs:** Ensure measurable impacts on business outcomes.
– **Select an AI Solution:** Choose customizable tools that fit your needs.
– **Implement Gradually:** Start with a pilot, gather data, and expand wisely.
For AI KPI management advice, contact us at hello@itinai.com. Stay updated on AI insights via our Telegram or Twitter. Explore how AI can enhance your sales processes and customer engagement at itinai.com.