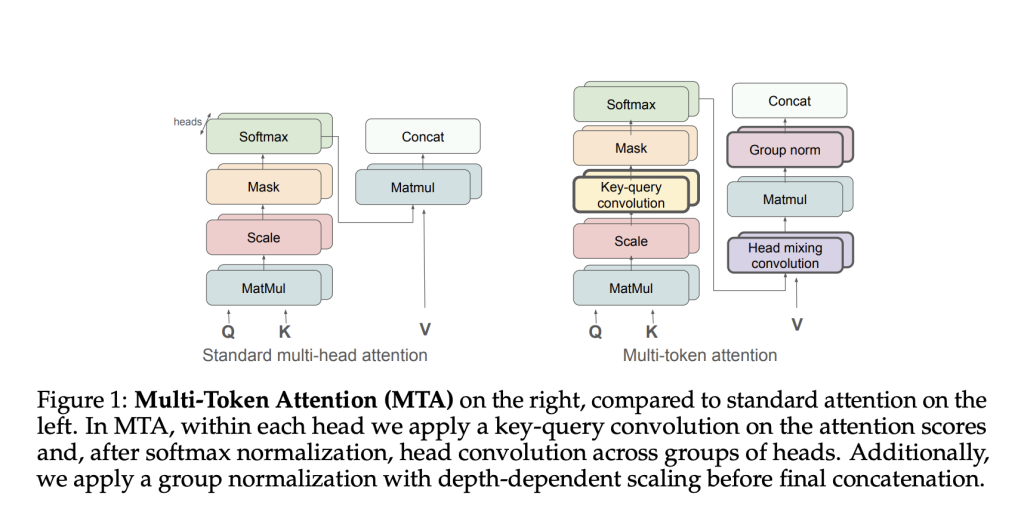
Meta AI’s Multi-Token Attention: Revolutionizing Language Models
Introduction to Attention Mechanisms in Language Models
Large Language Models (LLMs) rely heavily on attention mechanisms to efficiently retrieve contextual information. However, traditional attention methods are limited to single-token attention, which focuses on individual pairs of query and key vectors. This constraint can hinder the model’s ability to understand complex linguistic dependencies, such as sentences that contain multiple relevant tokens. Addressing this challenge is critical for improving the effectiveness of LLMs in understanding nuanced language.
The Innovation: Multi-Token Attention (MTA)
Meta AI has introduced a groundbreaking approach known as Multi-Token Attention (MTA), which allows LLMs to condition their attention weights on multiple query and key vectors simultaneously. This enhancement addresses the limitations of conventional attention mechanisms by integrating convolution operations across queries, keys, and attention heads, thus improving both the precision and efficiency of contextual information retrieval.
Key Features of MTA
- Key-Query Convolution: This component aggregates multiple token signals within individual attention heads, facilitating better context understanding.
- Head Mixing Convolution: This feature promotes information sharing among different attention heads, enhancing the model’s ability to capture relevant signals.
- Group Normalization: Implemented with depth-dependent scaling, this technique stabilizes gradient flow, contributing to improved training stability and efficiency.
Technical Overview
MTA modifies traditional attention calculations by applying a two-dimensional convolution operation on attention logits before softmax normalization. This allows adjacent queries and keys to mutually influence attention scores, enabling the model to capture intricate contextual relationships more accurately. Consequently, MTA efficiently aggregates local token interactions without significantly increasing model complexity.
Empirical Evidence of MTA’s Effectiveness
Empirical evaluations affirm the superiority of MTA across multiple benchmarks. In a structured task designed to highlight the weaknesses of single-token attention, MTA achieved an impressive error rate of just 0.1%, compared to over 50% for standard Transformer models. Additionally, in large-scale experiments with an 880M-parameter model trained on 105 billion tokens, MTA consistently outperformed baseline models, achieving better validation perplexity scores across diverse datasets like arXiv, GitHub, and Wikipedia.
Case Study: Performance on Complex Tasks
In tasks requiring extensive context comprehension, such as the Needle-in-the-Haystack and BabiLong benchmarks, MTA demonstrated remarkable performance. Specifically, in the Needle-in-the-Haystack task with 4,000-token contexts, MTA achieved accuracies between 67% and 97.6%, significantly outperforming standard models.
Conclusion
Multi-Token Attention (MTA) represents a significant advancement in attention mechanisms, effectively addressing the limitations of traditional single-token attention. By employing convolutional operations to integrate multiple query-key interactions, MTA enhances language models’ capabilities in handling complex contextual dependencies. These methodological improvements lead to more precise and efficient performance, especially in scenarios involving intricate token interactions and long-range contextual understanding. As businesses increasingly adopt AI technologies, MTA stands as a pivotal development toward creating more sophisticated, accurate, and computationally efficient language models.
Next Steps for Businesses
To leverage these advancements in your organization, consider the following steps:
- Identify processes that can be automated to maximize efficiency.
- Determine key performance indicators (KPIs) to assess the impact of AI investments.
- Select tools that align with your business objectives and allow customization.
- Start with small pilot projects, gather data, and gradually expand your use of AI.
Contact Us for AI Solutions
If you require guidance on integrating AI into your business practices, please reach out to us at hello@itinai.ru. Connect with us on Telegram, X, and LinkedIn.