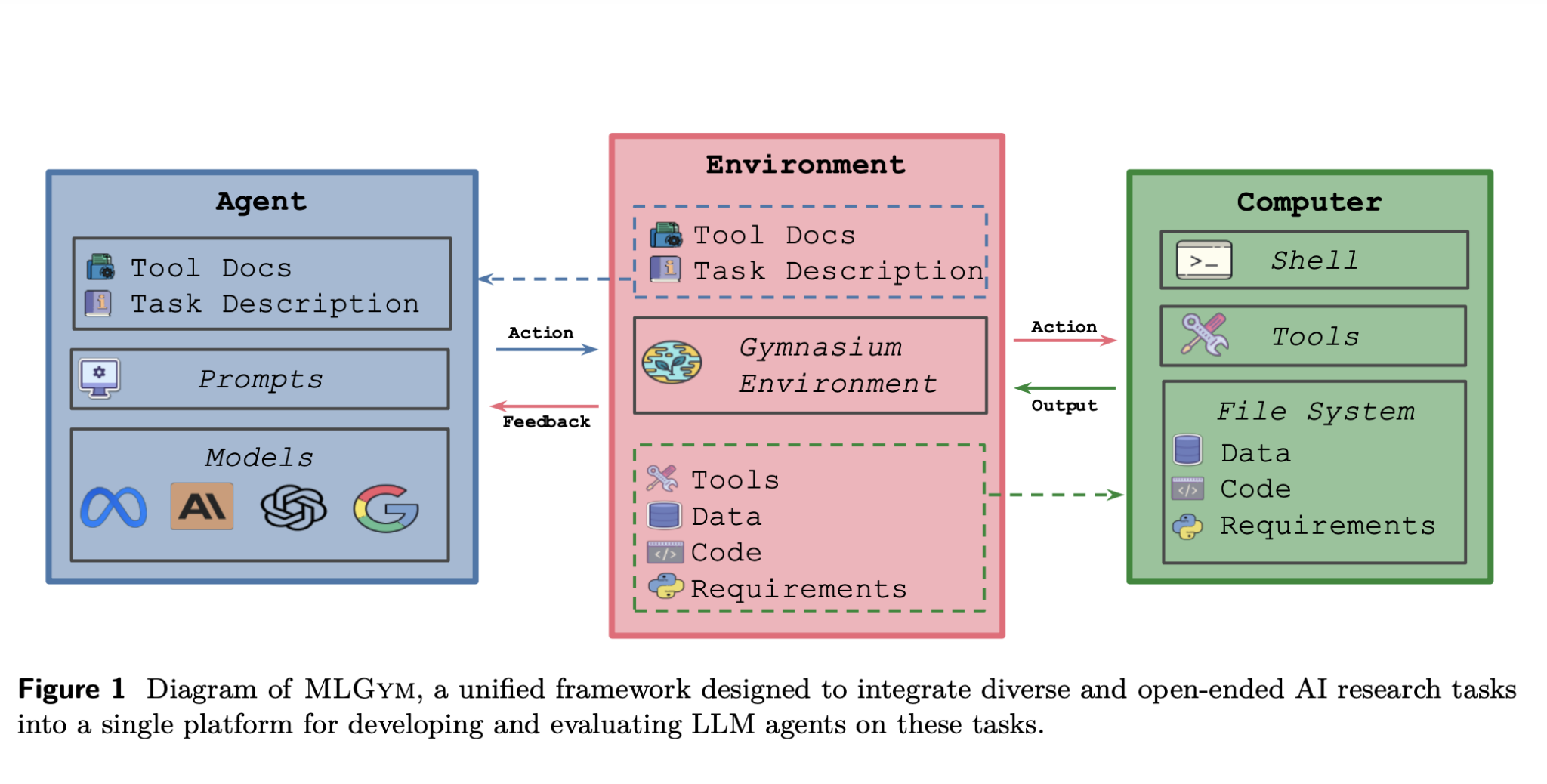
The ambition to enhance scientific discovery through artificial intelligence (AI) has been a long-standing goal, with notable initiatives like the Oak Ridge Applied AI Project starting as far back as 1979. Recent advancements in foundation models now allow for fully automated research processes, enabling AI systems to independently conduct literature reviews, develop hypotheses, design experiments, analyze results, and even draft scientific papers. These AI systems can also optimize scientific workflows by automating repetitive tasks, freeing researchers to concentrate on more complex conceptual work. However, assessing the effectiveness of AI-driven research poses challenges due to the absence of standardized benchmarks that can thoroughly evaluate their capabilities across various scientific fields.
Recent studies have aimed to fill this gap by creating benchmarks that assess AI agents on different software engineering and machine learning tasks. While there are frameworks for evaluating AI agents on specific problems, such as code generation and model optimization, most current benchmarks do not adequately support open-ended research challenges, where multiple solutions may arise. Additionally, these frameworks often lack the flexibility to evaluate diverse research outputs, including new algorithms, model architectures, or predictions. To enhance AI-driven research, we need evaluation systems that encompass a broader range of scientific tasks, allow experimentation with different learning algorithms, and accommodate various research contributions. Developing such comprehensive frameworks will help us move towards AI systems that can independently foster meaningful scientific advancements.
Researchers from University College London, University of Wisconsin–Madison, University of Oxford, Meta, and other institutions have created a new framework and benchmark for evaluating and developing large language model (LLM) agents in AI research. This system, known as MLGym, is the first Gym environment for machine learning tasks and supports the study of reinforcement learning techniques for training AI agents. The benchmark, MLGym-Bench, includes 13 open-ended tasks covering areas like computer vision, natural language processing, reinforcement learning, and game theory, which require real-world research skills. It employs a six-level framework to categorize AI research agent capabilities, with MLGym-Bench emphasizing Level 1: Baseline Improvement, where LLMs enhance models but do not contribute to scientific knowledge.
MLGym is structured to evaluate and develop LLM agents for machine learning research tasks, allowing interaction with a shell environment through sequential commands. It consists of four main components: Agents, Environment, Datasets, and Tasks. Agents execute bash commands, manage their history, and integrate external models. The environment offers a secure Docker-based workspace with regulated access. Datasets are specified separately from tasks, promoting reuse across experiments. Tasks include evaluation scripts and configurations for various machine learning challenges. Furthermore, MLGym provides tools for literature searches, memory storage, and iterative validation, ensuring efficient experimentation and adaptability in long-term AI research workflows.
The study utilizes a SWE-Agent model tailored for the MLGYM environment, employing a ReAct-style decision-making process. Five advanced models—OpenAI O1-preview, Gemini 1.5 Pro, Claude-3.5-Sonnet, Llama-3-405b-Instruct, and GPT-4o—are evaluated under standardized conditions. Performance is measured using AUP scores and performance profiles, comparing models based on Best Attempt and Best Submission metrics. OpenAI O1-preview demonstrates the highest overall performance, with Gemini 1.5 Pro and Claude-3.5-Sonnet closely following. The study showcases performance profiles as an effective evaluation method, indicating that OpenAI O1-preview consistently ranks among the top models across various tasks.
In conclusion, the study underscores the potential and challenges of utilizing LLMs as scientific workflow agents. MLGym and MLGymBench exhibit adaptability across various quantitative tasks but also highlight areas for improvement. Key growth opportunities lie in expanding beyond machine learning, testing interdisciplinary generalization, and evaluating scientific novelty. The study emphasizes the significance of data openness to foster collaboration and discovery. As AI research evolves, advancements in reasoning, agent architectures, and evaluation methods will be essential. Strengthening interdisciplinary collaboration will help ensure that AI-driven agents accelerate scientific discovery while upholding reproducibility, verifiability, and integrity.
To explore how artificial intelligence technology can enhance your business operations, consider the following steps:
- Examine processes for automation and identify customer interactions where AI can provide significant value.
- Establish key performance indicators (KPIs) to ensure your AI investments yield positive business impacts.
- Choose tools that align with your needs and allow for customization to achieve your objectives.
- Start with a small project, assess its effectiveness, and gradually expand your AI applications.
If you require guidance on managing AI in your business, please contact us at hello@itinai.ru. Connect with us on Telegram, X, and LinkedIn.