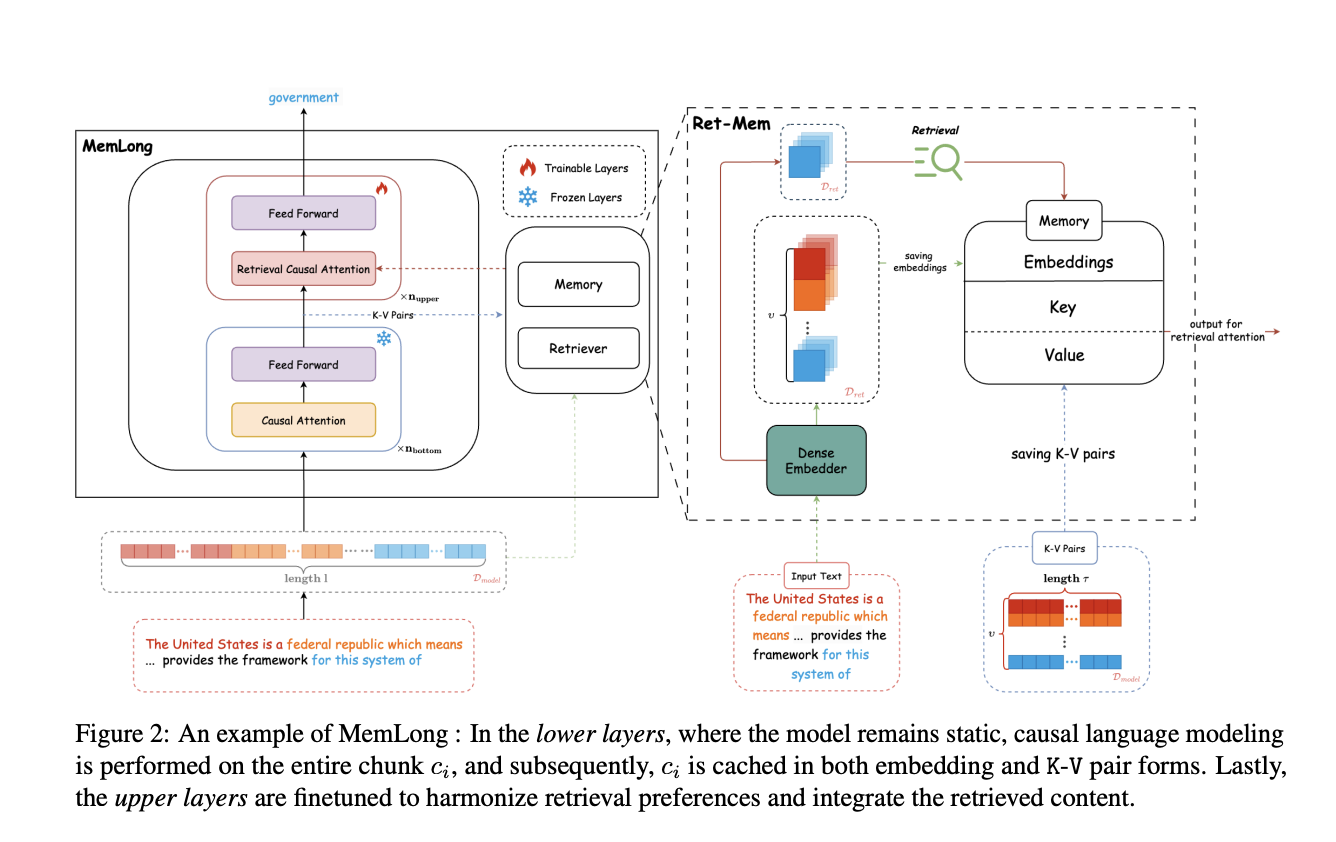
MemLong: Revolutionizing Long-Context Language Modeling with Memory-Augmented Retrieval
The paper “MemLong: Memory-Augmented Retrieval for Long Text Modeling” introduces MemLong, a solution addressing the challenge of processing long contexts in Large Language Models (LLMs). By integrating an external retrieval mechanism, MemLong significantly extends the context length that LLMs can handle, enhancing their applicability in tasks such as long-document summarization and multi-turn dialogue.
Practical Solutions and Value
MemLong utilizes a non-differentiable retrieval-memory module combined with a partially trainable decoder-only language model to efficiently retrieve historical information during text generation. It extends the context length from 4,000 to 80,000 tokens on a single GPU, outperforming existing LLMs in managing long contexts without sacrificing original capabilities. The dynamic memory management system intelligently updates stored information based on retrieval frequency, prioritizing relevant data and discarding outdated information. This approach enhances overall performance in long-text processing, offering a robust framework for future developments in retrieval-augmented applications.
AI Solutions for Business Evolution
Identify Automation Opportunities: Locate key customer interaction points that can benefit from AI. Define KPIs: Ensure AI endeavors have measurable impacts on business outcomes. Select an AI Solution: Choose tools aligning with needs and providing customization. Implement Gradually: Start with a pilot, gather data, and expand AI usage judiciously.