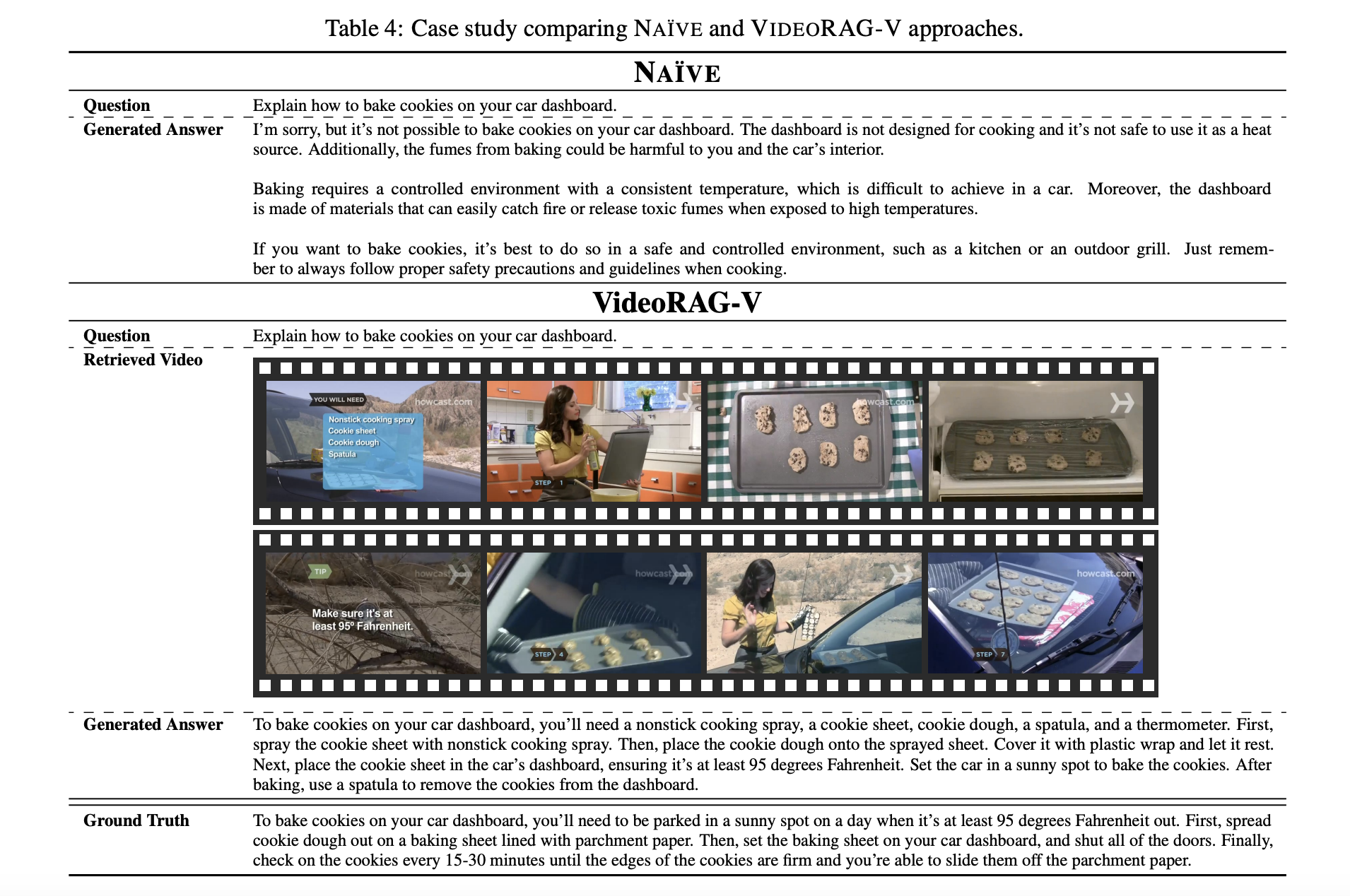
Video-Based Technologies: A New Era for Information Retrieval
Video-based technologies are essential for understanding complex concepts. They provide a rich combination of visual and contextual data, making them more effective than static images or text. With many educational videos online, using these resources allows us to answer questions that need detailed context and spatial understanding.
Challenges with Current Systems
Most retrieval-augmented generation (RAG) systems focus on text and static images, missing out on the full potential of video data. Traditional methods either limit video analysis to predefined clips or convert videos into text, losing vital visual information. This makes it hard to provide accurate answers for complex queries.
Introducing VideoRAG: A Game-Changer
Research teams have developed VideoRAG, a new framework that effectively uses video data in RAG systems. It dynamically retrieves videos relevant to user queries and integrates both visual and textual information for better responses. By utilizing advanced Large Video Language Models (LVLMs), VideoRAG ensures that retrieved videos are contextually relevant and maintain the richness of video content.
How VideoRAG Works
The VideoRAG framework consists of two main stages: retrieval and generation.
- During retrieval, it identifies videos based on their visual and textual similarities to the query.
- It uses automatic speech recognition to generate text for videos that lack subtitles, ensuring meaningful contributions from all videos.
These relevant videos are then processed together with other data, allowing LVLMs to produce comprehensive and accurate responses. This method highlights the importance of combining visual and textual elements, making it easier to explain complex processes.
Proven Results
VideoRAG has been tested on datasets like WikiHowQA and HowTo100M, showing improved response quality. For instance:
- ROUGE-L score: VideoRAG achieved 0.254, compared to 0.228 for traditional text-based methods.
- BLEU-4 score: VideoRAG scored 0.054, while text-based systems scored 0.044.
- Using both video frames and transcripts improved BERTScore to 0.881, surpassing the baseline of 0.870.
Why VideoRAG Matters
VideoRAG’s ability to combine visual and textual elements leads to richer, more precise responses. It excels in scenarios needing detailed spatial and temporal understanding. By addressing the limitations of existing methods, VideoRAG sets a new standard for future multimodal retrieval systems.
Unlock Your Company’s Potential with AI
Discover how AI can transform your business operations. Here are practical steps to get started:
- Identify Automation Opportunities: Find key customer interactions that could benefit from AI.
- Define KPIs: Ensure measurable impacts from your AI initiatives.
- Select an AI Solution: Choose tools that fit your needs and allow for customization.
- Implement Gradually: Start small, gather data, and expand wisely.
For AI KPI management advice, connect with us at hello@itinai.com. For continuous insights, follow us on Telegram or Twitter.
Learn More
Check out the research paper to explore VideoRAG further. Join our 65k+ ML SubReddit for more discussions on AI advancements.
Stay competitive and redefine your work with AI solutions at itinai.com.