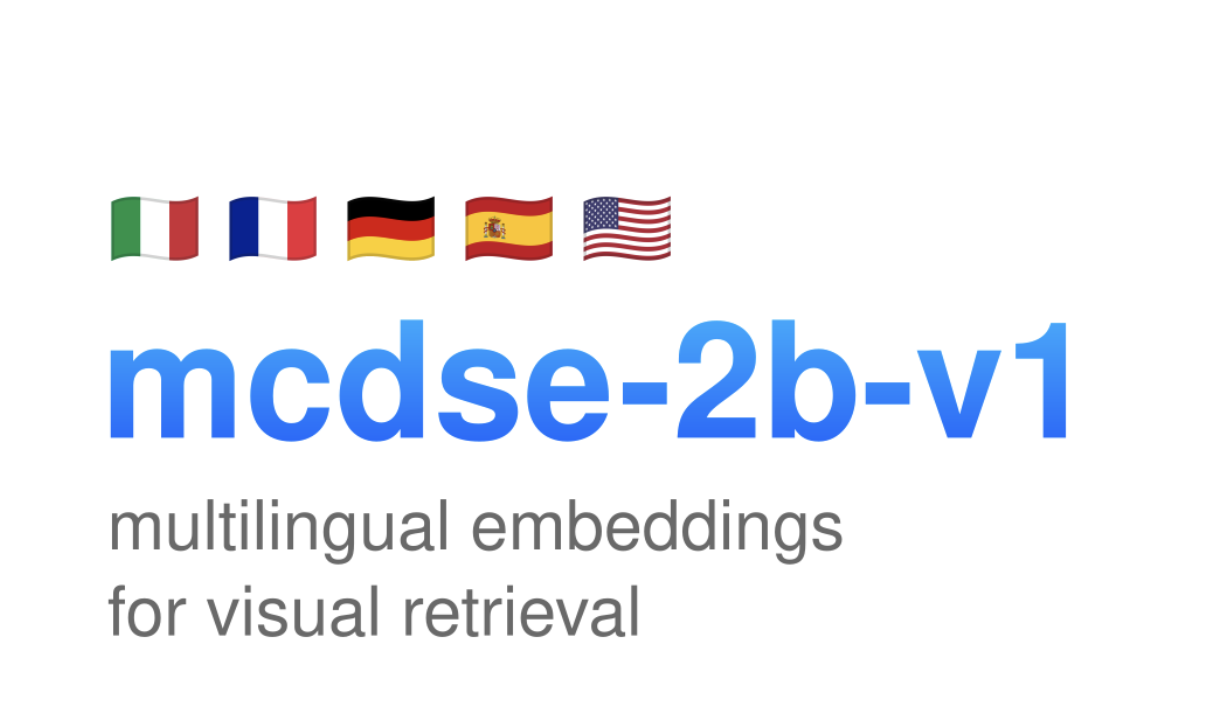
The Challenge of Information Retrieval
Today, we generate a vast amount of data in many formats, like documents and presentations, in different languages. Finding relevant information from these sources can be very difficult, especially when dealing with complex content like screenshots or slide presentations.
Traditional retrieval methods mainly focus on text, which makes it hard to extract information from documents that include both text and visuals. This is a challenge for businesses, researchers, and educators alike who need to search through mixed-format documents.
Introducing mcdse-2b-v1: A Smart Solution for Document Retrieval
Meet mcdse-2b-v1, an innovative AI model that allows you to include screenshots of pages or slides and search for information using everyday language. Unlike traditional systems that only consider text, mcdse-2b-v1 can analyze documents with text, images, and diagrams. This means you can simply take a screenshot of a presentation or an infographic and ask the model to find the information you need.
Time-Saving and Efficient
This model is particularly valuable in industries where analyzing content from visual documents is crucial. Instead of sifting through lengthy reports or searching for specific slides, users can query the model in natural language, making information retrieval faster and enhancing productivity.
Technical Benefits of mcdse-2b-v1
One major advantage of mcdse-2b-v1 is that it efficiently embeds up to 100 million pages in just 10 GB of storage. This is perfect for settings where space is limited, such as on-premises or edge deployments. Additionally, the model can be reduced in size by up to six times without losing much performance, allowing it to run on devices with limited computing power.
Moreover, mcdse-2b-v1 is compatible with popular frameworks like Transformers and vLLM, making it easy for developers and data scientists to integrate into their existing systems with minimal changes.
Why Choose mcdse-2b-v1?
mcdse-2b-v1 democratizes access to complex document analysis. Traditional methods often overlook valuable visual content, but this model allows users to easily find information within diagrams and charts, just like with text searches.
With proven high retrieval accuracy even at reduced sizes, it is ideal for large-scale applications that require efficiency without high computational costs. Its ability to handle multiple languages also serves a diverse range of users globally, making it a great fit for international organizations or academic environments.
Scaling Up with AI
For teams working on multimodal Retrieval-Augmented Generation (RAG), mcdse-2b-v1 provides scalable, high-performance results, improving the accuracy of complex queries and report generation.
Conclusion
mcdse-2b-v1 effectively addresses the challenges of retrieving information from diverse document types. It simplifies the way users interact with complex content, allowing them to find what they need without tedious manual searching. This model significantly enhances how we access and understand knowledge embedded in both text and visuals.
Explore more about mcdse-2b-v1 by checking it out on Hugging Face, and stay updated through our channels. Engage with our AI solutions to improve your company’s operations and stay ahead in your industry.