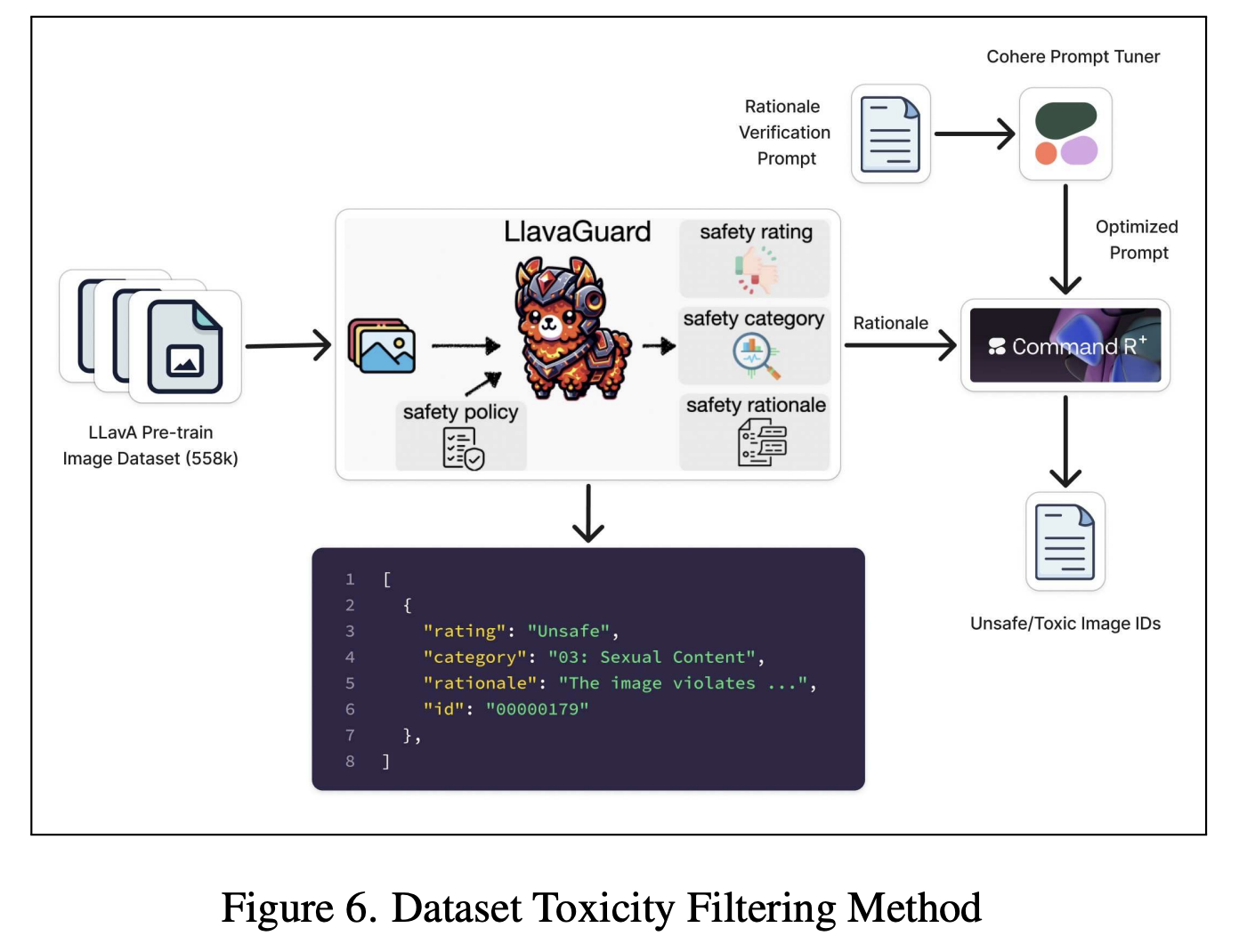
Understanding Vision-Language Models (VLMs)
Vision-Language Models (VLMs) help machines interpret the visual world using natural language. They are useful for tasks like image captioning, answering visual questions, and reasoning across different types of information.
However, many of these models primarily focus on high-resource languages, making them less accessible for speakers of low-resource languages. This creates a need for multilingual systems that can perform well across various languages and cultures.
Challenges in Current Datasets
Despite the existence of datasets, they face several challenges:
- Most datasets, like COCO and Visual Genome, mainly focus on English, limiting their effectiveness in other languages.
- Many datasets contain biased or harmful content, which can reinforce stereotypes and affect the ethical use of AI.
- The lack of representation for diverse languages and cultures can lead to unfair outcomes and hinder performance in underrepresented areas.
Efforts to Improve Datasets
Researchers are working on enhancing dataset quality through various methods:
- Diverse datasets like Multi30k aim to provide multilingual support, but more expansion is needed.
- Techniques like semi-automated translations have been used to broaden language coverage, but often result in imbalanced distributions.
- Addressing toxicity in datasets remains a significant challenge.
Introducing Maya
A collaborative team of researchers has developed Maya, an open-source multilingual model with 8 billion parameters that tackles the issues of dataset quality and toxicity. Key features include:
- A new dataset with 558,000 image-text pairs across eight languages, rigorously filtered for toxicity, removing over 7,531 toxic elements.
- Support for eight languages, ensuring balanced data distribution and cultural inclusivity.
- Advanced architecture that includes SigLIP for image encoding and Aya-23 for multilingual language understanding.
- Performance that exceeds similar models in five languages, demonstrating its effectiveness.
Key Highlights of Maya
- Expanded dataset to 4.4 million samples across eight languages.
- Rigorous toxicity filtering leads to cleaner, more ethical data.
- Outperformed comparable models in multiple benchmarks.
- Sets a new standard for ethical AI practices by addressing biases.
Conclusion
In summary, Maya addresses the gaps in multilingual and culturally sensitive datasets in VLMs. With its innovative dataset and advanced architecture, it ensures inclusivity and ethical deployment while outperforming similar models, paving the way for better multilingual AI solutions.
Get Involved and Learn More
Check out the Paper and Model on Hugging Face. Follow us on Twitter, join our Telegram Channel, and connect with our LinkedIn Group. Don’t forget to join our 60k+ ML SubReddit.
Elevate Your Business with AI
If you want to stay competitive and leverage AI in your company, consider the following steps:
- Identify Automation Opportunities: Find customer interaction points that can benefit from AI.
- Define KPIs: Ensure measurable impacts from your AI initiatives.
- Select an AI Solution: Pick tools that meet your needs and allow for customization.
- Implement Gradually: Start with a pilot project, gather data, and expand usage carefully.
For advice on AI KPI management, reach out to us at hello@itinai.com. For ongoing insights, stay connected on Telegram or Twitter.
Transform Your Sales Processes with AI
Explore innovative solutions at itinai.com.