Summary: Financial language presents challenges for existing NLP models due to its complexity and real-time demands. Recent advancements in financial NLP include specialized models like FinTral, a multimodal LLM tailored for the financial sector. FinTral’s versatility, real-time adaptability, and advanced capabilities show promise for improving predictive accuracy and decision-making in financial analysis.
(Word count: 50)
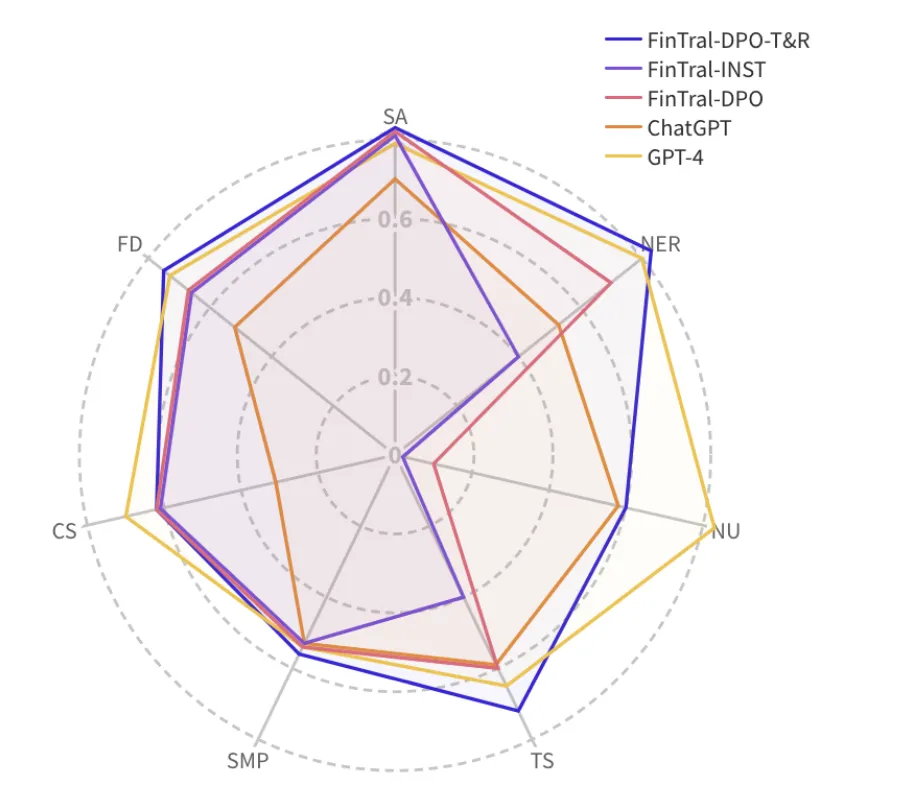
“`html
Financial Documents and AI Solutions
Financial documents are often challenging to process due to complex numerical data and specialized jargon. Natural Language Processing (NLP) models need advanced capabilities for numerical processing and a deep understanding of financial language, especially in the fast-paced financial markets.
Recent Advancements
Recent advancements in financial NLP have led to the development of specialized models like FinBERT, BloombergGPT, PIXIU, Instruct-FinGPT, and GPT-FinRE. These models address challenges such as sentiment analysis, event extraction, and investment strategy enhancement. The innovations have also extended to multimodal capabilities with models like FinVis-GPT and rigorous model evaluation frameworks like FinLMEval and DISCFinLLM.
Introducing FinTral
FinTral, a groundbreaking Large Language Model (LLM) from the University of British Columbia & Invertible AI, utilizes a multimodal approach to process textual, numerical, tabular, and visual data in financial documents. It introduces FinSet, a comprehensive benchmark for evaluating financial LLMs, and has shown remarkable capabilities in outperforming established models like GPT-4 in numerous tasks.
Key Features of FinTral
FinTral leverages textual, numerical, tabular, and visual data for enriched financial document analysis. It undergoes domain-specific pretraining on the expansive FinSet dataset and benefits from instruction tuning and AI-driven feedback to enhance performance. With visual data processing through CLIP encoders and tools for numerical tasks, FinTral can effectively tackle the complexities of financial analysis with unprecedented accuracy and depth.
Performance and Future Developments
Experimental results demonstrate FinTral’s exceptional performance, surpassing many contemporary models across various financial tasks. While there are opportunities for improvement, including real-time data handling and scarcity of annotated data, FinTral shows promising potential for real-time adaptability to financial markets and dynamic data retrieval.
Evolve Your Company with AI
If you want to evolve your company with AI and stay competitive, consider utilizing FinTral to analyze complex financial data and improve predictive accuracy and decision-making. AI can redefine your way of work by automating customer interaction points, providing measurable impacts on business outcomes, and offering tailored AI solutions to enhance your sales processes and customer engagement.
“`