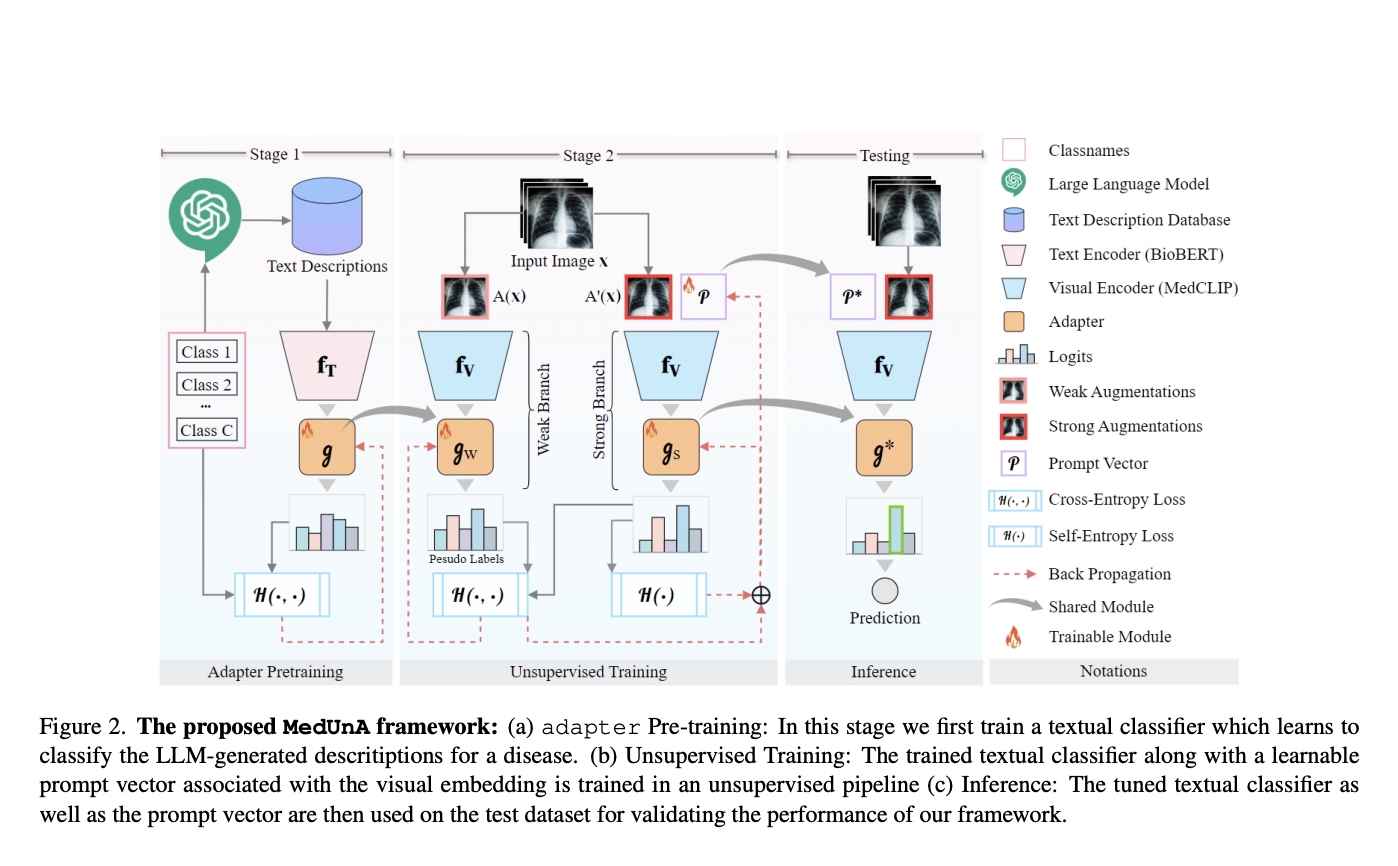
Practical Solutions for Medical Image Classification
Addressing Labeled Data Scarcity
Utilize Vision-Language Models (VLMs) for unsupervised learning and reduced reliance on labeled data.
Lowering Annotation Costs
Pre-train VLMs on large medical image-text datasets to generate accurate labels and captions, reducing annotation expenses.
Enhancing Data Diversity and Model Performance
VLMs generate synthetic images and annotations, improving model performance in medical imaging tasks.
Efficient Adaptation for Medical Tasks
MedUnA method efficiently adapts vision-language models for medical tasks, reducing reliance on labeled data and enhancing scalability.
Improved Efficiency and Performance
MedUnA offers improved efficiency and performance without extensive pre-training, leveraging the alignment between visual and textual embeddings.
Experimental Results and Performance Analysis
MedUnA achieved superior accuracy compared to baseline models, demonstrating notable improvements on several medical datasets.
AI Solution Implementation
Identify automation opportunities, define KPIs, select an AI solution, and implement gradually to evolve your company with AI.
Connect with AI Experts
For AI KPI management advice and continuous insights into leveraging AI, connect with us at hello@itinai.com or stay tuned on our Telegram t.me/itinainews or Twitter @itinaicom.