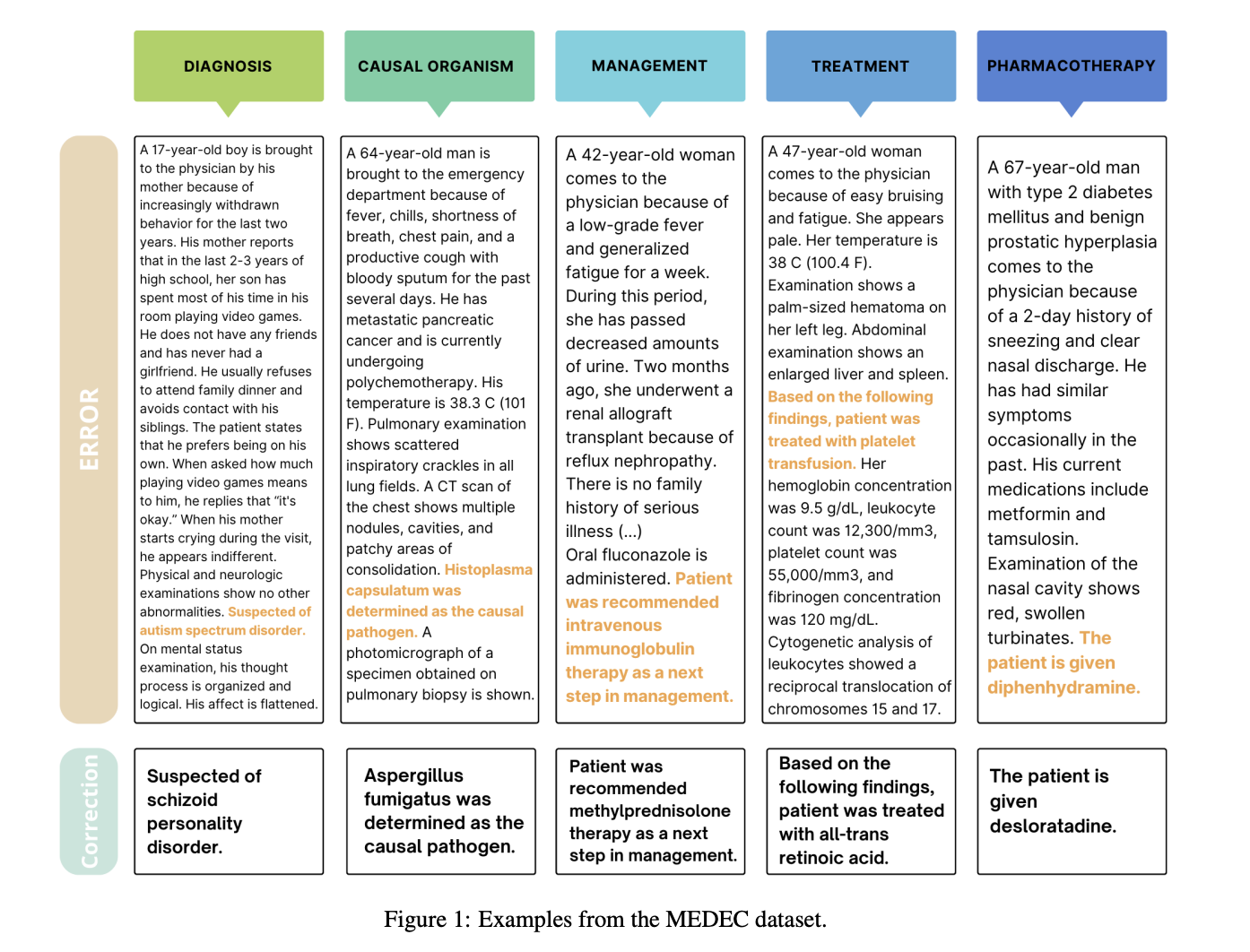
Understanding the Challenges and Solutions of LLMs in Medical Documentation
Impressive Capabilities but Significant Risks
Large Language Models (LLMs) can answer medical questions accurately and even outperform average humans in some medical exams. However, using them for tasks like clinical note generation poses risks, as they may produce incorrect or inconsistent information. Studies show that 20% of patients found errors in their clinical notes, with 40% considering these errors serious, often linked to misdiagnoses. This raises concerns about the reliability of LLMs in medical documentation.
The Need for Validation Frameworks
Although LLMs like ChatGPT and GPT-4 perform well in structured medical exams, they can generate misleading content that may harm clinical decision-making. This emphasizes the need for strong validation systems to ensure the accuracy and safety of medical content generated by LLMs.
Introducing MEDEC: A Solution for Medical Error Detection
Researchers from Microsoft and the University of Washington have created MEDEC, the first publicly available benchmark for identifying and correcting medical errors in clinical notes. MEDEC includes 3,848 clinical texts with five types of errors: Diagnosis, Management, Treatment, Pharmacotherapy, and Causal Organism. This benchmark helps evaluate LLMs’ performance in error detection and correction, highlighting the need for models with strong medical reasoning.
How MEDEC Works
MEDEC’s dataset consists of clinical texts with annotated errors, created by modifying real clinical notes. It assesses models on their ability to predict errors, identify erroneous sentences, and generate corrections. Various models, including GPT-4, were tested, revealing that while LLMs perform well, human medical experts still excel in detecting and correcting errors.
Performance Insights and Future Directions
The performance gap between LLMs and medical experts is likely due to limited error-specific data during LLM training. Some models showed high recall rates but struggled with precision, often overestimating errors. This indicates a need for more targeted training and better datasets.
Join the Conversation and Learn More
Check out the Paper and GitHub Page for more details. Follow us on Twitter, join our Telegram Channel, and connect with our LinkedIn Group. Don’t forget to join our 60k+ ML SubReddit.
Webinar Invitation
Join our webinar to gain actionable insights into enhancing LLM performance and ensuring data privacy.
Transform Your Business with AI
To stay competitive, leverage MEDEC for detecting and correcting medical errors in clinical notes. Here’s how AI can transform your work:
- Identify Automation Opportunities: Find key customer interaction points that can benefit from AI.
- Define KPIs: Ensure measurable impacts on business outcomes.
- Select an AI Solution: Choose tools that fit your needs and allow customization.
- Implement Gradually: Start with a pilot project, gather data, and expand wisely.
For AI KPI management advice, connect with us at hello@itinai.com. For ongoing insights into leveraging AI, stay tuned on our Telegram or @itinaicom.
Revolutionize Your Sales and Customer Engagement
Discover how AI can redefine your sales processes and customer engagement at itinai.com.