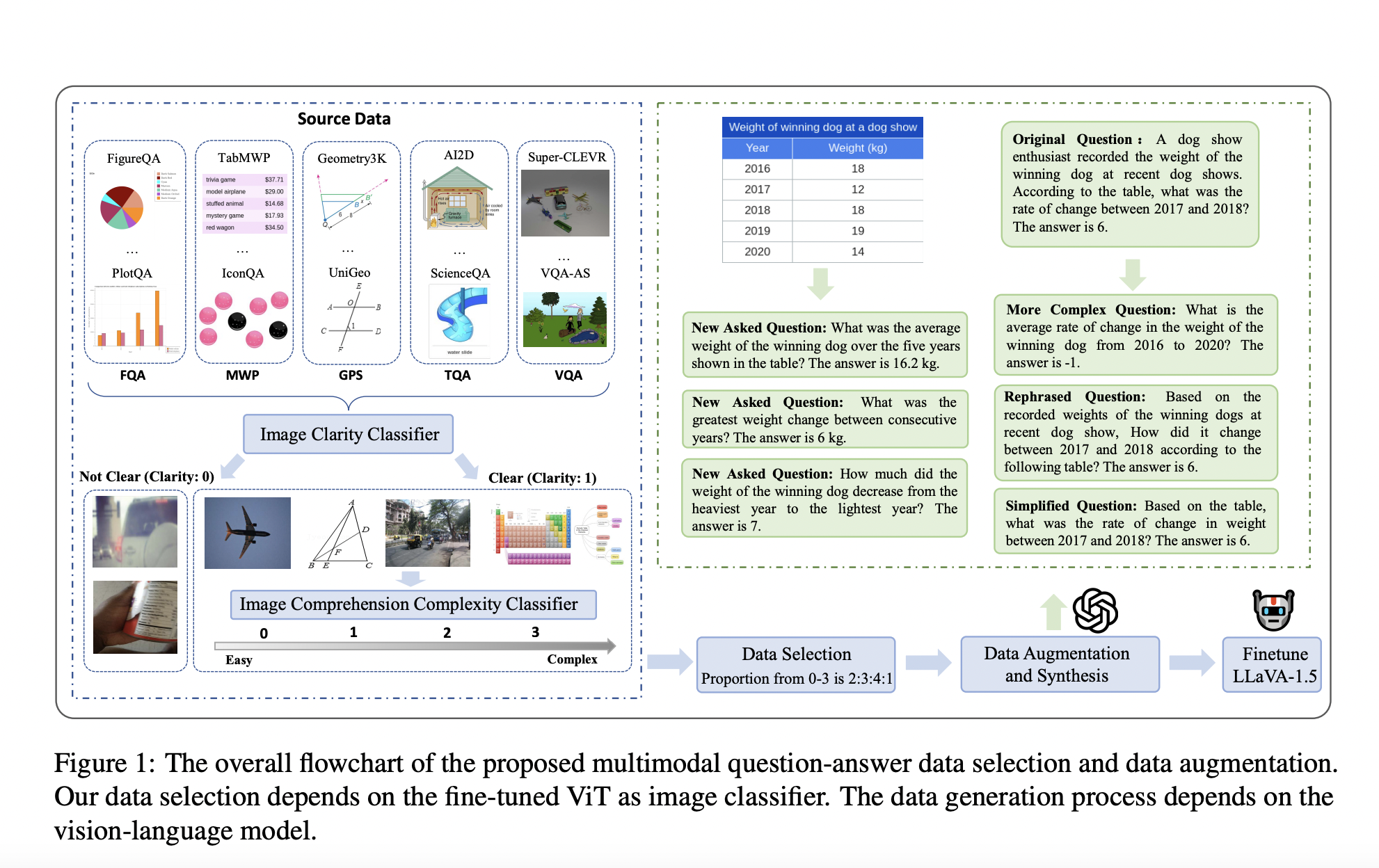
Enhancing Multimodal Mathematical Reasoning with Math-LLaVA
Integrating Visual and Textual Data for Advanced AI Capabilities
Research on Multimodal large language models (MLLMs) focuses on integrating visual and textual data to enhance artificial intelligence’s reasoning capabilities. By combining these modalities, MLLMs can interpret complex information from diverse sources such as images and text, enabling them to perform tasks like visual question answering and mathematical problem-solving with greater accuracy and insight. This interdisciplinary approach leverages the strengths of both visual and linguistic data, aiming to create more robust AI systems capable of understanding and interacting with the world like humans.
Challenges and Solutions in Developing Effective MLLMs
A significant challenge in developing effective MLLMs is their inability to solve complex mathematical problems involving visual content. Despite their proficiency in textual mathematical problem-solving, these models often need to improve when interpreting and reasoning through visual information. This gap highlights the need for improved datasets and methodologies that better integrate multimodal data. Researchers strive to create models that can understand text and derive meaningful insights from images, diagrams, and other visual aids critical in fields like education, science, and technology.
Addressing Limitations and Advancing MLLMs
Existing methods to enhance MLLMs’ mathematical reasoning include prompt and fine-tuning approaches. However, current open-source image instruction datasets are limited in scope, containing few question-answer pairs per image, which restricts the models’ ability to exploit visual information fully. The limitations of these datasets impede the development of MLLMs, necessitating the creation of more comprehensive and diverse datasets to train these models effectively.
Math-LLaVA: A Significant Advancement in Multimodal Mathematical Reasoning
Researchers introduced Math-LLaVA, a model fine-tuned with a novel dataset called MathV360K, aiming to improve the breadth and depth of multimodal mathematical reasoning capabilities. This comprehensive dataset includes 40K high-quality images and 320K synthesized question-answer pairs designed to enhance the diversity and complexity of the dataset. The development of Math-LLaVA represents a significant step forward in the field, addressing the gaps left by previous datasets and methods.
Performance and Generalizability of Math-LLaVA
Math-LLaVA demonstrated significant improvements, achieving a 19-point increase on the MathVista minutest split compared to the original LLaVA-1.5 model. Furthermore, it showed enhanced generalizability and performed well on the MMMU benchmark, highlighting the effectiveness of the diverse and comprehensive MathV360K dataset in enhancing the multimodal mathematical reasoning capabilities of MLLMs.
Implications and Future Prospects
The research underscores the critical need for high-quality, diverse multimodal datasets to improve mathematical reasoning in MLLMs. The MathV360K dataset and the Math-LLaVA model represent a substantial advancement in the field, providing a robust framework for future research and development. This work not only underscores the potential of MLLMs to transform various domains by integrating visual and textual data but also inspires hope for the future of AI, paving the way for more sophisticated and capable AI systems.
Check out the Paper. All credit for this research goes to the researchers of this project. Also, don’t forget to follow us on Twitter.
Join our Telegram Channel and LinkedIn Group.
If you like our work, you will love our newsletter.
Don’t Forget to join our 45k+ ML SubReddit
Evolve Your Company with AI
If you want to evolve your company with AI, stay competitive, use for your advantage Math-LLaVA: A LLaVA-1.5-based AI Model Fine-Tuned with MathV360K Dataset.
AI Integration and Business Transformation
Discover how AI can redefine your way of work. Identify Automation Opportunities: Locate key customer interaction points that can benefit from AI. Define KPIs: Ensure your AI endeavors have measurable impacts on business outcomes. Select an AI Solution: Choose tools that align with your needs and provide customization. Implement Gradually: Start with a pilot, gather data, and expand AI usage judiciously.
For AI KPI management advice, connect with us at hello@itinai.com. And for continuous insights into leveraging AI, stay tuned on our Telegram or Twitter.
AI for Sales Processes and Customer Engagement
Discover how AI can redefine your sales processes and customer engagement. Explore solutions at itinai.com.