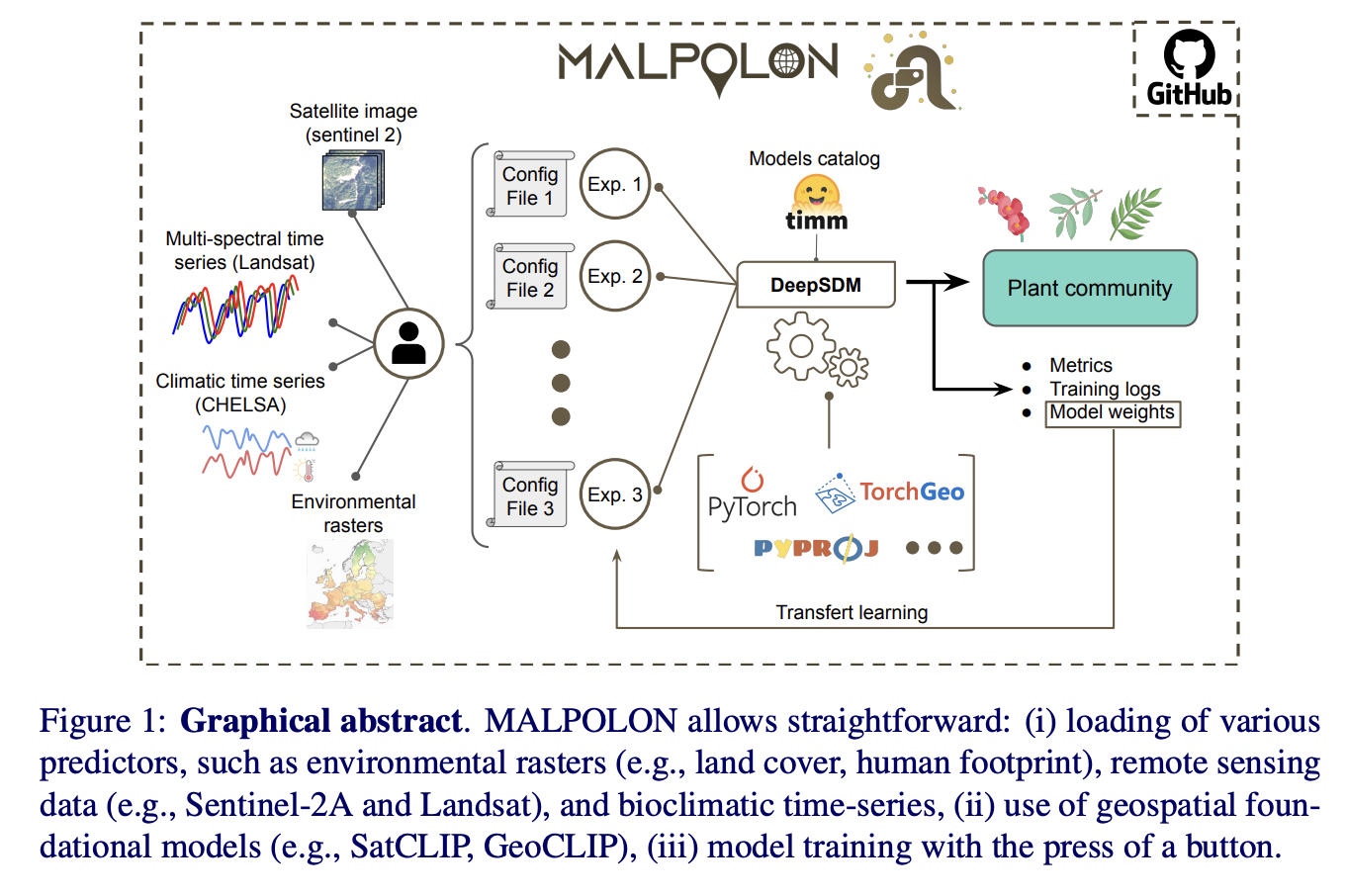
Practical Solutions for Species Distribution Modeling
Evolution of SDM
Species distribution modeling (SDM) is crucial in ecological research for predicting species distributions using environmental data. SDMs have advanced from basic statistical methods to machine-learning approaches for better accuracy.
Challenges with Traditional SDMs
Conventional SDMs face challenges in handling complex datasets efficiently. Existing models require manual intervention, struggle with multi-species predictions, and lack scalability for large datasets.
Integrating Deep Learning for SDMs
Deep learning methods like CNNs and Transformers are promising for capturing complex relationships in SDMs. However, adoption is hindered by accessibility barriers and usability issues in existing frameworks.
Introduction of MALPOLON Framework
MALPOLON is a Python-based deep SDM tool developed by a research team, offering seamless training and inference capabilities. It supports multi-modal data integration and modular customization, enhancing performance and scalability.
Key Advantages of MALPOLON
MALPOLON leverages PyTorch Lightning for distributed training, achieving high efficiency across multiple GPUs. It outperforms traditional methods with a micro-averaged precision of 30.1% and a sample-averaged precision of 29.9%.
Transformation of SDM Practices
MALPOLON simplifies deep learning integration, promotes reproducibility, and is accessible through GitHub and PyPi. Its compatibility with geospatial libraries and modularity allows for easy experimentation and customization, advancing ecological research.
—
If you are looking to enhance your company’s AI capabilities and stay competitive, consider leveraging MALPOLON for advanced species distribution modeling. Connect with us at hello@itinai.com for AI KPI management advice and stay updated on AI insights via our Telegram t.me/itinainews or Twitter @itinaicom.