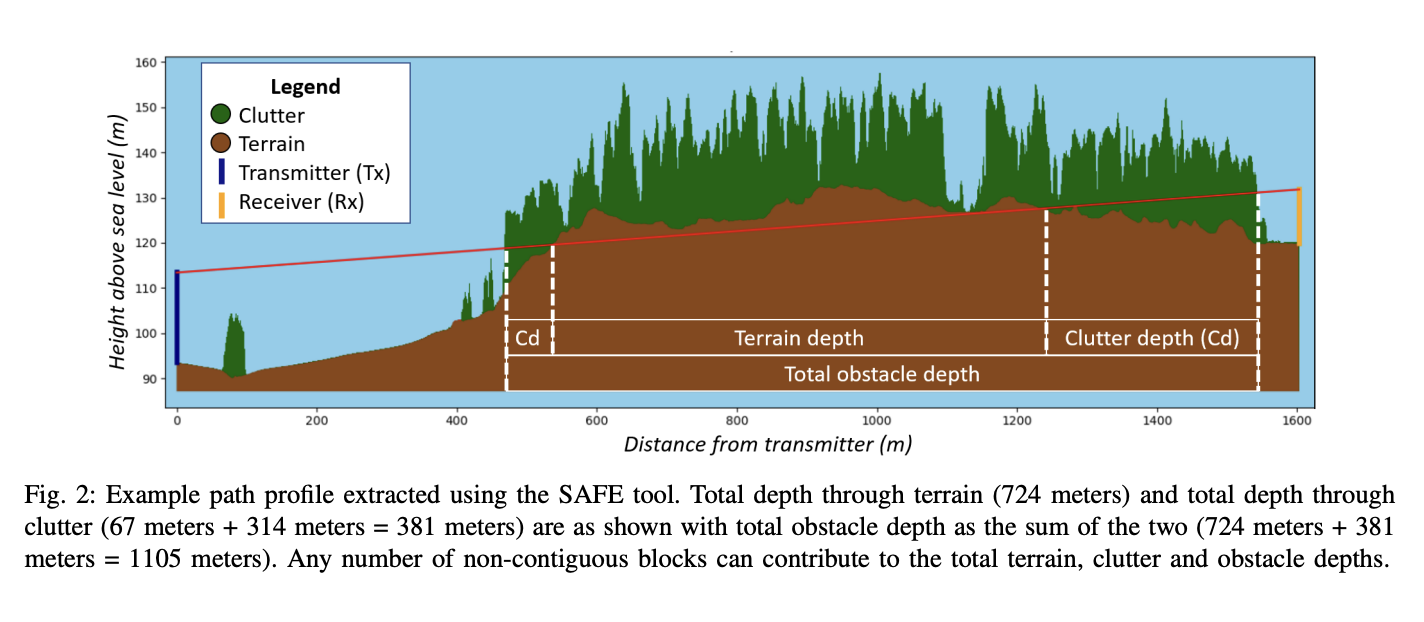
Machine Learning Revolutionizes Path Loss Modeling with Simplified Features
Practical Solutions and Value
Accurate propagation modeling is crucial for effective radio deployments, coverage analysis, and interference mitigation in wireless communications.
Traditional models like Longley-Rice and free space path loss (FSPL) exhibit reduced accuracy in non-line-of-sight (NLOS) scenarios. This is due to their inability to account for signal attenuation and interference from terrain and clutter.
Researchers have leveraged machine learning (ML) models and emphasized the use of measurement data for training to ensure reliable ground truth. Their work showcased that simple features can be used to train accurate ML-based propagation models, yielding well-generalized models with significantly fewer features than traditional models.
The results revealed that the ML-based model outperformed traditional models, leading to lower root mean squared errors (RMSEs). This approach paves the way for more efficient and accurate propagation modeling, ultimately enhancing wireless network planning, deployment, and optimization.
Check out the full research paper here.
Evolving with AI Solutions
Discover how AI can redefine your way of work, stay competitive, and revolutionize path loss modeling with simplified features.
Identify Automation Opportunities: Locate key customer interaction points that can benefit from AI.
Define KPIs: Ensure your AI endeavors have measurable impacts on business outcomes.
Select an AI Solution: Choose tools that align with your needs and provide customization.
Implement Gradually: Start with a pilot, gather data, and expand AI usage judiciously.
Contact us at hello@itinai.com for AI KPI management advice and continuous insights into leveraging AI.
Practical AI Solution: AI Sales Bot
Consider the AI Sales Bot designed to automate customer engagement 24/7 and manage interactions across all customer journey stages.
Explore solutions at itinai.com.