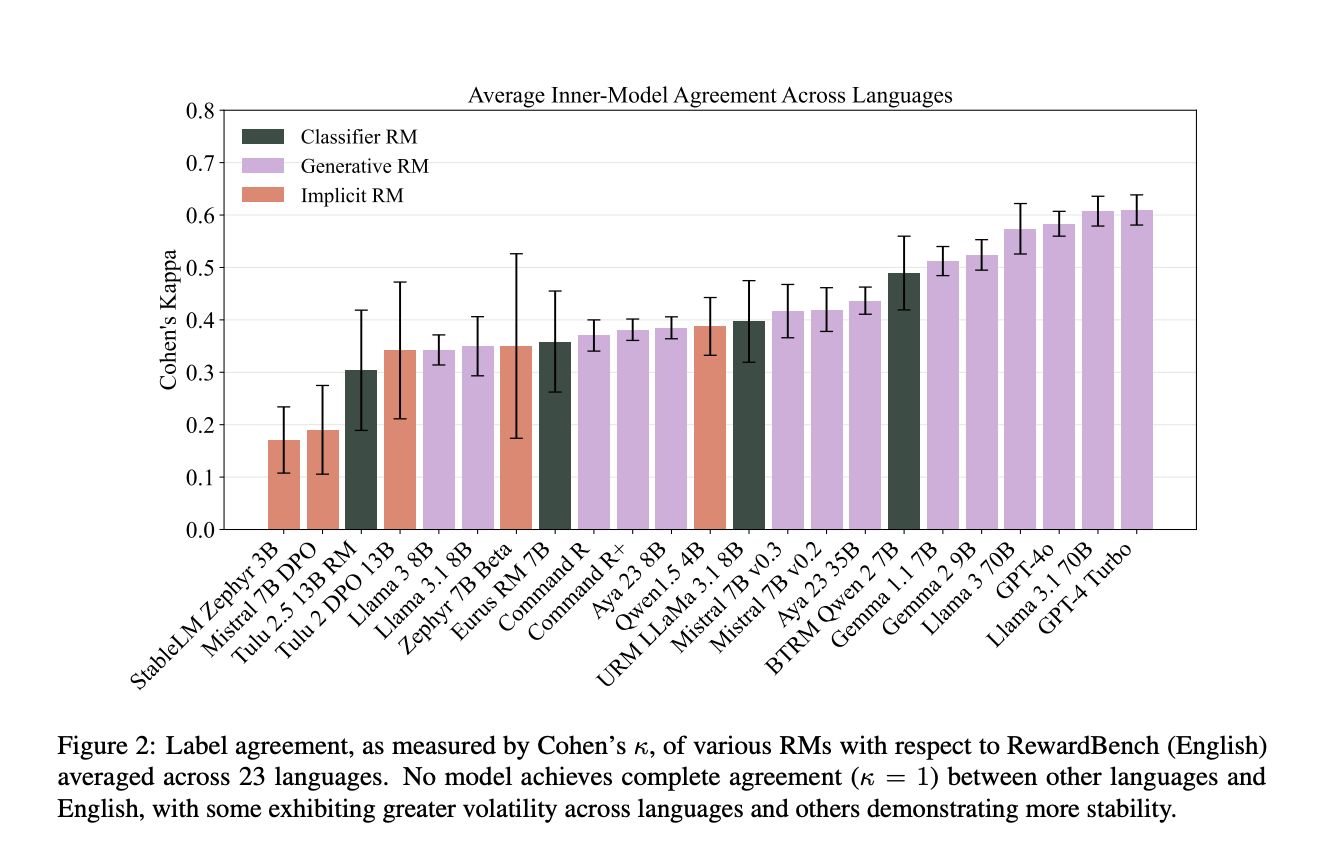
Transforming AI with Multilingual Reward Models
Introduction to Large Language Models (LLMs)
Large language models (LLMs) are changing how we interact with technology, improving areas like customer service and healthcare. They align their responses with human preferences through reward models (RMs), which act as feedback systems to enhance user experience.
The Need for Multilingual Adaptation
While many advancements have been made for English, adapting RMs for multiple languages is crucial. This ensures that users worldwide can access accurate and culturally relevant information. Currently, many RMs struggle to perform well in non-English languages, highlighting the need for better evaluation tools.
Current Evaluation Tools and Their Limitations
Existing tools like RewardBench assess RMs primarily in English, focusing on reasoning and safety. However, they do not adequately evaluate translation tasks or cross-cultural responses, which are essential for a global audience.
Introducing M-RewardBench
Researchers have developed M-RewardBench, a new benchmark that evaluates RMs across 23 languages. This tool includes 2,870 preference instances from various language families, providing a comprehensive testing environment for multilingual capabilities.
Methodology of M-RewardBench
M-RewardBench uses both machine-generated and human-verified translations to ensure accuracy. It assesses RMs in categories like Chat, Safety, and Reasoning, revealing how well these models perform in different conversational contexts.
Key Findings
- Dataset Scope: Covers 23 languages and 2,870 instances, making it a leading multilingual evaluation tool.
- Performance Gaps: Generative RMs scored an average of 83.5% in multilingual settings, but performance dropped by up to 13% for non-English tasks.
- Task-Specific Variations: More complex tasks like Chat-Hard showed greater performance drops compared to simpler reasoning tasks.
- Translation Quality Impact: Better translations improved RM accuracy by up to 3%, highlighting the need for high-quality translation methods.
- Consistency in High-Resource Languages: Models performed better in languages like Portuguese (68.7%) compared to lower-resource languages like Arabic (62.8%).
Conclusion
The research behind M-RewardBench emphasizes the importance of aligning language models with human preferences across diverse languages. This benchmark sets the stage for future improvements in reward modeling, focusing on cultural nuances and language consistency.
Get Involved
Check out the Paper, Project, and GitHub. Follow us on Twitter, join our Telegram Channel, and connect with our LinkedIn Group. If you appreciate our work, subscribe to our newsletter and join our 55k+ ML SubReddit.
Upcoming Webinar
Live Webinar on Oct 29, 2024: Discover the Best Platform for Serving Fine-Tuned Models: Predibase Inference Engine.
AI Solutions for Your Business
To stay competitive and leverage AI effectively, consider the following steps:
- Identify Automation Opportunities: Find key customer interactions that can benefit from AI.
- Define KPIs: Ensure measurable impacts from your AI initiatives.
- Select an AI Solution: Choose tools that fit your needs and allow customization.
- Implement Gradually: Start small, gather data, and expand AI usage wisely.
For AI KPI management advice, connect with us at hello@itinai.com. For ongoing insights, follow us on Telegram or Twitter.
Explore how AI can enhance your sales processes and customer engagement at itinai.com.