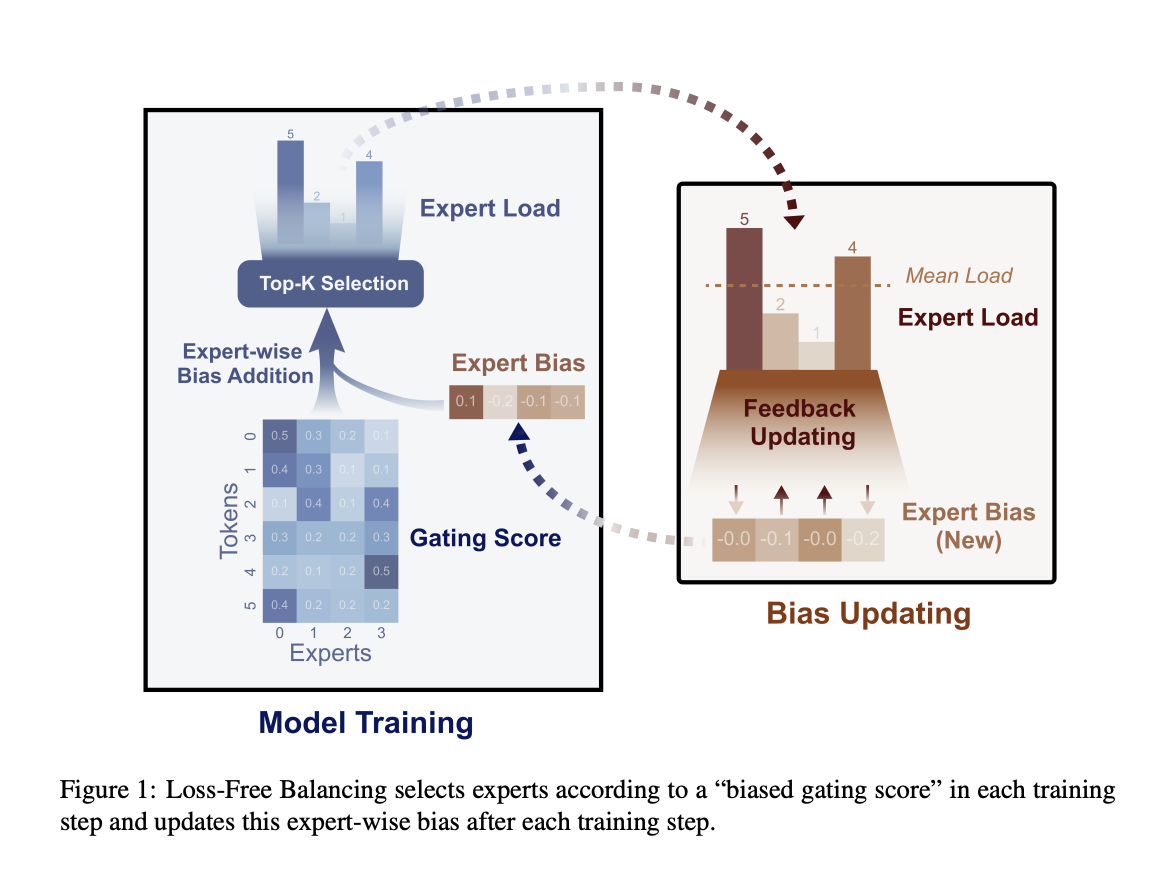
Mixture-of-Experts Models and Load Balancing
Practical Solutions and Value
Mixture-of-experts (MoE) models are crucial for large language models (LLMs), handling diverse and complex tasks efficiently in natural language processing (NLP).
Load imbalance among experts is a significant challenge, impacting the model’s ability to perform optimally when scaling up to handle large datasets and complex language processing tasks.
Traditional methods using auxiliary loss functions to mitigate load imbalance introduce undesired gradients, hindering the model’s performance.
The Loss-Free Balancing method dynamically adjusts task routing to experts based on their current load, ensuring a balanced distribution without interfering with the model’s primary training objectives.
Empirical results show that Loss-Free Balancing significantly improves load balance and overall model performance, reducing validation perplexity and achieving better load balance metrics compared to traditional methods.
The method’s adaptability and potential for further optimization highlight its effectiveness in enhancing MoE models’ performance.
If you want to evolve your company with AI, stay competitive, and use Loss-Free Balancing to enhance performance across various applications.
AI Solutions for Business Transformation
Practical Steps for AI Integration
Identify Automation Opportunities: Locate key customer interaction points that can benefit from AI.
Define KPIs: Ensure your AI endeavors have measurable impacts on business outcomes.
Select an AI Solution: Choose tools that align with your needs and provide customization.
Implement Gradually: Start with a pilot, gather data, and expand AI usage judiciously.
For AI KPI management advice, connect with us at hello@itinai.com.
For continuous insights into leveraging AI, stay tuned on our Telegram t.me/itinainews or Twitter @itinaicom.
Discover how AI can redefine your sales processes and customer engagement. Explore solutions at itinai.com.