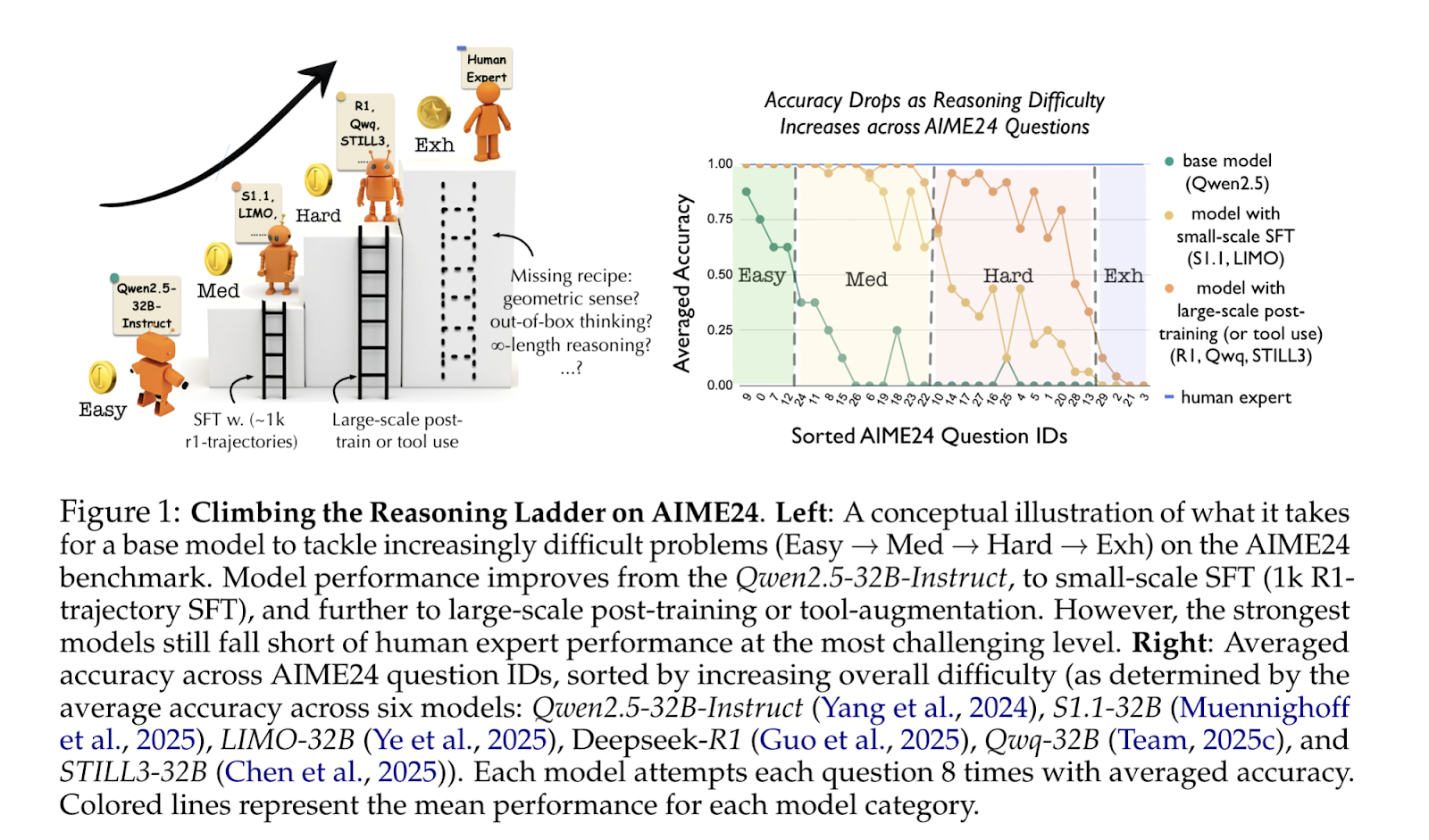
Unlocking Mathematical Reasoning in AI Models
Introduction
Recent advancements in large language models (LLMs) indicate that they can effectively tackle challenging mathematical problems with minimal data. Researchers from UC Berkeley and the Allen Institute for AI have developed a fine-tuning strategy that enhances these models’ capabilities across varying levels of difficulty.
Understanding the Progress
While fine-tuning methods like LIMO and s1 have shown significant improvements, questions remain regarding whether models can generalize their learning beyond the training data or if they are simply overfitting. The research community is striving to identify the exact strengths and weaknesses of these advanced models, as understanding their true reasoning capabilities is essential for leveraging AI effectively in business.
Challenges in Current Approaches
Various studies have examined the impact of supervised fine-tuning (SFT) on reasoning tasks. However, existing methods often fall short in determining the granularity of improvement across different problem categories. Key questions include:
- Do models merely improve on previously encountered problem types?
- Can they transfer problem-solving strategies to new contexts?
- What specific question types become solvable through fine-tuning?
Proposed Methodology
The research team proposes a tiered analysis framework utilizing the AIME24 dataset, known for its structured difficulty levels. The dataset categorizes questions into four tiers: Easy, Medium, Hard, and Extremely Hard. This systematic approach allows for a detailed examination of the requirements needed to advance through each level, highlighting critical insights regarding the capabilities of fine-tuned models.
Key Insights from Research
- The gap between potential performance and stability in SFT models.
- Minimal advantages from meticulous dataset curation.
- Diminishing returns from enlarging SFT datasets.
- Identification of intelligence barriers that may not be surmountable through SFT alone.
Case Studies and Data Analysis
The study employed a comprehensive analysis by examining multiple training variables, such as:
- Category of math problems
- Number of examples per category
- Length of reasoning trajectories
- Style of problem-solving trajectories
Findings indicate that a minimum of 500 normal or long R1-style trajectories is essential for achieving over 90% accuracy on Medium-level questions. This suggests that the structure and length of reasoning trajectories are more critical than the content-specific elements.
Implications for Business Applications
Given the findings, businesses can leverage AI in several practical ways:
- Identify Automation Opportunities: Look for repetitive tasks that AI can handle effectively.
- Enhance Customer Interactions: Use AI to streamline customer service processes and improve engagement.
- Monitor KPIs: Establish key performance indicators (KPIs) to assess the success of AI implementations.
- Choose Customizable Tools: Select AI tools that align with your business objectives and can be tailored to your needs.
- Start Small: Implement AI solutions in manageable projects first to gauge effectiveness before scaling up.
Conclusion
Advancements in fine-tuning LLMs reveal significant potential in enhancing mathematical reasoning capabilities. As businesses explore the integration of AI technologies, understanding the nuances of these models can inform strategic implementations and maximize their impact. By continuously assessing and refining AI applications, organizations can unlock new levels of efficiency and innovation.