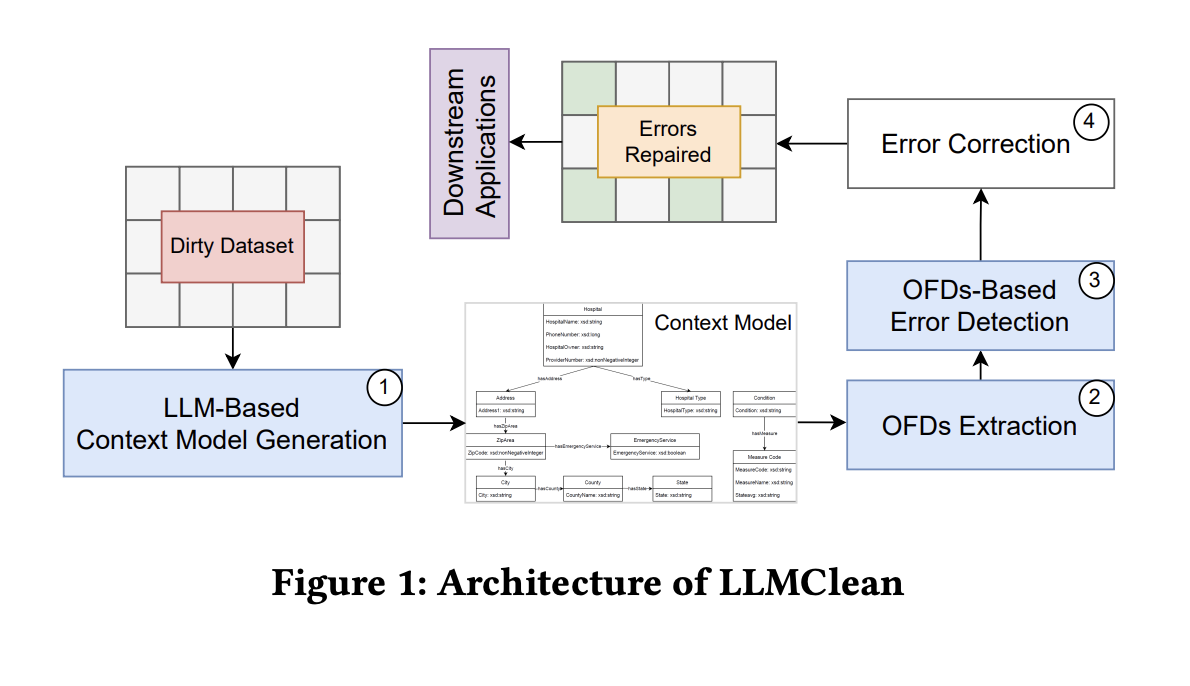
The Challenge of Data Quality in the IoT Era
The rapid growth of IoT has led to a flood of data, creating a challenge for ensuring data quality. Poor-quality data can undermine the effectiveness of Machine Learning applications, introducing biases and inaccuracies.
Automated Data Cleaning Tools
To address data quality issues, automated data cleaning tools have been developed. However, many of these tools lack context awareness, which is crucial for effective data cleaning within ML workflows.
Context-Aware Data Cleaning Tools
Context-aware data cleaning tools leverage Ontological Functional Dependencies (OFDs) to capture semantic relationships between attributes, enhancing error detection and correction precision.
The Solution: LLMClean
LLMClean leverages large language models (LLMs) to automatically generate context models from real-world data, addressing scalability, adaptability, and consistency challenges. It encompasses a three-stage architectural framework for identifying erroneous instances in tabular data.
Benefits of LLMClean
LLMClean provides a robust data cleaning and analytical framework tailored to the evolving nature of real-world data, including IoT datasets. It introduces Sensor Capability Dependencies and Device-Link Dependencies for precise error detection.
Evolve Your Company with AI
Discover how AI can redefine your way of work and stay competitive. Identify Automation Opportunities, Define KPIs, Select an AI Solution, and Implement Gradually. Connect with us for AI KPI management advice and insights into leveraging AI.
Practical AI Solution: AI Sales Bot
Consider the AI Sales Bot designed to automate customer engagement 24/7 and manage interactions across all customer journey stages. Explore solutions at itinai.com/aisalesbot.