Here is a summary of the text: Non-profit researchers have made several advancements in artificial intelligence (AI) in 2023. These include methods like ALiBi and Scaling Laws of RoPE-based Extrapolation, which improve the extrapolation capabilities of AI models. Other advancements include FlashAttention for training transformers faster, Branchformer for speech processing, Latent Diffusion Models for image processing tasks, CLIP-Guidance for text-to-3D generation, GPT-NeoX for language modeling, QLoRA for efficient fine-tuning, and the RMKV model that combines the strengths of transformers and recurrent neural networks. These advancements contribute to the development of more efficient AI models.
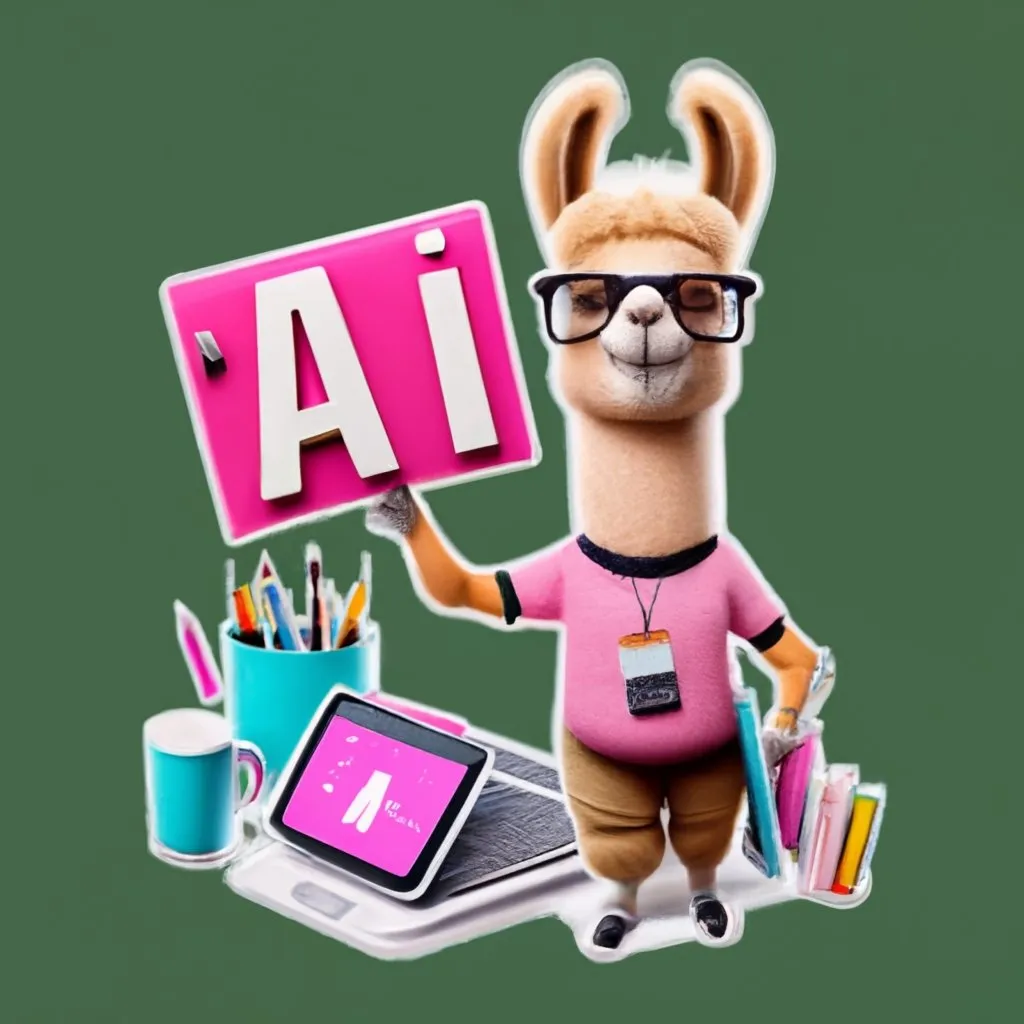
List of Artificial Intelligence AI Advancements by Non-Profit Researchers
Last year and this year, 2023, have seen significant advancements in AI applications thanks to the efforts of non-profit researchers. Here are some notable developments:
ALiBi
ALiBi is a simple method that addresses the issue of text extrapolation in Transformers. It allows models to extrapolate text sequences longer than their training data without affecting runtime or requiring additional parameters.
Scaling Laws of RoPE-based Extrapolation
This framework improves the extrapolating capabilities of transformers by fine-tuning a Rotary Position Embedding (RoPe) based LLM with different pre-training context lengths.
FlashAttention
FlashAttention is an IO-aware algorithm that trains transformers faster than existing methods, reducing the memory requirements for large text sequences.
Branchformer
Branchformer is an alternative encoder to Conformers (a variant of Transformers) used in speech processing. It offers a flexible and interpretable architecture with parallel branches to model dependencies in speech-processing tasks.
Latent Diffusion
Latent Diffusion Models achieve high performance on image-based tasks while requiring fewer computational resources compared to traditional Diffusion Models.
CLIP-Guidance
CLIP-Guidance is a method for text-to-3D generation that leverages pretrained vision-language models like CLIP to generate images from text descriptions of 3D objects, without the need for large-scale labeled datasets.
GPT-NeoX
GPT-NeoX is an autoregressive language model with 20B parameters that performs well on knowledge-based and mathematical tasks. Its model weights are publicly available to promote research in various areas.
QLoRA
QLoRA is a fine-tuning approach that reduces memory usage while maintaining optimal task performance. It allows for efficient fine-tuning of large models on limited GPU resources.
RMKV
The Receptance Weighted Key Value (RMKV) model combines the strengths of Transformers and Recurrent Neural Networks (RNNs) to achieve comparable performance to Transformers with fewer drawbacks.
These advancements are the result of the hard work and dedication of researchers in their respective projects. To stay updated on the latest AI research news and projects, you can join our ML SubReddit, Facebook Community, Discord Channel, and Email Newsletter.
If you want to leverage AI for your company and stay competitive, consider implementing these advancements. Discover how AI can redefine your work processes and identify automation opportunities. Define measurable KPIs and select AI solutions that align with your needs. Start with a pilot implementation and gradually expand AI usage.
For AI KPI management advice and continuous insights into leveraging AI, you can connect with us at hello@itinai.com. To explore practical AI solutions for sales processes and customer engagement, check out our AI Sales Bot at itinai.com/aisalesbot.