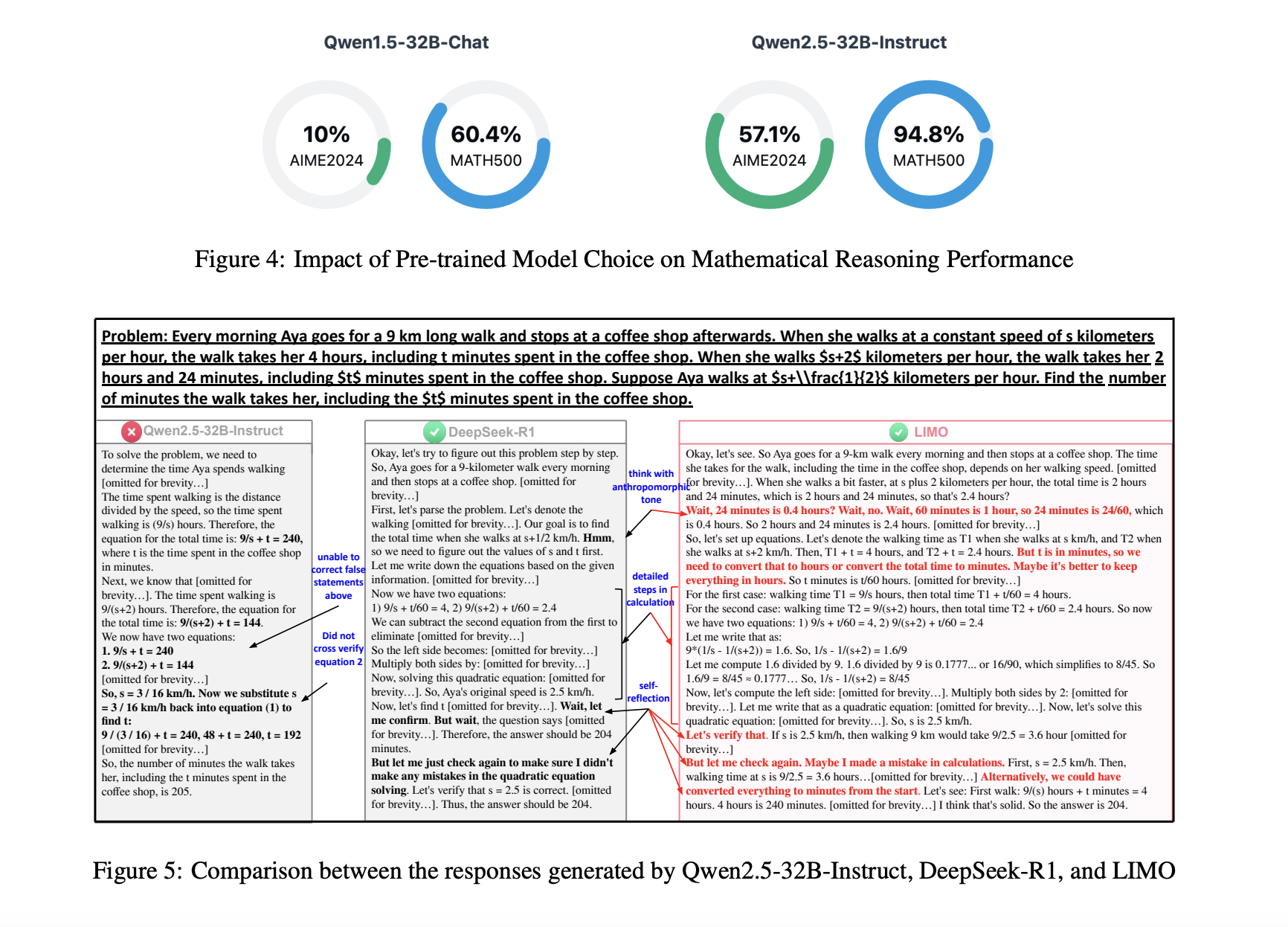
Challenges in Reasoning Tasks for Language Models
Reasoning tasks remain a significant challenge for many language models. Developing reasoning skills, especially for programming and math, is still a distant goal. This difficulty arises from the complexity of these tasks, which require multi-step logical deductions and domain knowledge to find structured solutions.
Current Training Methods
Language models are trained on vast amounts of data, often requiring hundreds of thousands of examples. This training is based on two main assumptions: first, that cognitive skills can only be learned through numerous supervised examples, and second, that this leads to memorization rather than true understanding. Additionally, this approach incurs high computational costs and demands extensive data collection.
Introducing the Less-Is-More (LIMO) Hypothesis
Researchers from Shanghai Jiao Tong University propose the Less-Is-More (LIMO) hypothesis. This suggests that sophisticated reasoning capabilities can be developed in models with minimal, precise demonstrations of cognitive processes, provided that domain knowledge is well-encoded during pre-training.
Key Factors of the LIMO Hypothesis
- Prerequisite Knowledge: The model’s parameter space contains essential domain knowledge from pre-training.
- Minimal Exemplars: Effective examples that demonstrate systematic problem-solving processes act as cognitive prompts during reasoning tasks.
Benefits of the LIMO Approach
LIMO focuses on the quality and structure of prompts rather than quantity, encouraging the model to utilize past lessons instead of merely recalling them. This challenges the idea that supervised fine-tuning leads to mere memorization.
Research Findings
The authors conducted experiments using only hundreds of examples instead of the typical hundreds of thousands. LIMO showed impressive results across 10 benchmarks, achieving:
- 57.1% accuracy on the challenging American Invitational Mathematics Examination (AIME) with just 817 curated training samples.
- 94.8% accuracy on the MATH dataset, outperforming traditional supervised fine-tuning methods.
LIMO achieved a remarkable 40.5% improvement over models trained on significantly larger datasets, challenging the assumptions of supervised training.
Conclusion
The LIMO model provides valuable insights into reasoning training for language models, demonstrating that quality training can surpass quantity. It shows exceptional performance on challenging datasets, proving that less can indeed be more.
Explore Further
Check out the Paper. All credit goes to the researchers behind this project. Follow us on Twitter and join our 75k+ ML SubReddit.
Transform Your Business with AI
Stay competitive by leveraging LIMO: The AI Model that Proves Quality Training Beats Quantity.
How AI Can Enhance Your Operations
- Identify Automation Opportunities: Find key customer interaction points that can benefit from AI.
- Define KPIs: Ensure your AI initiatives have measurable impacts on business outcomes.
- Select an AI Solution: Choose tools that fit your needs and allow for customization.
- Implement Gradually: Start with a pilot project, gather data, and expand AI usage wisely.
For AI KPI management advice, connect with us at hello@itinai.com. For ongoing insights into leveraging AI, follow us on Telegram or @itinaicom.
Discover how AI can transform your sales processes and customer engagement. Explore solutions at itinai.com.