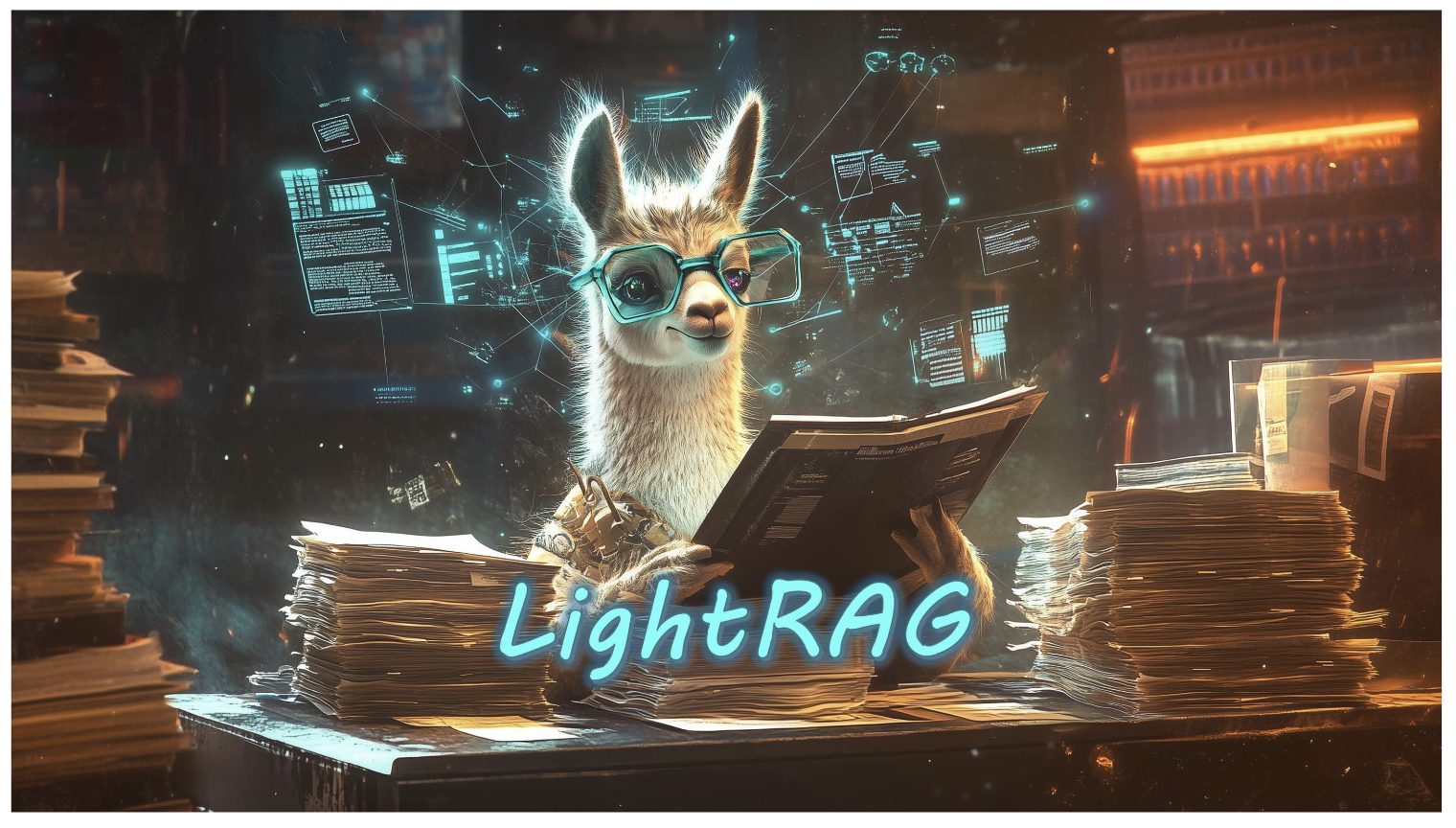
Understanding Retrieval-Augmented Generation (RAG)
Retrieval-augmented generation (RAG) combines external knowledge with large language models (LLMs) to provide accurate and relevant answers. This method is valuable in applications like AI question-answering systems, knowledge retrieval platforms, and content creation tools that need current information.
Challenges with Traditional RAG Systems
Traditional RAG systems struggle with complex relationships between information. They often use flat data representations, leading to fragmented responses when queries involve multiple topics. As demand for sophisticated AI responses grows, the ability to synthesize information from various sources is crucial.
Limitations of Existing Methods
Current RAG tools focus on breaking down text into smaller chunks for easier retrieval. While effective, they often fail to connect related information and adapt quickly to new data, making them less efficient in fast-paced environments.
Introducing LightRAG
A research team from Beijing University of Posts and Telecommunications and the University of Hong Kong developed LightRAG to address these challenges. This innovative framework uses graph structures to capture complex relationships in data, enhancing retrieval speed and accuracy.
Key Features of LightRAG
- Dual-Level Retrieval: Combines detailed and conceptual information retrieval for comprehensive answers.
- Incremental Updates: Allows real-time data integration without reprocessing the entire database, making it responsive to changes.
Proven Performance
LightRAG has outperformed existing RAG methods in accuracy and efficiency across various datasets. For instance, in legal datasets, it achieved over 80% retrieval accuracy, significantly higher than other models.
Adaptability Advantage
Unlike traditional methods, LightRAG quickly adapts to new data without disrupting existing structures, making it ideal for rapidly changing fields like legal and medical research.
Conclusion
LightRAG offers a powerful solution to the limitations of traditional RAG systems. By integrating graph structures and a dual-level retrieval framework, it enhances the ability to retrieve and synthesize complex information efficiently.
Stay Connected
Check out the Paper and GitHub. Follow us on Twitter, join our Telegram Channel, and be part of our LinkedIn Group. If you enjoy our work, subscribe to our newsletter and join our 50k+ ML SubReddit.
Upcoming Event
RetrieveX – The GenAI Data Retrieval Conference on Oct 17, 2024.
Transform Your Business with AI
Use LightRAG to stay competitive and redefine your work processes:
- Identify Automation Opportunities: Find key customer interactions that can benefit from AI.
- Define KPIs: Ensure measurable impacts from your AI initiatives.
- Select an AI Solution: Choose tools that fit your needs and allow customization.
- Implement Gradually: Start small, gather data, and expand wisely.
For AI KPI management advice, connect with us at hello@itinai.com. For ongoing insights, follow us on Telegram or Twitter.
Explore how AI can enhance your sales processes and customer engagement at itinai.com.