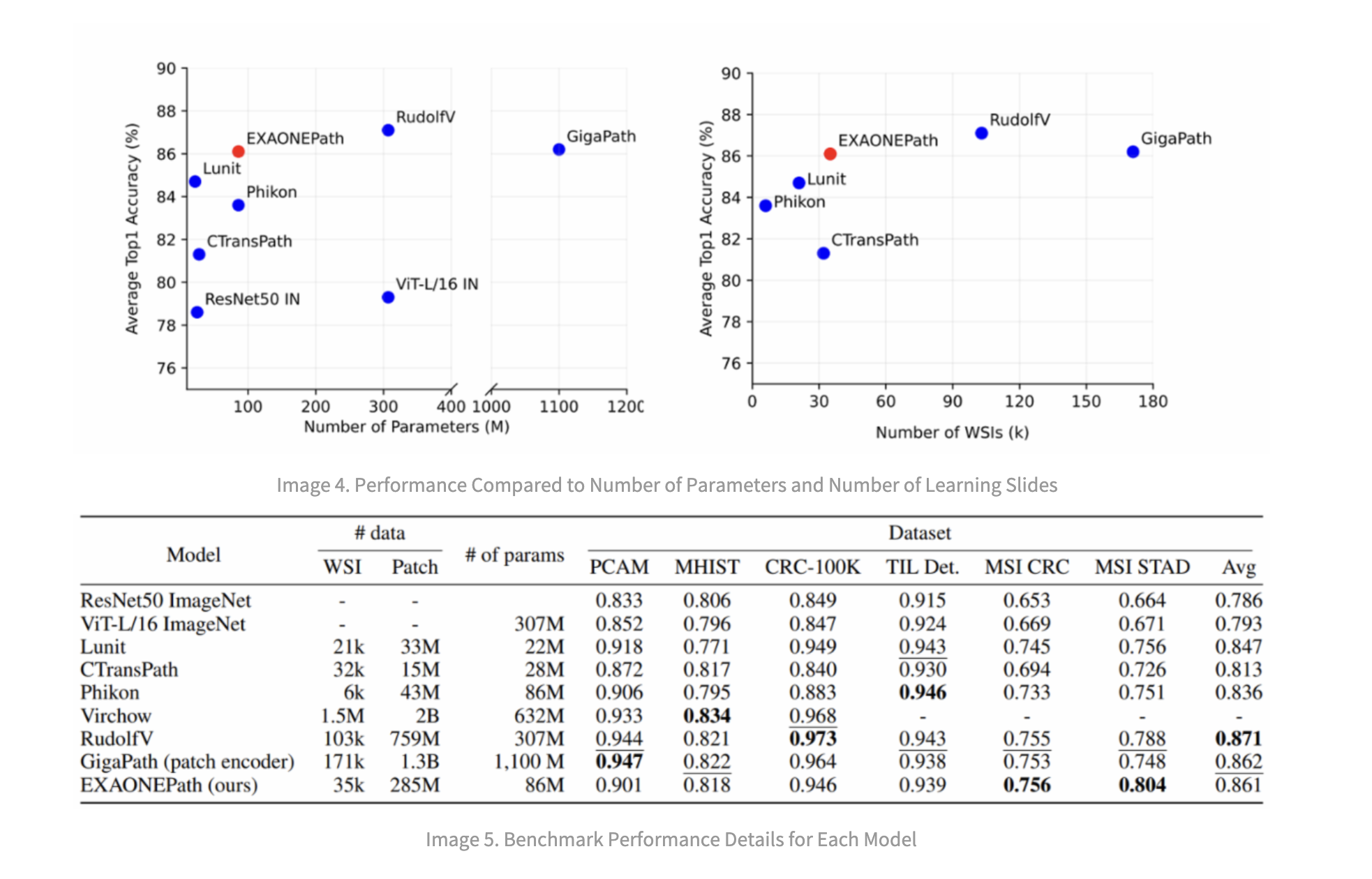
Introduction to EXAONEPath: A New Frontier in Digital Histopathology
EXAONEPath is a groundbreaking model designed to transform digital histopathology by efficiently processing histopathology images for medical diagnostics. It reduces genetic testing time, saves costs, and enhances patient care.
Technical Innovations in EXAONEPath: Overcoming WSI-Specific Feature Collapse
EXAONEPath addresses the challenge of WSI-specific feature collapse by employing self-supervised learning and stain normalization techniques to standardize color characteristics and preserve intricate details of cell structures and tissues.
Multi-Instance Learning (MIL) Framework: A Cornerstone in Histopathology Image Processing
The MIL framework divides WSIs into smaller patches, processes them through a pre-trained image encoder, and integrates the resulting vectors to ensure preservation of cell structures and tissues. EXAONEPath excels in processing gigapixel-scale histopathology images using this framework.
Advancing Self-Supervised Learning: Overcoming Color-Based Feature Collapse
EXAONEPath employs stain normalization to focus on learning pathological features rather than color variations, improving the quality of the model’s outputs and setting a new standard in histopathology image encoders.
Training EXAONEPath: A Rigorous and Ethical Approach
EXAONEPath was trained on a diverse and representative dataset using self-supervised learning methods, ensuring robust features critical for downstream tasks such as cancer classification and survival analysis. Ethical considerations were embedded into the model’s development and deployment.
Performance Evaluation: Benchmarking EXAONEPath Against the State-of-the-Art
EXAONEPath’s performance was rigorously evaluated across diverse patch-level tasks, demonstrating competitive results and setting new standards in digital histopathology.
New Horizons: Potential Applications and Future Directions
EXAONEPath has the potential to revolutionize medical diagnostics and personalized medicine. Future research may focus on developing more advanced stain normalization techniques and exploring new model architectures in histopathology.
Ethical Considerations: Ensuring Responsible Use of AI in Histopathology
LG AI Research has implemented strict guidelines to ensure the ethical and responsible use of EXAONEPath, setting a standard for the deployment of AI in medical applications.
Explore the Innovation of EXAONEPath: A Breakthrough in Digital Histopathology
EXAONEPath, released as open-source, sets new standards in digital histopathology and unlocks transformative possibilities for AI-driven healthcare innovations.
Conclusion: A New Era in Digital Histopathology
EXAONEPath is a remarkable feat in digital histopathology, offering valuable tools for pathologists worldwide and paving the way for more accurate, efficient, and ethical diagnostic tools.