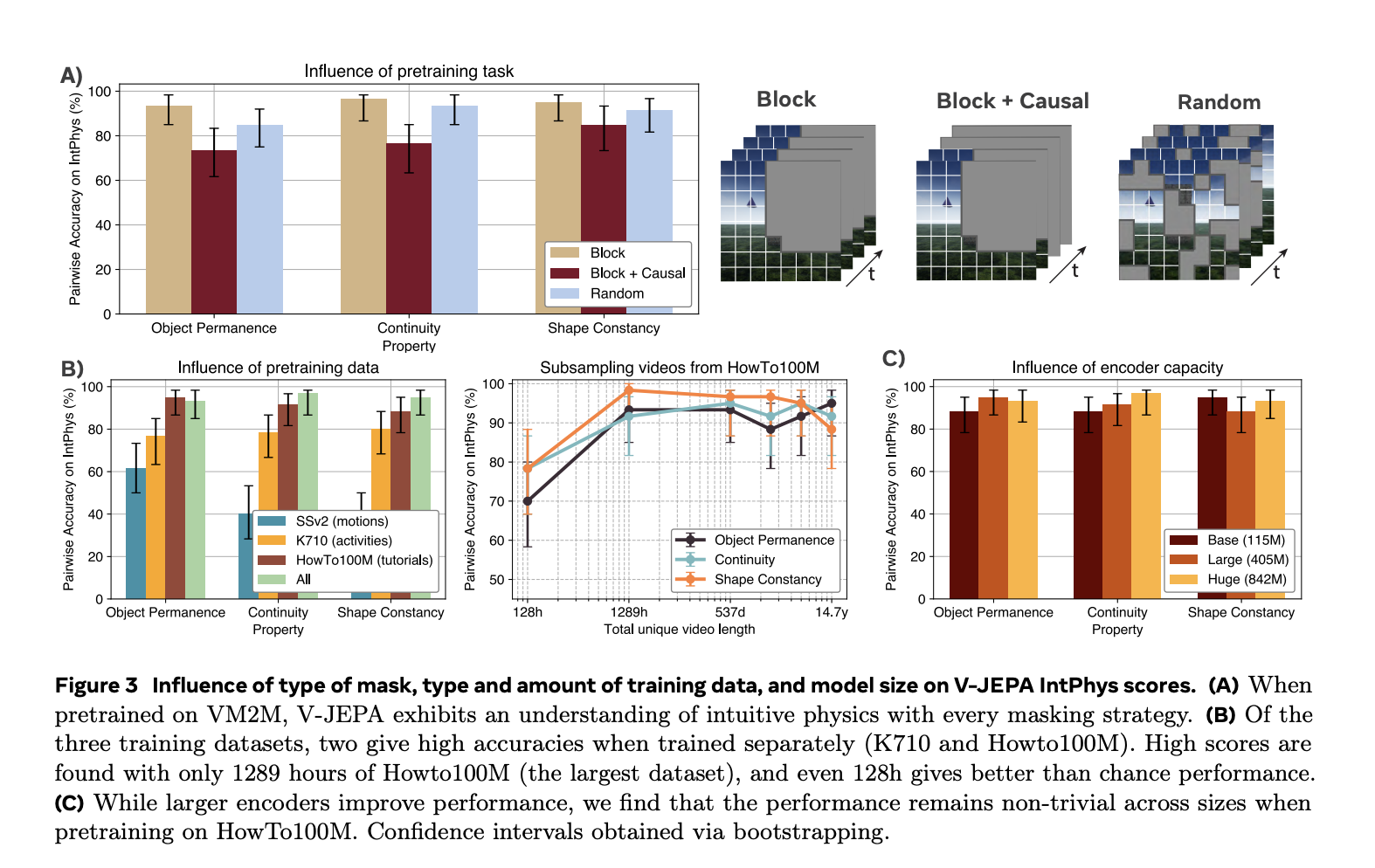
Understanding Intuitive Physics in AI
Humans naturally understand how objects behave, such as not expecting sudden changes in their position or shape. This understanding is seen even in infants and animals, supporting the idea that humans have evolved to reason about objects and space.
AI’s Challenge with Intuitive Physics
While AI excels in complex tasks like programming, it struggles with intuitive physics, known as Moravec’s paradox. AI can learn physics in two main ways:
- Structured Models: These use specific rules to simulate interactions between objects.
- Pixel-Based Models: These predict future sensory information without clear abstractions.
Research Insights
Researchers from Meta and other institutions have studied how deep neural networks learn intuitive physics by predicting missing parts of videos. Their findings suggest that models trained in an abstract way, like Joint Embedding Predictive Architectures (JEPAs), can effectively recognize key physical properties such as object permanence and shape consistency.
The V-JEPA Model
The focus of the study is the V-JEPA model, which predicts future video frames using learned representations. It showed a remarkable 98% accuracy in intuitive physics tests, outperforming other models. Even smaller versions of V-JEPA performed well, challenging the idea that intuition in physics requires innate knowledge.
Evaluating AI’s Understanding
To assess intuitive physics, researchers use the violation-of-expectation method, traditionally applied to infants. This method measures reactions to impossible physical scenarios. The V-JEPA model demonstrated its ability to understand object dynamics by predicting masked video segments, allowing it to anticipate physical events effectively.
Benchmark Performance
V-JEPA was benchmarked against datasets like IntPhys and performed significantly better than other models. This shows that learning in a structured representation space enhances AI’s physical reasoning abilities.
Future Directions
The study concludes that V-JEPA’s understanding of intuitive physics comes from general learning principles rather than just hardwired knowledge. There is potential for improvement by enhancing model memory and integrating action-based learning. Future research may involve training models on infant-like visual data for better physical reasoning.
Practical Applications of AI in Business
To stay competitive and leverage AI effectively:
- Identify Automation Opportunities: Find customer interaction points that can benefit from AI.
- Define KPIs: Ensure your AI initiatives have measurable business impacts.
- Select an AI Solution: Choose tools that fit your needs and allow customization.
- Implement Gradually: Start small, gather data, and expand AI usage wisely.
For expert advice on AI KPI management, connect with us at hello@itinai.com. Stay updated on leveraging AI through our Telegram or follow us on Twitter @itinaicom.
Discover how AI can transform your sales processes and customer engagement by visiting itinai.com.