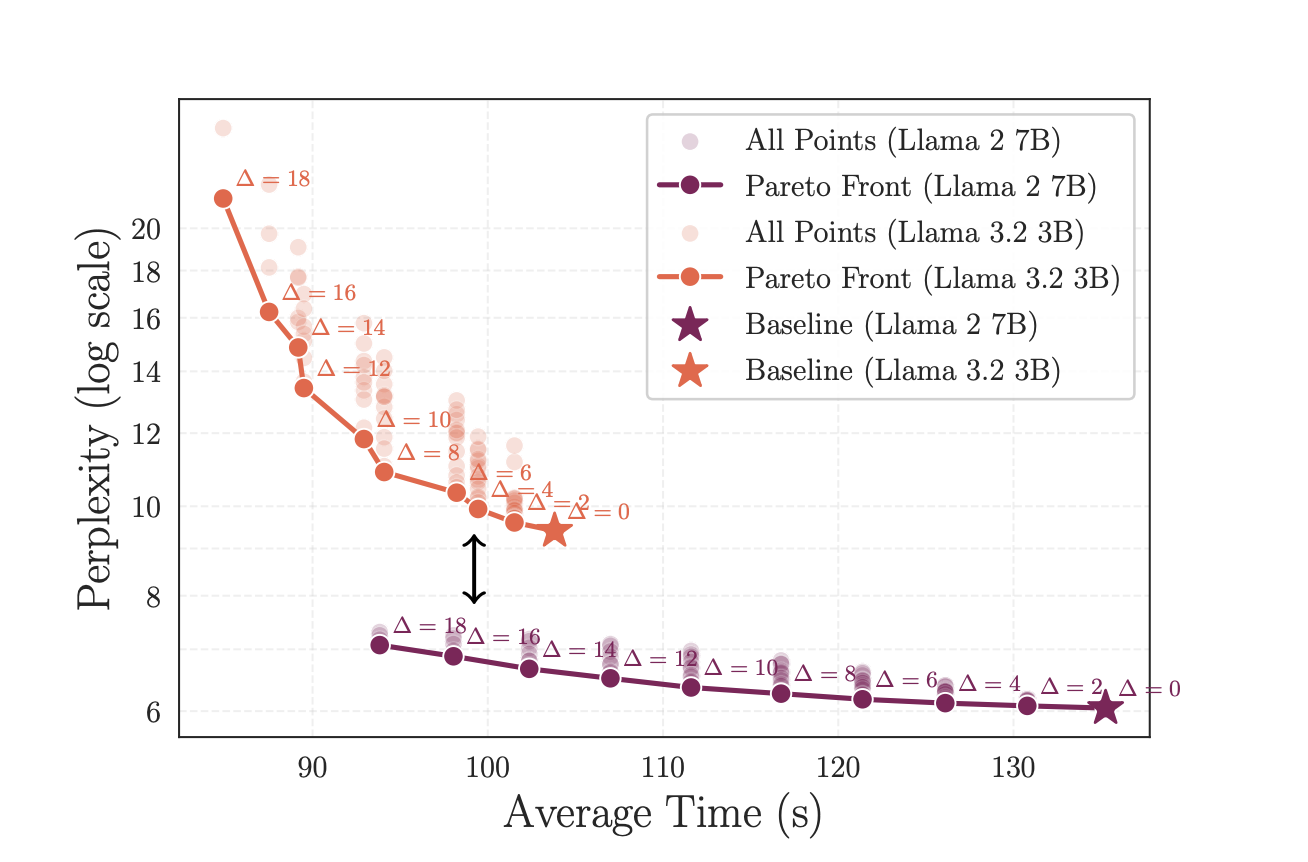
Challenges in Deploying Large Language Models (LLMs)
LLMs are powerful but require a lot of computing power, making them hard to use on a large scale. Optimizing how these models work is essential to improve efficiency, speed, and reduce costs. High-traffic applications can lead to monthly bills in the millions, so finding efficient solutions is crucial. Additionally, deploying these models on devices with limited resources requires strategies that keep performance high while lowering computing demands.
Improving Efficiency with Practical Solutions
Several methods can enhance the efficiency of LLMs:
- Pruning: This technique removes unnecessary parameters, making the model faster and using memory better.
- Quantization: This reduces the precision of calculations, converting them to lower-bit formats, which saves energy and improves hardware efficiency.
- Parallelization: Distributing tasks across multiple processors speeds up inference and reduces communication delays.
Innovative Approaches to Layer Management
Recent research has focused on modifying how layers in LLMs are structured to improve efficiency. By grouping and executing layers in parallel, researchers have found ways to speed up inference without retraining the model. This method maintains a high level of accuracy while significantly enhancing performance.
Key Findings from Recent Research
- Researchers from the University of Geneva, EPFL, and Meta FAIR have developed a method that reduces the depth of LLMs while keeping performance intact.
- By applying transformations like merging and shuffling layers, they demonstrated that certain layers can be reordered or run in parallel with minimal loss in performance.
- Layer Parallelism (LP) allows for faster processing by executing layer pairs simultaneously, leading to significant speed improvements.
Results and Benefits of Layer Parallelism
The study showed that:
- LP reduced model depth by 21% for Llama2 7B and 18% for Llama3.2 3B, resulting in speed increases of 1.29x and 1.22x, respectively.
- Fine-tuning helped recover some accuracy losses, proving the method’s effectiveness.
- Layer Parallelism challenges the traditional view that layers must be processed sequentially, opening new avenues for efficiency.
Next Steps for AI Implementation
To leverage AI effectively in your business:
- Identify Automation Opportunities: Find areas where AI can enhance customer interactions.
- Define KPIs: Ensure your AI initiatives have measurable impacts.
- Select an AI Solution: Choose tools that fit your needs and allow for customization.
- Implement Gradually: Start small, gather data, and expand your AI usage wisely.
Stay Connected and Informed
For more insights on leveraging AI, connect with us at hello@itinai.com, and follow us on Telegram or @itinaicom.
Discover More
Explore how AI can transform your sales processes and customer engagement at itinai.com.