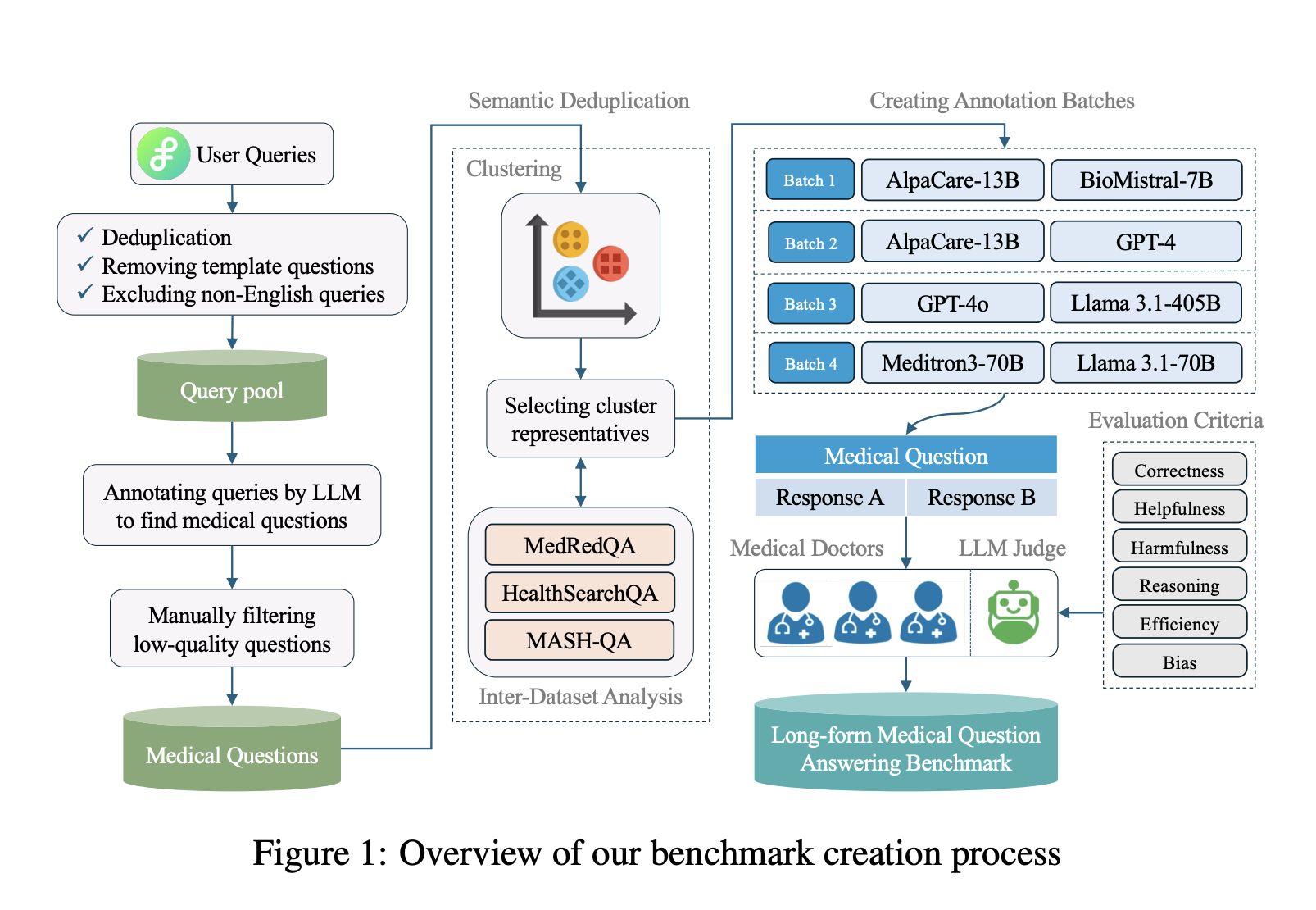
Importance of Medical Question-Answering Systems
Medical question-answering (QA) systems are essential tools for healthcare professionals and the public. Unlike simpler models, long-form QA systems provide detailed answers that reflect the complexities of real-world clinical situations. These systems are designed to understand nuanced questions, even when the information is incomplete or unclear, and deliver reliable, in-depth responses. As reliance on AI for health inquiries grows, the demand for effective long-form QA systems increases, enhancing healthcare accessibility and improving decision-making and patient engagement.
Challenges in Current QA Systems
Despite their potential, long-form QA systems face significant challenges:
- Lack of Benchmarks: There is a need for effective benchmarks to evaluate the performance of large language models (LLMs) in generating long-form answers. Existing benchmarks often rely on automatic scoring and multiple-choice formats, which do not capture the intricacies of real-world clinical settings.
- Transparency Issues: Many benchmarks are closed-source and lack expert annotations, hindering the development of robust QA systems.
- Data Quality Concerns: Some datasets contain errors or outdated information, affecting their reliability for assessments.
Efforts to Improve QA Systems
Various methods have been attempted to address these issues, but they often fall short. Automatic evaluation metrics and curated datasets like MedRedQA and HealthSearchQA provide basic assessments but miss the broader context of long-form answers. The absence of diverse, high-quality datasets and clear evaluation frameworks has slowed the development of effective long-form QA systems.
New Benchmark by Lavita AI and Partners
A team from Lavita AI, Dartmouth Hitchcock Medical Center, and Dartmouth College has created a publicly accessible benchmark to comprehensively evaluate long-form medical QA systems. This benchmark includes:
- Over 1,298 real-world medical questions annotated by medical professionals.
- Performance criteria such as correctness, helpfulness, reasoning, harmfulness, efficiency, and bias.
- A diverse dataset enhanced by human expert annotations and advanced clustering techniques.
Research Methodology
The research involved a multi-phase approach:
- Collection of over 4,271 user queries from Lavita Medical AI Assist.
- Filtering and deduplication to produce high-quality questions.
- Semantic similarity analysis to ensure a wide range of scenarios.
- Classification of questions into basic, intermediate, and advanced levels.
Key Findings
Insights from the benchmark revealed:
- The dataset includes 1,298 curated medical questions across different difficulty levels.
- Models were evaluated on six criteria: correctness, helpfulness, reasoning, harmfulness, efficiency, and bias.
- Llama-3.1-405B-Instruct outperformed GPT-4o, while AlpaCare-13B surpassed BioMistral-7B.
- Specialized model Meditron3-70B did not significantly outperform its general-purpose counterpart.
- Open models showed equal or superior performance to closed systems, indicating the potential of open-source solutions in healthcare.
Conclusion
This study addresses the lack of robust benchmarks for long-form medical QA by introducing a dataset of 1,298 expert-annotated medical questions evaluated across six performance metrics. The results highlight the superior performance of open models like Llama-3.1-405B-Instruct, emphasizing the viability of open-source solutions for privacy-conscious and transparent healthcare AI.
Get Involved
For more insights, check out the Paper and GitHub Page. Follow us on Twitter, join our Telegram Channel, and connect with our LinkedIn Group. If you appreciate our work, subscribe to our newsletter and join our 60k+ ML SubReddit.
Transform Your Business with AI
Stay competitive and leverage AI solutions to evolve your company:
- Identify Automation Opportunities: Find key customer interaction points that can benefit from AI.
- Define KPIs: Ensure your AI initiatives have measurable impacts on business outcomes.
- Select an AI Solution: Choose tools that meet your needs and allow for customization.
- Implement Gradually: Start with a pilot, gather data, and expand AI usage wisely.
For AI KPI management advice, connect with us at hello@itinai.com. For continuous insights, follow us on Telegram or Twitter.
Explore AI Solutions for Sales and Engagement
Discover how AI can redefine your sales processes and customer engagement at itinai.com.