Knowledge graphs, like the Financial Dynamic Knowledge Graph (FinDKG) and the Knowledge Graph Transformer (KGTransformer), are valuable tools for enhancing AI systems. These graphs capture interconnected facts and temporal dynamics, allowing for better understanding and analysis. The FinDKG, created from financial news, can be used for risk monitoring and investing. The KGTransformer model outperforms other static knowledge graph models by incorporating temporal context and relation-specific parameters. These innovations open up possibilities for applying knowledge graphs in various industries.
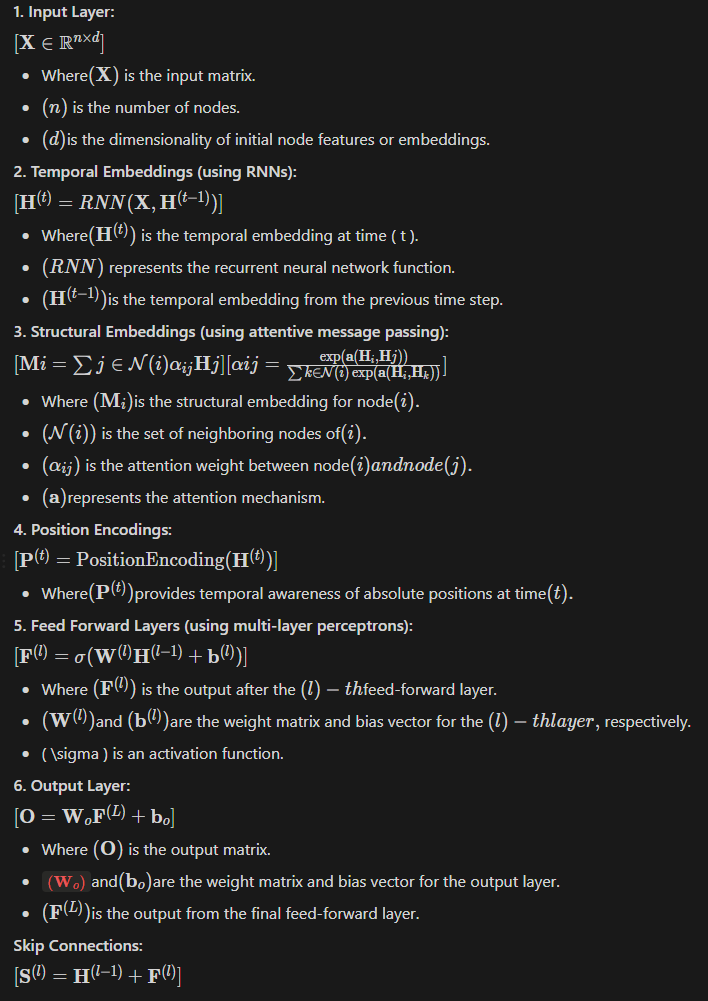
Knowledge Graph Transformers: Architecting Dynamic Reasoning for Evolving Knowledge
Knowledge graphs are a powerful technique for enhancing AI systems by representing facts as interconnected entities. However, real-world knowledge is constantly evolving, requiring dynamic representations that can capture the fluid complexities of the world.
Temporal knowledge graphs (TKGs) fulfill this need by incorporating a temporal dimension, allowing for the modeling of relationships over time. This unlocks new potentials for AI, especially in specialized domains like finance.
Introducing the Financial Dynamic Knowledge Graph (FinDKG)
Xiaohui Victor Li has developed an open-source Financial Dynamic Knowledge Graph (FinDKG) powered by a novel temporal knowledge graph learning model called Knowledge Graph Transformer (KGTransformer). FinDKG is constructed from a corpus of global financial news spanning over two decades, encapsulating both quantitative indicators and qualitative drivers of financial systems into an interconnected, temporal framework.
FinDKG has demonstrated its utility in generating actionable insights for real-world applications like risk monitoring and thematic investing in the financial sector.
Advantages of Knowledge Graph Transformers
Knowledge Graph Transformers (KGTs) are specialized architectures for dynamic learning on TKGs. They incorporate explicit temporal modeling, multi-relational handling, continuous entity tracking, and graph-level summarization to capture the fluid complexities of evolving knowledge graphs.
Potential Applications and Future Directions
KGTs have the potential for various applications, including supply chain management, where they can track supplier performance, forecast demand, and identify risks over time.
Future directions include constructing dynamic knowledge graphs for specialized verticals, enhancing KGTransformer’s capabilities using self-supervised learning, combining large language models with structured knowledge graphs, and exploring architectural improvements.
Implementing AI Solutions for Your Company
If you want to evolve your company with AI, consider using Knowledge Graph Transformers. Identify automation opportunities, define KPIs, select an AI solution, and implement gradually. For AI KPI management advice, connect with us at hello@itinai.com.
For practical AI solutions, explore the AI Sales Bot from itinai.com/aisalesbot. It can automate customer engagement 24/7 and manage interactions across all customer journey stages.
Discover how AI can redefine your sales processes and customer engagement. Visit itinai.com for more information.