GateLoop is a novel sequence model developed by researchers from Johannes Kepler University. It outperforms existing linear recurrent models in auto-regressive language modeling. GateLoop offers low-cost recurrent and efficient parallel modes and introduces a surrogate attention mode with implications for Transformer architectures. It emphasizes the significance of data-controlled cumulative products for more robust sequence models. GateLoop is empirically validated with lower perplexity scores on the WikiText103 dataset. The research highlights the benefits of data-controlled state transitions in sequence modeling and outlines future research possibilities.
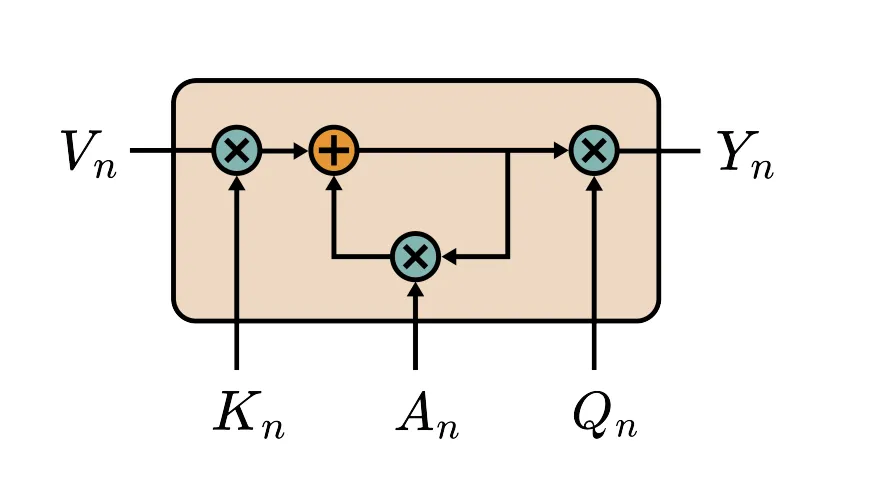
GateLoop: Advancing Sequence Modeling with Linear Recurrence and Data-Controlled State Transitions
GateLoop is a versatile sequence model that enhances linear recurrent models for efficient long-sequence modeling. It outperforms existing models in auto-regressive language modeling and offers low-cost recurrent and efficient parallel modes. GateLoop introduces a surrogate attention mode with implications for Transformer architectures, providing data-controlled relative-positional information to Attention.
Key Features of GateLoop:
– Extends the capabilities of linear recurrent models like S4, S5, LRU, and RetNet
– Employs data-controlled state transitions for improved performance
– Excels in auto-regressive language modeling
– Offers cost-efficient recurrent and highly efficient parallel modes
– Provides versatile operational modes, including a surrogate attention mode
Benefits of GateLoop:
– Lower perplexity scores on the WikiText103 dataset compared to existing models
– Addresses the challenges of long-range dependencies in machine learning
– Overcomes the stability issues faced by traditional Recurrent Neural Networks (RNNs)
– Enables efficient parallel training and global pairwise dependencies
– Empirically validated for its autoregressive natural language modeling prowess
Practical Solutions for AI Implementation:
– Identify Automation Opportunities: Locate key customer interaction points that can benefit from AI.
– Define KPIs: Ensure your AI endeavors have measurable impacts on business outcomes.
– Select an AI Solution: Choose tools that align with your needs and provide customization.
– Implement Gradually: Start with a pilot, gather data, and expand AI usage judiciously.
Spotlight on AI Sales Bot:
Consider the AI Sales Bot from itinai.com/aisalesbot designed to automate customer engagement 24/7 and manage interactions across all customer journey stages. Discover how AI can redefine your sales processes and customer engagement.
List of Useful Links:
- AI Lab in Telegram @aiscrumbot – free consultation
- Johannes Kepler University Researchers Introduce GateLoop: Advancing Sequence Modeling with Linear Recurrence and Data-Controlled State Transitions
- MarkTechPost
- Twitter – @itinaicom